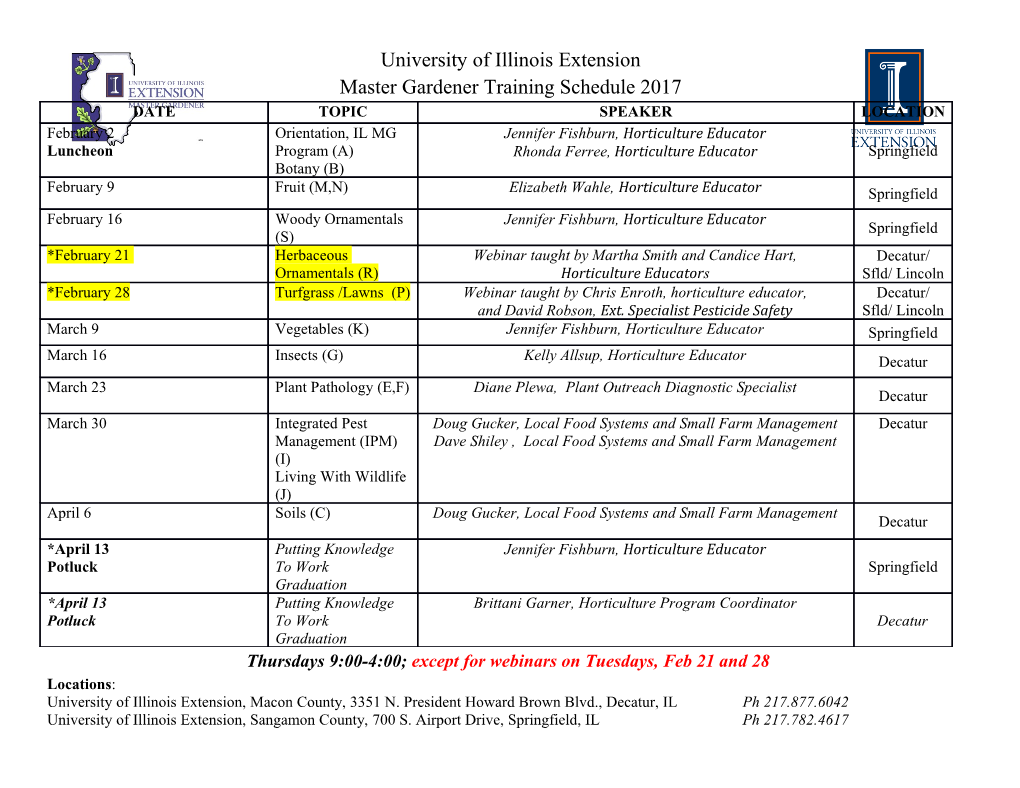
INTEGRATING PROBABILISTIC REASONING WITH CONSTRAINT SATISFACTION by Eric I-Hung Hsu A thesis submitted in conformity with the requirements for the degree of Doctor of Philosophy Graduate Department of Computer Science University of Toronto Copyright c 2011 by Eric I-Hung Hsu Abstract Integrating Probabilistic Reasoning with Constraint Satisfaction Eric I-Hung Hsu Doctor of Philosophy Graduate Department of Computer Science University of Toronto 2011 We hypothesize and confirm that probabilistic reasoning is closely related to constraint sat- isfaction at a formal level, and that this relationship yields effective algorithms for guiding constraint satisfaction and constraint optimization solvers. By taking a unified view of probabilistic inference and constraint reasoning in terms of graphical models, we first associate a number of formalisms and techniques between the two areas. For instance, we characterize search and inference in constraint reasoning as summation and multiplication (or disjunction and conjunction) in the probabilistic space; necessary but insufficient consistency conditions for solutions to constraint problems (like arc-consistency) mirror approximate objective functions over probability distributions (like the Bethe free en- ergy); and the polytope of feasible points for marginal probabilities represents the linear relax- ation of a particular constraint satisfaction problem. While such insights synthesize an assortment of existing formalisms from varied research communities, they also yield an entirely novel set of “bias estimation” techniques that con- tribute to a growing body of research on applying probabilistic methods to constraint prob- lems. In practical terms, these techniques estimate the percentage of solutions to a constraint satisfaction or optimization problem wherein a given variable is assigned a given value. By de- vising search methods that incorporate such information as heuristic guidance for variable and value ordering, we are able to outperform existing solvers on problems of interest from con- straint satisfaction and constraint optimization–as represented here by the SAT and MaxSAT ii problems. Further, for MaxSAT we present an “equivalent transformation” process that normalizes the weights in constraint optimization problems, in order to encourage prunings of the search tree during branch-and-bound search. To control such computationally expensive processes, we determine promising situations for using them throughout the course of an individual search process. We accomplish this using a reinforcement learning-based control module that seeks a principled balance between the exploration of new strategies and the exploitation of existing experiences. iii Acknowledgements I would like to recognize the support and friendship of Sheila McIlraith, my academic advisor, throughout the preparation of this dissertation, the conduct of the research that it represents, and the overall process of completing the doctoral program. Thanks also to my committee members, Fahiem Bacchus and Chris Beck, as well as the external examiner, Gilles Pesant, for their many valuable corrections, criticisms, questions, and suggestions, as well as their constant advocacy and flexibility. For their guidance and inspiration earlier in my career, I would also like to acknowledge Barbara Grosz, Karen Myers, Charlie