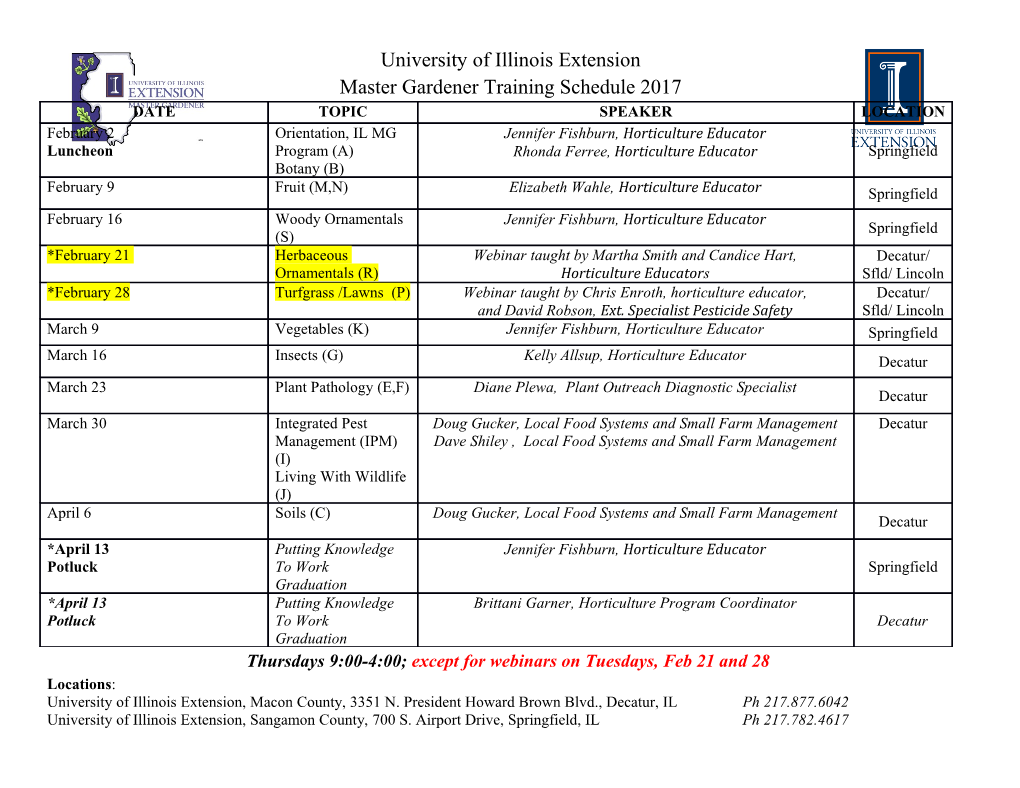
Front. Earth Sci. DOI 10.1007/s11707-015-0518-3 RESEARCH ARTICLE Mapping paddy rice distribution using multi-temporal Landsat imagery in the Sanjiang Plain, northeast China Cui JIN (✉)1, Xiangming XIAO1,2, Jinwei DONG1, Yuanwei QIN1, Zongming WANG3 1 Department of Microbiology and Plant Biology, Center for Spatial Analysis, University of Oklahoma, Norman, OK 73019, USA 2 Institute of Biodiversity Sciences, Fudan University, Shanghai 200433, China 3 Northeast Institute of Geography and Agricultural Ecology, Chinese Academy of Sciences, Changchun 130102, China © Higher Education Press and Springer-Verlag Berlin Heidelberg 2015 Abstract Information of paddy rice distribution is staple, feeding more than half of the world’s population essential for food production and methane emission (Van Nguyen and Ferrero, 2006). It plays an important role calculation. Phenology-based algorithms have been uti- in ensuring global food security. Global rice consumption lized in the mapping of paddy rice fields by identifying the has been predicted to exceed rice production (Kuenzer and unique flooding and seedling transplanting phases using Knauer, 2013). Approximately 95% of global rice is multi-temporal moderate resolution (500 m to 1 km) cultivated on flooded soil (Belder et al., 2004). Irrigation images. In this study, we developed simple algorithms to for rice cultivation requires large amounts of water and has identify paddy rice at a fine resolution at the regional scale an important impact on water quality. In addition, rice using multi-temporal Landsat imagery. Sixteen Landsat fields are one of the main sources of greenhouse gas images from 2010–2012 were used to generate the 30 m emissions (Li et al., 2004). Therefore, accurate high- paddy rice map in the Sanjiang Plain, northeast resolution maps of paddy rice distribution are critical for China—one of the major paddy rice cultivation regions in food production, water management, agriculture migra- China. Three vegetation indices, Normalized Difference tion, and agriculture adaption under global climate change Vegetation Index (NDVI), Enhanced Vegetation Index (Döll, 2002). (EVI), and Land Surface Water Index (LSWI), were used Remote sensing is an efficient tool for generating paddy to identify rice fields during the flooding/transplanting and rice maps. The potentials of fine-resolution satellite ripening phases. The user and producer accuracies of imagery, such as 20 m SPOT and 30/79 m Landsat, for paddy rice on the resultant Landsat-based paddy rice map classifying paddy rice fields have been explored (McCloy were 90% and 94%, respectively. The Landsat-based et al., 1987; Panigrahy and Parihar, 1992; Okamoto and paddy rice map was an improvement over the paddy rice Fukuhara, 1996; Laba et al., 1997; Okamoto et al., 1998; layer on the National Land Cover Dataset, which was Turner and Congalton, 1998). Single images were typically generated through visual interpretation and digitalization used in earlier studies due to the limited availability of on the fine-resolution images. The agricultural census data satellite imagery. Rice fields were visually interpreted from substantially underreported paddy rice area, raising serious color composite images, and their boundaries were then concern about its use for studies on food security. artificially digitalized onscreen (Rao and Rao, 1987; Qiu et al., 2003; Liu et al., 2005). Other studies used the