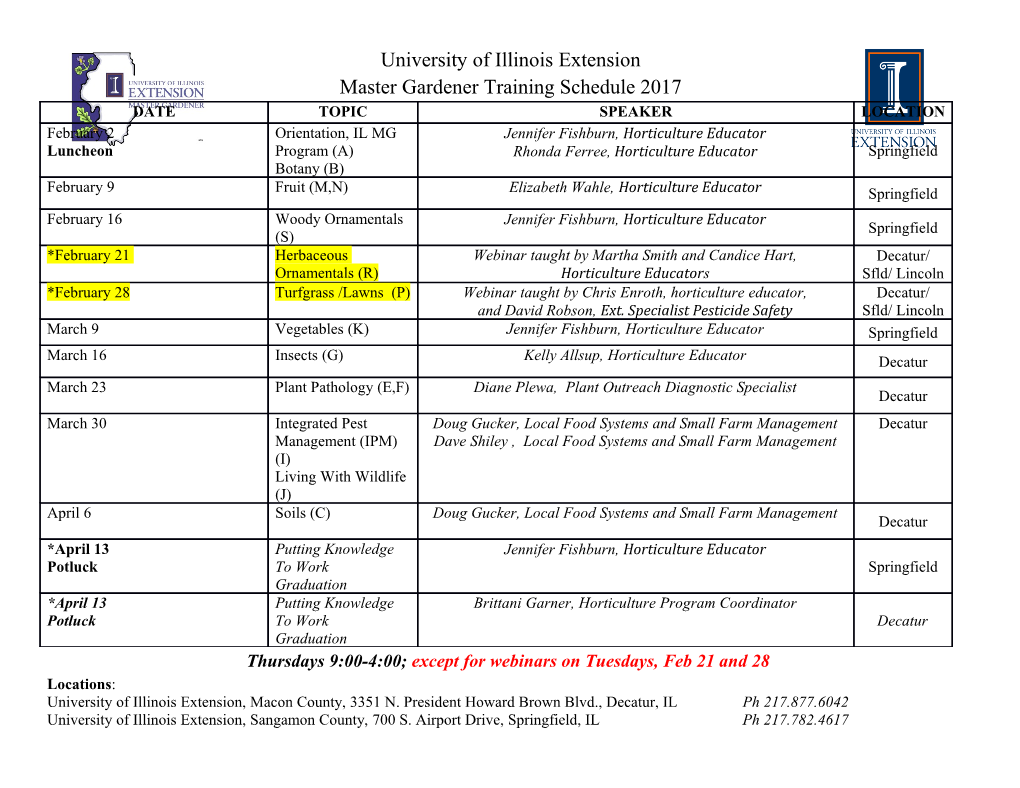
Color Image Processing MTAT.03.260 Pattern Recognition and Image Analysis (MTAT) Kristjan Krips Timo Petmanson University of Tartu March 18, 2011 Kristjan Krips, Timo Petmanson (University of Tartu)Color Image Processing March 18, 2011 1 / 39 Outline 1 Fundamentals 2 Color models 3 Pseudo-color processing 4 Full-color processing 5 Color transformations 6 Smoothing and sharpening 7 Color segmentation 8 Noise in color images Kristjan Krips, Timo Petmanson (University of Tartu)Color Image Processing March 18, 2011 2 / 39 Visible light Human eye is sensitive to electromagnetic radiation between wavelengths of approximately from 390 nm to 750 nm. Kristjan Krips, Timo Petmanson (University of Tartu)Color Image Processing March 18, 2011 3 / 39 Human eye reception / primary colors 65% cones are sensitive to red light 33% of cones are sensitive to green light 2% of cones are sensitive to blue light Kristjan Krips, Timo Petmanson (University of Tartu)Color Image Processing March 18, 2011 4 / 39 Primary / secondary colors red + blue = magneta red + green = yellow blue + green = cyan Kristjan Krips, Timo Petmanson (University of Tartu)Color Image Processing March 18, 2011 5 / 39 Color characteristics brightness - a subjective measure of chromatic intensity of color hue - represents the dominant color (dominant wavelenght), e.g. red, blue saturation - the relative purity of the color, the amount of white light mixed with the hue. chromaticity = hue + saturation Kristjan Krips, Timo Petmanson (University of Tartu)Color Image Processing March 18, 2011 6 / 39 Trichromatic coecients A color can be specied by its trichromatic coecients: x X , y Y , z Z , where x y z 1 I = X +Y +Z = X +Y +Z = X +Y +Z + + = R ¥ R ¥ R ¥ X = 0 I (l)x(l)dl, Y = 0 I (l)y(l)dl, Z = 0 I (l)z(l)dl I (l) is the spectral power distribution of a given color, x(l);y(l);z(l) are color matching functions of standard observer eg. combination of three linear light detectors. Kristjan Krips, Timo Petmanson (University of Tartu)Color Image Processing March 18, 2011 7 / 39 CIE chromaticity diagram The diagram is the function of red and green. The blue is obtained from equation z = 1 − (x + y). The colors on the boundary are fully saturated, where the ticks denote the wavelength in nanometers. Kristjan Krips, Timo Petmanson (University of Tartu)Color Image Processing March 18, 2011 8 / 39 CIE chromaticity diagram By connecting any two points with a line in the plot, we get the all possible combinations of colors that can obtained by mixing the endpoint colors. Grassman's Law: Any colour can be matched by a linear combination of three other colours, provided that none of those three can be matched by a combination of the other two Kristjan Krips, Timo Petmanson (University of Tartu)Color Image Processing March 18, 2011 9 / 39 sRGB color space and the gamut Gamut represents the colors producable of a typical color monitor. In experiments carried out by W. David Wright and John Guild, the standard observer used to set up the sRGB diagram was human. Color matching functions x(l); y(l); z(l) were summarized based on the experiments The vertices of the triangle represent 3 primary colors easily producable by monochromatic lines of a mercury vapor discharge. Kristjan Krips, Timo Petmanson (University of Tartu)Color Image Processing March 18, 2011 10 / 39 RGB model Derived from primary color components red, green and blue. Normalized into range [0,1] (in computers typically 0..255]) Color cube: I black is (0,0,0) I white is (1,1,1) I red is (1,0,0) I green (0,1,0) I blue is (0,0,1) Kristjan Krips, Timo Petmanson (University of Tartu)Color Image Processing March 18, 2011 11 / 39 RGB model http://www.mathworks.com/help/toolbox/images/f8-15484.html Kristjan Krips, Timo Petmanson (University of Tartu)Color Image Processing March 18, 2011 12 / 39 RGB <=> CIE color space 1 We need a transfer functions fr ; fg ; fb for each color channel of the monitor device that dene the relationship between input pixel values and displayed intensity. 2 The relationship between CIE trimulus values X,Y,Z and displayed image pixel values R, G, B is given below. 3 The 3x3 matrix can be calculated by deom chromaticity values for three channels and the white point usually published by the manufacturer. 2 X 3 2 Xr Xg Xb 3 2 fr (R) 3 4 Y 5 = 4 Yr Yg Yb 5 ∗ 4 fg (G) 5 Z Zr Zg Zb fb(B) (−1) 2 fr (R) 3 2 Xr Xg Xb 3 2 X 3 4 fg (G) 5 = 4 Yr Yg Yb 5 ∗ 4 Y 5 fb(B) Zr Zg Zb Z Kristjan Krips, Timo Petmanson (University of Tartu)Color Image Processing March 18, 2011 13 / 39 Calculating the 3x3 matrix Manufacturer usually publishes chromaticity coordinates for the primaries and the white point. Colour Chromaticity coordinates red (xr ;yr ;zr ) green (xg ;yg ;zg ) blue (xb;yb;zb) white (xw ;yw ;zw ) Assuming that relative luminance equals to 1, we can nd constants ar ;ag ;ab from the simulanteus equations xn ar xr ag xg abxb + + = yn ar yr + ag yg + abyb = 1 => zn ar zr ag zg abzb + + = yn 2 X 3 2 ar xr ag xg abxb 3 2 fr (R) 3 4 Y 5 = 4 ar yr ag yg abyb 5 ∗ 4 fg (G) 5 Z ar zr ag zg abzb fb(B) Kristjan Krips, Timo Petmanson (University of Tartu)Color Image Processing March 18, 2011 14 / 39 CMYK model Derived from secondary colors. Used as a main model in printing devices where blending of color pigements are used to achieve colors. Equal amounts of cyan, magneta and yellow does not give true black, thus black pigment is additionally used in blending process. However, this simple transformation is good enough for simper graphics, but it will produce poor results for applications with higher requirements such as an colour printer. 2 C 3 2 1 3 2 R 3 CMY <=> RGB4 M 5 = 4 1 5 − 4 G 5 Y 1 B Kristjan Krips, Timo Petmanson (University of Tartu)Color Image Processing March 18, 2011 15 / 39 CMY => CMYK Black = min(Cyan, Magneta, Yellow) Cyan = (Cyan - Black) / (1 - Black) Magneta = (Magneta - Black) / (1 - Black) Yellow = (Yellow - Black) / (1 - Black) Kristjan Krips, Timo Petmanson (University of Tartu)Color Image Processing March 18, 2011 16 / 39 HSL, HSV, (HSI) model HSL (hue, saturation, lightness) and HSV (hue, saturation, value) model is easily interpretable by humans. They are also two most common cylindrical-coordinate representations of points in an RGB color model. Kristjan Krips, Timo Petmanson (University of Tartu)Color Image Processing March 18, 2011 17 / 39 Basic idea Starting at the red primary at 0°, passing through the green primary at 120° and the blue primary at 240°, and then wrapping back to red at 360°. In each geometry, the central vertical axis comprises the neutral, achromatic, or