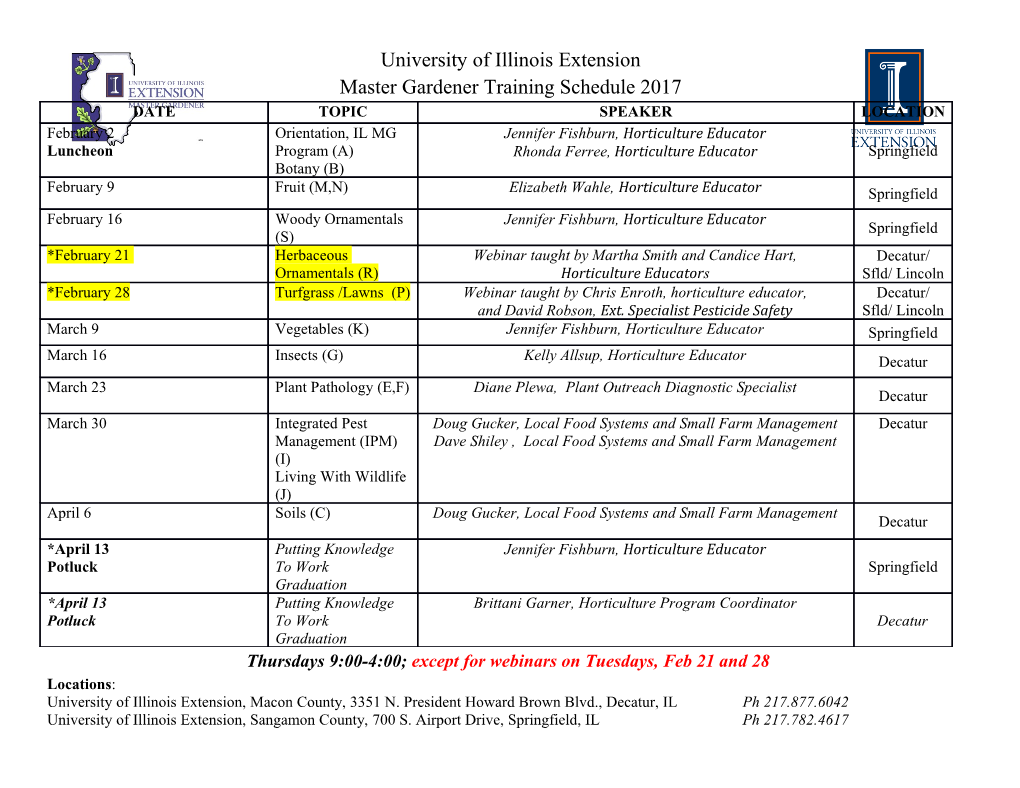
Bioinformatics 1 -- Lecture 22 IMMUNOINFORMATICS: Bioinformatics Challenges in Immunology Most slides courtesy of Julia Ponomarenko, San Diego Supercomputer Center or Oliver Kohlbacher, WSI/ZBIT, Eberhard-Karls- Universität Tübingen The Immune Reaction 2 Vaccines have been made for 36 of >400 human pathogens Immunological Bioinformatics, 2005 The 4 MIT press. +HPV & Rotavirus Epitope Quantum unit of immunity Surface on which an antibody binds Comprises antigenic matter Linear epitope consists of a short AA sequence Conformational epitope depends on tertiary structure. 4 Two branches of immunity Innate Adaptive immunity immunity Recognizes molecules, called pathogen- Recognizes epitopes; that are specific associated molecular patterns (~103), or B- and T-cell recognition sites on PAMPs shared by groups of related antigens. microbes; e.g. LPS from the gram-negative cell wall, RNA from viruses, flagellin, and glucans from Is antigen-specific. fungal cell walls. 3 to 10 days response. Is antigen-nonspecific. Involves the following: Immediate or within several hours response. antigen-presenting cells (APCs) such as macrophages and dendritic cells; Involves body defense cells that have pattern-recognition receptors: . antigen-specific B-lymphocytes (~109); . Leukocytes: neutrophils, eosinophils, basophils . antigen-specific T-lymphocytes (~ and monocytes; 1012); and . cells that release inflammatory mediators: . cytokines. macrophages and mast cells; . natural killer cells (NK cells); and Improves with repeated exposure and . complement proteins and cytokines. becomes protective. Does not improve with repeated exposure to 3 a given infection. Components of the immune system 6 5 B and T cells recognize diferent epitopes of the same protein T-cell epitope B-cell epitope Denatured antigen Native or denatured (rare) antigen Linear (often) peptide 8-37 aa Sequential (continuous) or Internal (often) conformational (discontinuous) Accessible, hydrophilic, mobile, usually on the surface or could be Binding to T cell receptor: exposed as a result of -5 -7 physicochemical change Kd 10 – 10 M (low afnity) Binding to antibody: Slow on-rate, slow of-rate -7 -11 (once bound, peptide may stay Kd 10 – 10 M (high afnity) associated for hours to many days) Rapid on-rate, variable of-rate 11 B cell (magenta, orange) and T cell epitopes (blue, green, red) of hen egg-white lysozyme 10 PDB: 1dpx MHC class I pathway Intracellular pathogen (virus, mycobacteria) Cytosolic protein Proteasome Peptides CD8 TAP epitope ER ER MHC I TCR CD8 CTL (TCD8+) Any cell 13 Xenoreactive Complex AHIII 12.2 TCR bound to P1049 (ALWGFFPVLS) /HLA- MHC class I pathway A2.1 Intracellular pathogen T-Cell (virus, mycobacteria) Receptor Cytosolic protein V β Proteasome Vα Peptides CD8 TAP epitop ER ER e MHC I TCR MHC class I CD8 CTL (TCD8+) β-2- Any cell 14 Microglobulin 1lp9 MHC class I pathway Intracellular pathogen (virus, mycobacteria) Bioinformatics approaches at epitope prediction: Cytosolic protein (1) Prediction of proteosomal cleavage Proteasome sites (several methods exist based on small amount of in vitro data). Prediction of peptide-TAP binding Peptides (2) (ibid.). CD8 TAP epitop (3) Prediction of peptide-MHC ER ER e binding. MHC I TCR (4) Prediction of pMHC-TCR binding. CD8 CTL (TCD8+) Any cell 15 MHC class I epitope prediction: Challenges High rate of pathogen mutations. Pathogens evolve to