Keywords phenology, flooding, transplanting, ripening, supervised or unsupervised classification algorithms to land use identify the spectral cluster of paddy rice (Panigrahy and Parihar, 1992; Laba et al., 1997; Okamoto et al., 1998; Turner and Congalton, 1998). However, the application of 1 Introduction these two approaches at regional or national scales is often labor-intensive and time-consuming. Changes in research Rice is the world’s second-largest crop and is a major food personnel and methods over time make it particularly difficult to obtain consistent classification results in the projects that analyze multiple-year images. Received October 12, 2014; accepted January 23, 2015 The Moderate Resolution Imaging Spectroradiometer E-mail: [email protected] (MODIS) provides global coverage of imagery every 1–2 2 Front. Earth Sci. days at 250 m, 500 m, and 1 km, and is free to the public. 2 Materials and methods The phenology-based algorithm developed on the multi- temporal MODIS data has shown a great potential for 2.1 Study area tracking the dynamics of the vegetation-to-water ratio during the rice growth, and can consistently map the The Sanjiang Plain is located in the northeast region of annual paddy rice distribution at regional scales (Xiao et Heilongjiang Province, China (129.19°E‒135.08°E, al., 2005; Sakamoto et al., 2006; Xiao et al., 2006; 43.83°N‒48.45°N). It covers 23 counties with a total Sakamoto et al., 2009a, b; Biradar and Xiao, 2011). area of 10.88 Â 104 km2. Approximately 80% of the However, the accuracy of the MODIS phenology-based Sanjiang Plain is relatively flat with an elevation < 200 m paddy rice maps was still questionable due to the mixed (Fig. 1(a)). The plain is characterized by a temperate and pixels caused by the coarse spatial resolution; a problem sub-humid continental monsoon climate, with a mean especially relevant in Asia, with over 200 million small- annual precipitation of 500–650 mm, the majority of which holding farms, typically under 1 hectare (Xiao et al., 2006; falls between July and September. The mean monthly Sun et al., 2009). One solution is the use of multi-temporal temperature varies from ‒18°C in January to 22 °C in July. high-resolution imagery (Xiao et al., 2006). The typical land cover types were cropland, woodland, and The United States Geological Survey (USGS) Earth natural wetland, accounting for 55.2%, 30.3%, and 7.4% Resources Observation and Science (EROS) Center has of the entire area, respectively (Huang et al., 2010b). offered free historical and new Landsat imagery to the The abundant water resources and fertile soils, along public since 2008. This provided a great opportunity for with the flat topography, make the Sanjiang Plain favorable regional-scale land cover classification. One significant for paddy rice cultivation. Rice cultivation is relatively accomplishment was the capability to track forest cover identical across the entire Sanjiang Plain (Zhang et al., dynamics by using multi-temporal Landsat imagery 2011). One rice crop per year is cultivated in this region (Hansen et al., 2013). Some studies tracked the continuous with a rice growth cycle duration of approximately 140– dynamics of spectral features derived from all available 150 days (Fig. 2(a)). From mid-April to early May, rice Landsat imagery across multi-years to identify forest and fields are prepared by plowing, overturning, flooding, and forest disturbance (Masek et al., 2008; Huang et al., 