gray colors, ranging from black at lightness 0 or value 0, the bottom, to white at lightness 1 or value 1, the top. Kristjan Krips, Timo Petmanson (University of Tartu)Color Image Processing March 18, 2011 18 / 39 Hue and chroma Hue is roughly the angle of a vector representing the color, where chroma is the length. Kristjan Krips, Timo Petmanson (University of Tartu)Color Image Processing March 18, 2011 19 / 39 Hue and chroma Kristjan Krips, Timo Petmanson (University of Tartu)Color Image Processing March 18, 2011 20 / 39 Lightness The simplest denition is just the average of the three components, in the HSI model called intensity. 1 I I = 3 (R + G + B) In the HSV "hexcone" model, value is dened as the largest component of a color, our M above. This places all three primaries, and also all of the "secondary colors" cyan, yellow, and magenta into a plane with white, forming a hexagonal pyramid out of the RGB cube. I V = M In the HSL "bi-hexcone" model, lightness is dened as the average of the largest and smallest color components (g. 11c). This denition also puts the primary and secondary colors into a plane, but a plane passing halfway between white and black. 1 I L = 2 (M + m) Kristjan Krips, Timo Petmanson (University of Tartu)Color Image Processing March 18, 2011 21 / 39 Lightness Kristjan Krips, Timo Petmanson (University of Tartu)Color Image Processing March 18, 2011 22 / 39 Saturation Kristjan Krips, Timo Petmanson (University of Tartu)Color Image Processing March 18, 2011 23 / 39 HSV => RGB Kristjan Krips, Timo Petmanson (University of Tartu)Color Image Processing March 18, 2011 24 / 39 HSL => RGB Kristjan Krips, Timo Petmanson (University of Tartu)Color Image Processing March 18, 2011 25 / 39 Pseudo-color (false color) processing / Basic idea Pseudo-color (false color) processing takes a monochrome image and applies colors to it. The idea is to make grayscale images more easily readable and interpretable to humans. I mapping intensity ranges to color. I for example we can divide the intensity range [0..1] into subregions and map a color to each range Kristjan Krips, Timo Petmanson (University of Tartu)Color Image Processing March 18, 2011 26 / 39 Gray level to color transformations Wider range of pseudocolor results, nonlinear Independent transformation for each primary color Results are mixed together into a composite image Kristjan Krips, Timo Petmanson (University of Tartu)Color Image Processing March 18, 2011 27 / 39 Full-color image processing Process each component image individually and form a composite image I standard gray-scale image processing methods Work directly with color pixels I pixels are vectors I averaging per-color-component Kristjan Krips, Timo Petmanson (University of Tartu)Color Image Processing March 18, 2011 28 / 39 Basic color transformations Processing the components of an image in a single model I g (x;y) = T [f (x;y)], where f(x,y) is the input image and g(x,y) is the processed image I si = Ti (r1;r2;:::;rn), where i = 1,2,...,n and si , ri denote the color components of f(x,y), g(x,y) at any point (x,y); n is the number of components; fT1;T2;:::;Tng is a set of color transformation functions.
Details
-
File Typepdf
-
Upload Time-
-
Content LanguagesEnglish
-
Upload UserAnonymous/Not logged-in
-
File Pages39 Page
-
File Size-