Ortiz, and Wheeler Ruml. Many other researchers have made direct contributions, small or large, to the specific project described in this dissertation. A partial listing would include: Dimitris Achlioptas, Ronan Le Bras, Jessica Davies, Rina Dechter, Niklas Een,´ John Franco, Brendan Frey, Inmar Givoni, Vibhav Gogate, Carla Gomes, Geoff Hinton, Holger Hoos, Frank Hutter, Matthew Kitching, Lukas Kroc, Chu Min Li, Victor Marek, Mike Molloy, Christian Muise, Pandu Nayak, Andrew Ng, Ashish Sabharwal, Horst Samulowitz, Sean Weaver, Toma´sˇ Werner, Lin Xu, Alessandro Zanarini, and many anonymous reviewers who have applied their attention and intellect to previous publications. I am glad to be in the professional and/or personal company of such researchers, as well as the many more who did not have a direct role in this specific project but have enriched my experience to this point. Finally, the dissertation is dedicated to my mate, Sara Mostafavi. iv Contents I Foundations 13 1 Computing Marginal Probabilities over Graphical Models 14 1.1 Graphical Models and Marginal Probabilities . 15 1.1.1 Basic Factor Graphs: Definitions, Notation, and Terminology . 16 1.1.2 Transforming a Factor Graph: the Dual Graph and Redundant Graph . 19 1.1.3 The Marginal Computation Problem . 23 1.2 Exact Methods for Computing Marginals . 27 1.2.1 Message Passing for Trees . 27 1.2.2 Transforming Arbitrary Graphs into Trees . 31 1.2.3 Other Methods: Cycle-Cutset and Recursive Conditioning . 38 1.3 Inexact Methods for Computing Marginals . 39 1.3.1 Message-Passing Algorithms . 39 1.3.2 Gibbs Sampling and MCMC . 41 1.4 The Most Probable Explanation (MPE) Problem . 42 2 Message-Passing Techniques for Computing Marginals 50 2.1 Belief Propagation as Optimization . 50 2.1.1 Gibbs Free Energy . 51 2.1.2 Mean Field Free Energy Approximation . 53 2.1.3 Bethe Free Energy Approximation . 55 v 2.1.4 Kikuchi Free Energy Approximation . 57 2.2 Other Methods as Optimization: Approximating the Marginal Polytope . 58 3 Solving Constraint Satisfaction Problems 62 3.1 Factor Graph Representation of Constraint Satisfaction . 63 3.2 Complete Solution Principles for Constraint Satisfaction . 70 3.2.1 Search . 71 3.2.2 Inference . 77 3.3 Incomplete Solution Principles for Constraint Satisfaction . 84 3.3.1 Decimation . 85 3.3.2 Local Search . 86 3.4 The Optimization, Counting, and Unsatisfiability Problems . 89 4 Theoretical Models for Analyzing Constraint Problems 91 4.1 The Phase Transition for Random Constraint Satisfaction Problems . 92 4.1.1 Defining Random Problems and the Phase Transition . 92 4.1.2 Geometry of the Solution Space . 94 4.2 The Survey Propagation Model of Boolean Satisfiability . 97 4.3 Backbone Variables and Backdoor Sets . 98 4.3.1 Definitions and Related Work . 98 4.3.2 Relevance to the Research Goals . 100 5 Integrated View of Probabilistic and Constraint Reasoning 102 5.1 Relationship between Probabilistic Inference and Constraint Satisfaction . 103 5.1.1 Representing Constraint/Probabilistic Reasoning as Discrete/Continuous Optimization . 105 5.1.2 Duality in Probabilistic Reasoning, Constraint Satisfaction, and Nu- merical/Combinatorial Optimization . 113 vi 5.1.3 Node-Merging . 116 5.1.4 Adding Constraints During the Solving Process . 117 5.1.5 Correspondences between Alternative Approaches . 118 5.2 EMBP: Expectation Maximization Belief Propagation . 119 5.2.1 Deriving the EMBP Update Rule within the EM Framework. 