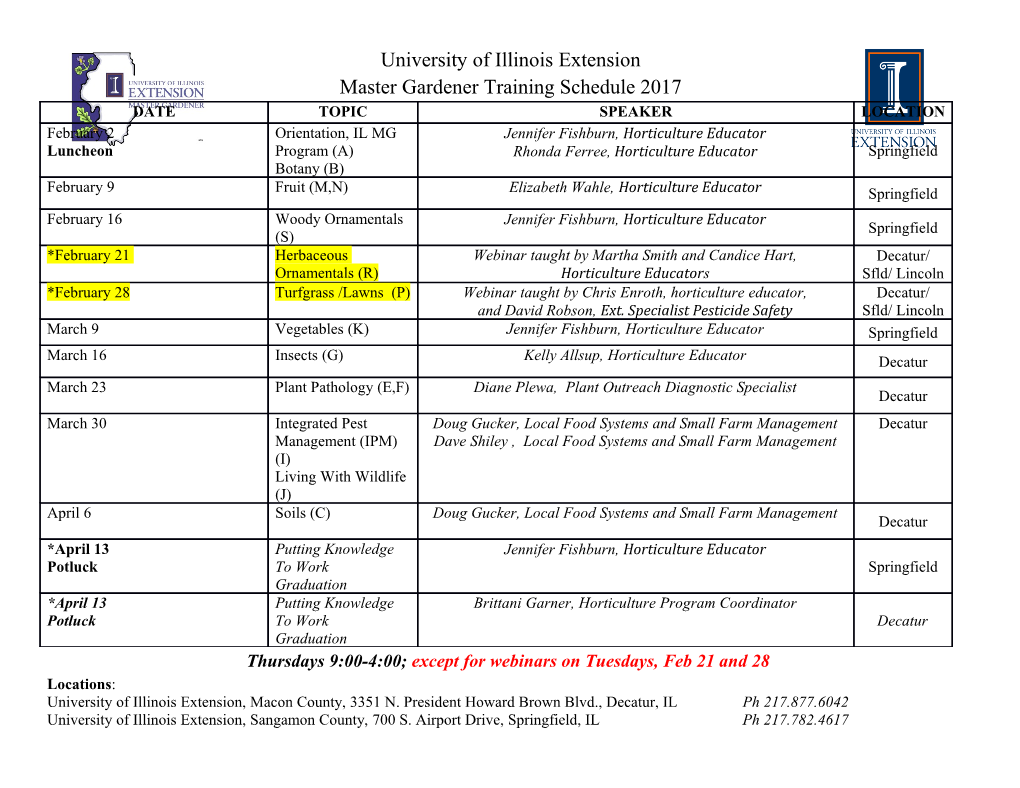
Monetization in Product and Display Advertising Marketplaces by Hana Choi Business Administration Duke University Date: Approved: Carl F. Mela, Supervisor Santiago R. Balseiro Bryan Bollinger Giuseppe Lopomo Hema Yoganarasimhan Dissertation submitted in partial fulfillment of the requirements for the degree of Doctor of Philosophy in the Department of Business Administration in the Graduate School of Duke University 2019 ABSTRACT Monetization in Product and Display Advertising Marketplaces by Hana Choi Business Administration Duke University Date: Approved: Carl F. Mela, Supervisor Santiago R. Balseiro Bryan Bollinger Giuseppe Lopomo Hema Yoganarasimhan An abstract of a dissertation submitted in partial fulfillment of the requirements for the degree of Doctor of Philosophy in the Department of Business Administration in the Graduate School of Duke University 2019 Copyright c 2019 by Hana Choi All rights reserved Abstract This dissertation considers monetization strategies in the context of online product and display ad marketplaces. The first chapter considers online marketplace platforms that trade-off their fees from advertising with commissions from product sales. While featuring advertised products can make search less efficient (lowering transaction commissions), it incen- tivizes sellers to compete for better placements via advertising (increasing advertising fees). We consider this trade-off by modeling both sides of the platform. On the de- mand side, we develop a joint model of browsing (impressions), clicking, and purchase. On the supply side, we consider sellers' valuation and advertising competition under various fee structures (CPM, CPC, CPA) and ranking algorithms. Using buyer, seller, and platform data from an online marketplace where advertis- ing dollars affect the order of seller items listed, we explore various product ranking and ad pricing mechanisms. We find that sorting items below the fifth position by expected sales revenue while conducting a CPC auction in the top 5 positions yields the greatest improvement in profits (181%) because this approach balances the high- est valuations from advertising in the top positions with the transaction revenues in the lower positions. The second chapter considers how a publisher should set reserve prices for real- time bidding (RTB) auctions when selling display advertising impressions through ad exchanges. Through a series of field experiments, we show that a reserve price set based on an imputed demand curve (in the absence of constraints) can increase publisher's revenues by 32%, thereby affirming the importance of reserve price in maximizing publisher's revenues. Further, we find that advertisers increase their bids in response to an experimental increase in reserve price and show this behavior iv is consistent with the use of a minimum impression constraint to ensure advertising reach. Based on this insight, we construct an advertiser bidding model and use it to infer the overall demand curve for advertising as a function of reserve prices. Using this constraint-based demand model, we solve the publisher