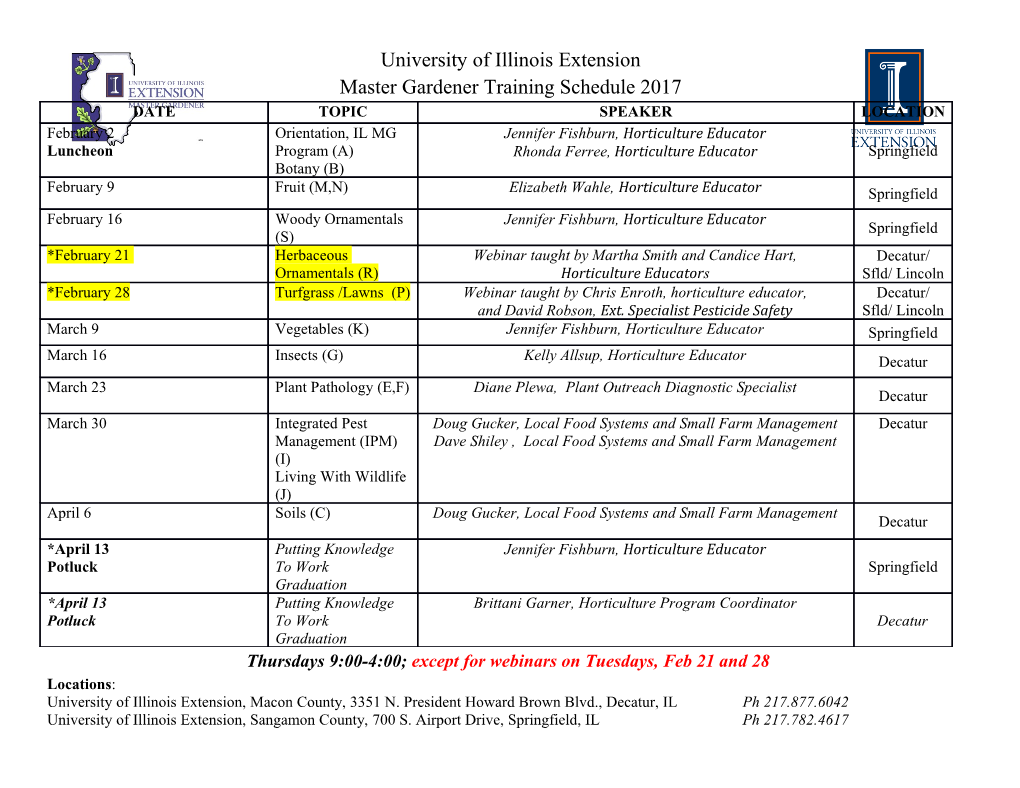
Lecture Notes Introduction to Support Vector Machines Raj Bridgelall, Ph.D. Overview A support vector machine (SVM) is a non-probabilistic binary linear classifier. The non- probabilistic aspect is its key strength. This aspect is in contrast with probabilistic classifiers such as the Naïve Bayes. That is, an SVM separates data across a decision boundary (plane) determined by only a small subset of the data (feature vectors). The data subset that supports the decision boundary are aptly called the support vectors. The remaining feature vectors of the dataset do not have any influence in determining the position of the decision boundary in the feature space. In contrast with SVMs, probabilistic classifiers develop a model that best explains the data by considering all of the data versus just a small subset. Subsequently, probabilistic classifiers likely require more computing resources. The binary and linear aspects, however, are two SVM limitations. Recent advances using a “Kernel Trick” have addressed the linearity restriction on the decision boundary. However, the inability to classify data into more than two classes is still an area of ongoing research. Methods so far involve creating multiple SVMs that compare data objects among themselves in a variety of ways, such as one-versus-all (OVA) or all-versus-all (AVA) (Bhavsar and Ganatra 2012). The latter is also called one-versus-one (OVO). For k classes, OVA requires training k classifiers so that each class discriminates against the remaining k-1 classes. AVA requires k(k-1)/2 classifiers because each class is discriminated against every other class, for all possible pairings. After constructing the number of required binary classifiers for either method, a new object is classified based on the comparison that provides the largest discriminant value. The SVM separates all data objects in a feature space into two classes. The data objects must have features {x1 ... xn} and a class label, yi. SVM treats each data object as a point in feature space such that the object belongs to one class or the other. Specifically, a data object (characterized by its feature vector) either belongs to a class, in which case the class label is yi = 1, or it does not belong to the class (implying that it belongs to the other class) in which case the class label is yi = -1. Therefore, the definition for the data is n Data x , y | x p , y 1,1 (1) i i i i i1 where p is the dimension of the feature vector and n is the number of data points. During training, the SVM classifier finds a decision boundary in the feature space that separates the data objects into the two classes. The optimization problem is to find the decision boundary (a linear hyperplane) that has the maximum separation (margin) between the two classes. The margin of a hyperplane is the distance between parallel equidistant hyperplanes on either side of the hyperplane such that the gap is void of data objects. The optimization during training finds a hyperplane that has the maximum margin. The SVM then uses that hyperplane to predict the class of a new data object once presented with its feature vector. Dr. Raj Bridgelall 9/2/2017 Page 1/18 Lecture Notes: Introduction to Support Vector Machines Hyperplane Definition In geometry, a hyperplane is a subspace that has one dimension fewer than its ambient space. The hyperplane separates the space into two parts. A classifier is linear when it uses a hyperplane in multidimensional space to separate data. From geometry, the general equation for a hyperplane is w x 0 (2) For example, in a 2D space the equation of a hyperplane is a line where y ax b (3) Rewriting the equation for the line yields the standard form for the hyperplane, which is y axb 0 (4) The equivalent vector notation is b 1 w a and x x (5) 1 y where w0 = -b, w1 = -a, and w2 = 1. Taking the dot product of w and x and setting that equal to zero then produces the equation of a line in standard form. Given that the vectors are column vectors, the dot product is equivalent to the matrix operation wT x 0 (6) The vector and matrix forms are easier to work with when using matrix algebra within numerical packages. Some notations explicitly write out the w0 = -b component of the vector, in which case the dimension index starts at 1 instead of 0. The w vector is always normal to the hyperplane (Dawkins 2017). Its unit vector u is w u (7) w The vector w must be perpendicular (normal) to the hyperplane H0 because the dot product is zero by definition, when the cosine of the