escape: . Proteosomal cleavage (HIV); . TAP binding (HIV, HSV type I); . MHC binding. MHC genes are highly polymorphic (2,292 human alleles/1,670 - 2 yeas ago). MHC polymorphism is essential to protect the population from invasion by pathogens. But it generates problem for epitope-based vaccine design: a vaccine needs to contain a unique epitope binding to each MHC allele. Every normal (heterozygous) human expresses six diferent MHC class I molecules on every cell, containing α-chains derived from the two alleles of HLA-A, HLA-B, HLA-C genes that inherited from the parents. Every human has ~1012 lymphoid cells with a T-cell receptors repertoire of ~107 , depending on her immunological status (vaccinations, disease history, environment, etc.). 16 Prediction of MHC class I binding peptide – potential epitopes ALAKAAAAM ALAKAAAAN MHC allele or allele supertype (similar in sequences ALAKAAAAV alleles bind similar peptides) specific. ALAKAAAAT Peptide length (8-, 9-, 10-, 11-mers) specific. GMNERPILT Sequence-based approaches: GILGFVFTM . Gibbs sampling (when the training peptides are TLNAWVKVV of diferent lengths) KLNEPVLLL . Hidden Markov Models (ibid.) AVVPFIVSV . Sequence motifs, position weight matrices Peptides . Artificial Neural Networks (require a large known to number of training examples) bind to the HLA-A*0201 . SVM* molecule. 17 Performance measures for prediction methods Predicted score (binding afnity ROC curve 1.0 value) TP 0.8 FP 0.5 Score AUC FN threshold 0.3 or TN AROC True positive rate, TP / (TP + FN) / (TP positive rate, TP True 0 0 0.2 0.4 0.6 0.8 1 based on TP+FN – actual binders ( False positive rate, FP / (FP + TN) a defined threshold on binding afnity values) TN+ FP – actual non-binders (ibid.) Sensitivity = TP / (TP + FN) = 6/7= 0.86 Specificity = TN / (TN + FP) = 6/8 = 0.75 18 Performance measures for prediction methods (cont) Predicted score pi (binding afnity Pearson’s correlation value) coefcient TP FP Score FN threshold TN TP+FN – actual binders (based on a defined threshold on actual binding afnity values ai ) TN+ FP – actual non-binders (ibid.) Sensitivity = TP / (TP + FN) = 6/7= 0.86 19 Specificity = TN / (TN + FP) = 6/8 = 0.75 MHC class I pathway MHC class II pathway Intracellular pathogen (virus, mycobacteria) Extracellular protein Cytosolic protein Endosome Proteasome ? Peptides MHC II CD8 TAP epitope CD4 epitope ER ER MHC I TCR TCR CD4 CD8 CTL TCD4+ (TCD8+) Any cell B-cell, macrophage, or dendritic cell29 Complex Of A Human TCR, Influenza HA Antigen Peptide (PKYVKQNTLKLAT) and MHC Class II MHC class I pathway MHC class II pathway T-Cell Intracellular pathogen (virus,Receptor mycobacteria) Extracellular protein Cytosolic protein Endosome Proteasome Vα V β ? Peptides MHC II TAP CD4 MHC class II ER ER epitop α e MHC I TCR MHCT classCD8+ II CD4 TCD4+ β Any cell B-cell, macrophage, or dendritic cell30 Complex Of A Human TCR, Influenza HA Xenoreactive Complex AHIII 12.2 TCR Antigen Peptide (PKYVKQNTLKLAT) and MHC bound to P1049 (ALWGFFPVLS) /HLA- Class II A2.1 Epitope, or antigenic determinant, is T-Celldefined as the site of an antigen recognized by immune response T-Cell Receptormolecules (antibodies, MHC, TCR) Receptor T cell epitope – a short linear peptide or other chemical entity (native or denatured antigen) that binds MHC V β (class I binds 8-10 ac peptides; classV αII Vα bindsV β 11-25 ac peptides) and may be recognized by T-cell receptor (TCR). TMHC cell