pricing problem. Incor- porating the minimum impression constraint into the reserve price setting process yields a 50% increase over a solution that does not incorporate the constraint and an additional increase in profits of 9 percentage points. v Acknowledgements This dissertation would not be complete without acknowledging those who have sup- ported and encouraged me throughout my doctoral studies. First and foremost, I want to thank my advisor. My time at Duke has been invaluable because I have had the chance to learn from and work with Carl. His enthusiasm for studying interest- ing marketing topics and his perseverance for achieving rigorousness in research have shown me how to be a good researcher. His ability to think both theoretically and practically and his proficiency in delivering in-depth thoughts in a well-organized and approachable manner have taught me how to be a good teacher and presenter. His unflagging support, patience, and belief in my potential have not only sustained me through the process but also prepared me to be a generous mentor to others in the years to come. I am utmost grateful to be Carl's student. Second, I want to thank my committee members, Santiago Balseiro, Bryan Bollinger, Giuseppe Lopomo, and Hema Yoganarasimhan, who have provided thoughtful and constructive feedback during the dissertation process. Additionally, I want to thank Adam Leary who has given me the invaluable opportunity to closely study/experience display advertising markets, Peter Arcidiacono and Garrett Johnson who have served in my preliminary committee, and other Duke marketing faculty members. They all have generously provided helpful feedback on my research at many stages, and I have learnt much from talking with them, thinking about their comments, and collaborat- ing with them. Lastly, I also thank my student colleagues and friends in Duke and other market- ing programs. They have supported both professionally and personally by providing fresh perspectives on my projects and sharing the ups and downs of the journey we have gone through together. Finally, I thank my family, who have been most pa- vi tient and supportive while I slowly figured out my career path through a winding road. I especially thank my husband, Joon, for being emergency research assis- tant/programmer, attentive audience for my practice talks, and most importantly, for encouraging, supporting, and loving me every step of the way. vii Contents Abstract iv Acknowledgements vi List of Figures xii List of Tables xiii 1 Introduction 1 2 Monetizing Online Marketplaces 4 2.1 Introduction . .4 2.1.1 Overview . .4 2.1.2 Relevant Research . .6 2.1.3 Key Findings . .8 2.2 Data . .9 2.2.1 The Platform . 10 2.2.2 The Buyers . 14 2.2.3 The Advertisers . 19 2.3 Model . 22 2.3.1 The Consumer Model . 23 2.3.2 The Advertiser Model . 33 2.4 Estimation . 39 2.4.1 The Consumer Model . 39 2.4.2 The Advertiser Model . 44 viii 2.5 Results . 47 2.5.1 The Consumer Model . 47 2.5.2 The Advertiser Model . 48 2.6 Policy Simulation . 51 2.6.1 Simulation Procedures . 52 2.6.2 Consumer Model Simulations . 53 2.6.3 Advertiser Model Simulations . 