2010a; leveling. In mid- to late May, rice seedlings are Zhu et al., 2012). Several others extracted the annual transplanted to flooded fields. During these two phases, trajectory of image features from yearly Landsat imagery, rice fields are mostly dominated by water (Fig. 2(b)). Rice such as using one image within the peak of the annual canopy starts to rise rapidly during the vegetative growing growing season (Cohen et al., 2010; Kennedy et al., 2010). phase (tillering and stem elongation) from mid-June to Another study evaluated the spectral features of the forest early July (Fig. 2(c)), resulting in changes of the during different phenological phases, then screened the vegetation-to-water ratio. The reproductive phase starts specific phases when the forest showed spectral features in mid-July (panicle initiation, Fig. 2(d)), the vegetation- that were distinguishable from other land types (Dong to-water ratio reaches its maximum value in late July, and et al., 2013). then remains stable or slightly decreases during the The potential of multi-temporal Landsat imagery to ripening phase from late August to September (Fig. 2 monitor crops has been underestimated due to their (e)). Rice is harvested from late September to early spectral and phenological variability features (Zhong et October. al., 2014). Recent studies have highlighted the capability of intra-annual Landsat to identify corn and soybean 2.2 Landsat images and preprocessing (Zhong et al., 2014; Müller et al., 2015). Therefore, we hypothesize that paddy rice distribution mapping will The Sanjiang Plain is covered by 13 Landsat footprints benefit from the phenological features captured by multi- (Fig. 1(b)). 119 L1T Landsat images from 2010–2012 were temporal Landsat imagery. We examined if single dates of collected from http://landsat.usgs.gov/ and were used to phenological or spectral characteristics from the Landsat extract the multi-temporal curves of the Landsat vegetation imagery with low-observation frequency could be indices for typical land cover types. Sixteen images were extracted for paddy rice. For example, could multi- eventually used to generate the paddy rice map after temporal Landsat imagery track the dynamics of the examining three criteria (Table 1): 1) image acquisition vegetation-to-water ratio for rice fields similarly to the use date was during the peak of the transplanting/flooding and of MODIS data? ripening phases when paddy rice showed the distinguish- The objective of this study is to: (i) develop the Landsat ably phenological or spectral features from other land phenology-based scheme to identify paddy rice fields types; 2) cloud coverage was less than 5%; and 3) the gap- during two phenological (flooding/transplanting and filling strategy (see Section 2.5). ripening) phases at regional scales, and (ii) systematically All images were first processed for atmospheric evaluate the accuracy and uncertainties of the resultant correction and converted to surface reflectance using the Landsat-based paddy rice map. Landsat Ecosystem Disturbance Adaptive Processing Cui JIN et al. Mapping paddy rice distribution 3 Fig. 1 (a) Geographic location of the Sanjiang Plain, northeast China; (b) location of all Landsat footprints, high-resolution images available on Google Earth, and ground truth pointes collected in 2011.
Details
-
File Typepdf
-
Upload Time-
-
Content LanguagesEnglish
-
Upload UserAnonymous/Not logged-in
-
File Pages14 Page
-
File Size-