recognition of antigen involves tertiaryclass IIcomplex α “antigen-TCR-MHC”. MHC class I MHC class II β β-2- 31 1fyt Microglobulin 1lp9 MHC class II epitope prediction: Challenges Complex Of A Human TCR, Influenza HA MHC class II genes are as highly Antigen Peptide (PKYVKQNTLKLAT) and polymorphic as MHC class I (1,012 MHCExtracellular Class II protein human alleles for today). T-Cell The repertoire of T-cell receptors ReceptorEndosome is ~107 and depends on an individual’s immunological status (vaccinations, disease history, environment, etc.). ? MHC II Vα The epitope length 9-37 aa. V The peptide may have non-linear β CD4 conformation. epitope MHC The MHC binding groove is open class II from both sides and it is known α that residues outside the groove efect peptide binding. TMHCCD4+ class II B-cell, macrophage, or dendriticβ cell32 MHC class II epitope prediction: Challenges Extracellular protein Endosome The processing of MHC class II epitopes is still a mystery and likely depends on the antigen structure, the ? cell type and other factors. MHC II CD4 epitope TCR CD4 TCD4+ B-cell, macrophage, or dendritic cell33 22 Prediction of MHC class II binding peptide – potential epitopes MHC allele or allele supertype (similar in sequences alleles bind similar peptides) specific. Predictions for peptides of length 9 aa (the peptide-MHC binding core) Sequence-based approaches: . Gibbs sampling . Sequence motifs, position weight matrices . Machine learning: SVM, HMM, evolutionary algorithms 35 Nielsen et al., Bioinformatics 2004 Benchmarking predictions of peptide binding to MHC II (Wang et al. PLoS Comput Biol. 2007) Data: pairs {peptide – afnity value in terms of IC50 nM} for a given MHC allele 16 diferent mouse and human MHC class II alleles. 10,017 data points. 9 diferent methods were evaluated: 6 matrix-based, 2 SVM, 1 QSAR-based. AUC values varied from 0.5 (random prediction) to 0.83, depending on the allele. Comparison with 29 X-ray structures of peptide-MHC II complexes (14 diferent alleles): . The success level of the binding core recognition was 21%-62%, . with exception of TEPETOPE method (100%) that is based on structural information and measured afnity values for mutant variants of MHC class II and peptides (Sturniolo et al., Nature Biotechnology, 1999). => Structural information together with peptide-MHC binding data should improve the prediction. 36 38 26 ] 27 28 29 30 31 32 33 34 35 36 37 38 39 40 41 42 43 44 45 46 47 48 49 50 51 52 53 54 55 56 57 58 59 60 61 62 Outstanding problems in TAP/ Proteosome/MHC peptide prediction High throughput data generation . Currently few verified peptide sequences. Overfitting likely Something better than the usual machine learning approach? . Structure-based motifs, length preferences? . Combine multiple motifs, reflecting multiple enzymes? Vaccine design to incorporate cleavage motifs . Optimize recombinant vaccine for cleavage? 63 Cellular immunity 64 65 Epitope-based vaccines A major reason for analyzing and predicting epitopes is because they may lead to the development of peptide-based synthetic vaccines. Thousands of peptides have been pre-clinically examined; over 100 of them have progressed to phase I clinical trials and about 30 to phase II, including vaccines for foot-and-mouth virus infection, influenza, HIV.
Details
-
File Typepdf
-
Upload Time-
-
Content LanguagesEnglish
-
Upload UserAnonymous/Not logged-in
-
File Pages67 Page
-
File Size-