56 3 Display Advertising Pricing in Exchange Markets 61 3.1 Introduction . 61 3.1.1 Overview . 61 3.1.2 Relevant Research . 64 3.1.3 Organization . 66 3.2 Data . 66 3.2.1 The Publisher and Data Source . 66 3.2.2 Summary Statistics . 67 3.2.3 Heterogeneity in Valuations . 69 3.2.4 Evidence for a Minimum Impression Constraint . 71 3.3 Model . 78 3.3.1 Model Overview . 79 3.3.2 Assumptions . 79 3.3.3 Advertiser Bidding Model . 82 3.3.4 Optimal Publisher Ad Auction Reserve Price . 86 3.4 Identification and Estimation . 89 3.4.1 Identification . 89 ix 3.4.2 Estimation . 91 3.4.3 Institutional Details . 93 3.5 Results . 96 3.5.1 The Valuation Distribution, FV ................. 97 3.5.2 The Impression Constraint, µ∗ .................. 98 3.6 Setting Reserve Prices . 99 3.6.1 Solving for the Optimal Reserve Price . 100 3.6.2 Optimal Reserves . 100 4 Conclusion 102 4.1 Monetizing Online Marketplaces . 102 4.2 Display Advertising Pricing in Exchange Markets . 105 4.3 Epilogue . 108 A Appendix: Monetizing Online Marketplaces 109 A.1 Data . 109 A.1.1 The Buyers: Data Sample . 109 A.1.2 The Advertisers . 109 A.2 Model . 113 A.2.1 State Transitions and Consumer Beliefs . 113 A.2.2 Additional Discussion on Sequential Search Process . 116 A.3 Estimation . 118 A.3.1 The Consumer Model . 118 A.3.2 The Advertiser Model . 125 A.4 Full Sample Results . 132 x A.4.1 The Consumer Model . 132 A.4.2 The Advertiser Model . 133 B Appendix: Display Advertising Pricing in Exchange Markets 135 B.1 Field Experiment . 135 B.1.1 Experiment Design . 135 B.1.2 Supplementary Field Experiment Analyses . 138 B.2 Model . 141 B.2.1 Proofs . 141 B.2.2 Theoretical Predictions . 144 B.3 Estimation and Policy Simulation . 148 B.3.1 Computational Steps in Estimation . 148 B.3.2 Computational Approach to Computing Optimal Reserves in the Policy Simulation . 149 B.4 Results . 151 B.4.1 The Valuation Distribution, FV ................. 151 Bibliography 151 xi List of Figures 2.1 Website Design . 11 2.2 Ranking Algorithm . 13 2.3 Browsing Length, Click, and Purchase by Position . 16 2.4 Position Effect on Click and Purchase . 18 2.5 Mean Organic Position and Advertising Percentage . 22 2.6 Model Overview . 23 2.7 Consumer Model . 24 2.8 Valuations from Consideration (Click) . 51 3.1 Advertiser Bid CPM: Heterogeneity . 69 3.2 Advertiser Bid CPM: Advertiser and Site Fixed Effects . 70 3.3 Treatment Effect on eCPM by Group . 75 3.4 Treatment Effect on Bid CPM Distribution . 76 3.5 Advertiser Valuations Distribution: Experiment 1 . 97 A.1 Product Listings . 110 A.2 Optimality of Search . 117 A.3 Beliefs on Product Placement . 128 B.1 Pre-Post Trends . 137 B.2 Optimal Bidding Strategy with a Maximum Budget Constraint . 147 B.3 Advertiser Valuation Distributions . 152 xii List of Tables 2.1 Summary Statistics of Consumers Behavior . 16 2.2 Deviations from Top-Down Search Process . 18 2.3 Product Attributes . 20 2.4 Advertising Strategies . 21 2.5 The Consumer Model Estimates . 49 2.6 The Advertiser Model Estimates . 50 2.7 Consumer Response . ..
Details
-
File Typepdf
-
Upload Time-
-
Content LanguagesEnglish
-
Upload UserAnonymous/Not logged-in
-
File Pages171 Page
-
File Size-