120 5.2.2 M-Step . 122 5.2.3 E-Step . 125 5.2.4 The EMBP Update Rule . 127 5.2.5 Relation to (Loopy) BP and Other Methods . 129 5.2.6 Practical Yield of EMBP . 130 II Solving Constraint Satisfaction Problems 132 6 A Family of Bias Estimators for Constraint Satisfaction 133 6.1 Existing Methods and Design Criteria . 134 6.2 Deriving New Bias Estimators for SAT . 139 6.2.1 The General EMBP Framework for SAT . 140 6.2.2 Representing the Q-Distribution in Closed Form . 143 6.2.3 Deriving EMBP-L . 144 6.2.4 Deriving EMBP-G . 146 6.2.5 Deriving EMSP-L . 148 6.2.6 Deriving EMSP-G . 149 6.2.7 Other Bias Estimators . 151 6.3 Interpreting the Bias Estimators . 153 7 Using Bias Estimators in Backtracking Search 158 7.1 Architecture and Design Decisions for the VARSAT Integrated Solver . 160 7.1.1 The General Design of VARSAT . 160 vii 7.1.2 Specific Design Decisions within VARSAT . 161 7.2 Standalone Performance of SAT Bias Estimators . 165 7.2.1 Experimental Setup . 166 7.2.2 Findings . 167 7.2.3 Other Experiments . 171 7.3 Performance of Bias Estimation in Search . 173 7.3.1 Limitations and Conclusions . 177 III Solving Constraint Optimization Problems 180 8 A Family of Bias Estimators for Constraint Optimization 181 8.1 Existing Methods and Design Criteria . 182 8.1.1 Branch-and-Bound Search . 183 8.1.2 Other Probabilistic Approaches . 185 8.2 Creating an Approximate Distribution over MaxSAT Solutions . 186 8.3 Deriving New Bias Estimators for MaxSAT . 188 9 Using Bias Estimators in Branch-and-Bound Search 191 9.1 Architecture and Design Decisions for the MAXVARSAT Integrated Solver . 191 9.2 Performance of Bias Estimation in Search . 195 9.2.1 Random Problems . 195 9.2.2 General Problems . 196 9.2.3 Limitations and Conclusions . 198 10 Computing MHET for Constraint Optimization 201 10.1 Motivation and Definitions . 202 10.2 Adopting the Max-Sum Diffusion Algorithm to Perform MHET on MaxSAT . 209 10.3 Implementation and Results . 217 viii 10.3.1 Problems for which MHET is Beneficial, Neutral, or Harmful Overall . 217 10.3.2 Direct Measurement of Pruning Capability, and Proportion of Runtime 219 10.3.3 Practical Considerations: Blending the Two Pruning Methods . 220 10.4 Related Work and Conclusions . 222 10.4.1 Comparison with the Virtual Arc-Consistency Algorithm . 223 10.4.2 Conclusion . 224 IV Pragmatics 227 11 Online Control of Constraint Solvers 228 11.1 Existing Automatic Control Methods . 229 11.2 Reinforcement Learning Representation of Branch-and-Bound Search . 233 11.2.1 Markov Decision Processes and Q-Learning . 234 11.2.2 Theoretical Features of Q-Learning. 237 11.3 Application to MaxSAT . 237 11.4 Experimental Design and Results . 240 11.4.1 Example Policy Generated Dynamically by Q-Learning . 244 11.4.2 Concluding Remarks . 246 12 Future Work 249 12.1 General Program for Future Research . 249 12.2 Specific Research Opportunities . 251 12.2.1 Conceptual Research Directions . 251 12.2.2 Algorithmic Research Directions . 253 12.2.3 Implementational Research Directions . 255 13 Conclusion 256 Bibliography 259 ix List of Tables 5.1 Primal/Dual concepts from constraint satisfaction and probabilistic reasoning. 115 5.2 EM formulation for estimating marginals using the redundant factor graph transformation.
Details
-
File Typepdf
-
Upload Time-
-
Content LanguagesEnglish
-
Upload UserAnonymous/Not logged-in
-
File Pages288 Page
-
File Size-