angle between x and w is zero. The cosine is zero when the angle is 90 degrees. For the 2D example, w w w u 1 , 2 (8) w w w This unit w vector is important in finding the distance of any point (feature) from the hyperplane by projecting that point to a normal vector of the hyperplane. For example, vector p in Figure 1 is the projection of point A onto the plane of the w vector. Hence the distance from point A to the hyperplane is the same as the length of p or ||p||. The projection of vector a onto the plane of w is p where p u au (9) The dot product produces a scalar, which is the magnitude (length) of the vector such that Dr. Raj Bridgelall 9/2/2017 Page 2/18 Lecture Notes: Introduction to Support Vector Machines u a uiai (10) i and the direction of the vector is u. Figure 1: Projection of a vector to compute the distance to a hyperplane. Margin of a Hyperplane The margin of a hyperplane is twice the length (norm) of the projection of the nearest point such that m 2 p (11) The norm of a vector is the square root of the sum of squares of all the elements in the vector such that 2 p pi (12) i Optimal Hyperplane As shown in Figure 2, the plane bounded by the margin along the hyperplane is void of data points. The optimal hyperplane is simply one that provides the largest margin m. As noted before, the support vectors are at the boundaries (H1 and H2) of the plane that the hyperplane equally bisects. Figure 2 indicates the support vectors with thick black borders. The data might be linearly or non-linearly separable. For linearly separable data, the problem amounts to maximizing the margin of a hyperplane H0 that separates the data. Handling non-linearly separable features require a transformation of the features to a higher dimension space by using kernel functions (covered later). The general solution uses linear Kernels for linearly separable feature spaces. Constraints of Classification Given a weight vector w, a parallel hyperplane with offset +δ to one side is Dr. Raj Bridgelall 9/2/2017 Page 3/18 Lecture Notes: Introduction to Support Vector Machines w x (13) Hence, the equal and opposite offset to the other side of the hyperplane is w x (14) Given a feature vector xi, the assigned class must satisfy w xi (15) for a class label of yi = 1 and w xi (16) for a class label of yi = -1. Figure 2: Maximizing the margin of a hyperplane. The SVM will calculate the optimum w for any offset. Therefore, any non-zero offset will establish a margin that the optimization can maximize. For mathematical convenience, theoreticians set the offset to δ=1 to simplify the design of the classifier. This allows the two classification constraints to be combined into a single constraint. That is, setting δ=1, multiplying both sides of equation (15) by yi, and assigning the class label value of +1 to the right yields yi w xi 1(1) yi w xi 1 (17) Doing the same for equation (16) with its yi label value at -1 yields yi w xi 1(1) yi w xi 1 (18) Note that per rule for inequalities, multiplying by negative 1 flips the inequality sign. Hence, the “mathematical convenience” produced the single constraint Dr. Raj Bridgelall 9/2/2017 Page 4/18 Lecture Notes: Introduction to Support Vector Machines yi w xi 1 1 i n (19) Equation (19) becomes the constraint in the optimization problem to ensure that the margin is void of data points. Training the SVM involves determining the hyperplane by solving for w that provides the maximum margin, given the data labels yi and feature vectors xi. Distance Between Hyperplanes As noted before, the vector w is perpendicular (normal) to the hyperplane H0. Therefore, its unit vector u = w/||w|| must be perpendicular to H0 with magnitude 1. The vector du is the perpendicular vector between hyperplane H0 and a parallel hyperplane separation H1 some distance d away. Let x0 be the base coordinate of the du vector on the hyperplane, and z0 be the tip coordinate, which must line on the hyperplane H1. Therefore, the distance vector is z0 x0 du (20) The fact that z0 is on H1 means that w z0 1 (21) Substituting z0 from Equation (20) yields w x0 du 1 (22) Substituting u from Equation (7) yields w w x d 1 0 (23) w Expanding Equation (23) yields w w w x0 d 1 (24) w Given that w w w 2 (25) Equation (24) becomes w x0 d w 1 (26) Hence, w x0 1 d w (27) The fact that x0 is on H0 means that w x0 0 (28) Substituting Equation (28) into Equation (27) yields 0 1 d w (29) Solving for d yields Dr.
Details
-
File Typepdf
-
Upload Time-
-
Content LanguagesEnglish
-
Upload UserAnonymous/Not logged-in
-
File Pages18 Page
-
File Size-