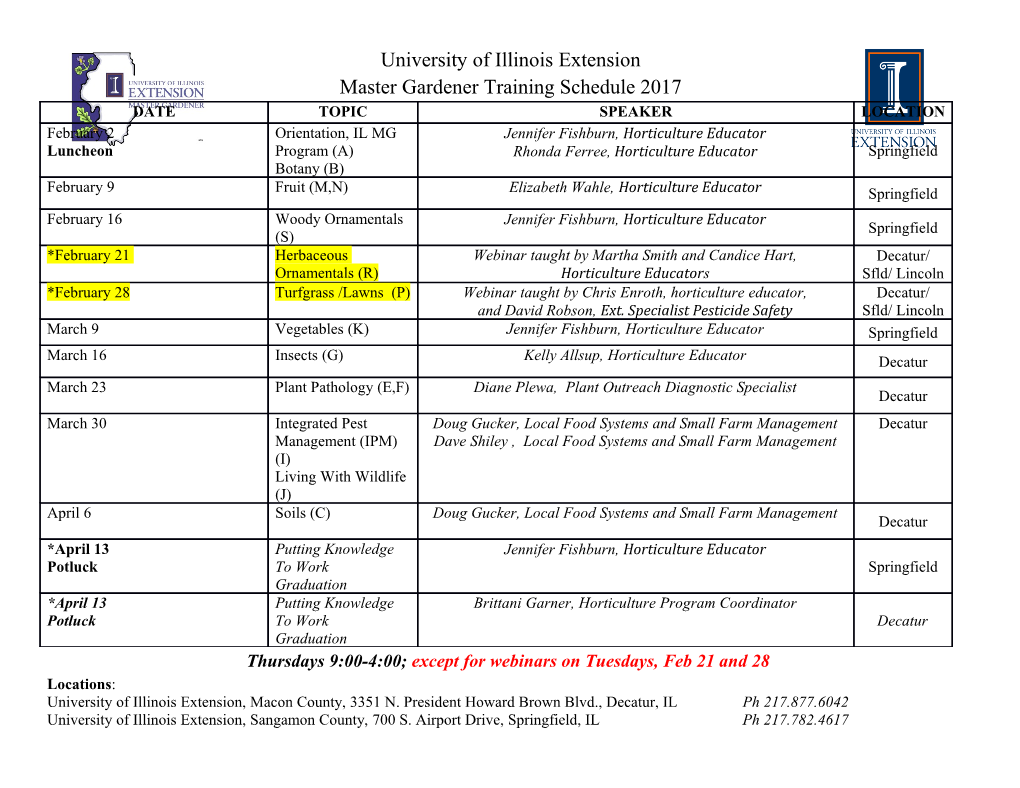
The Fourth International Workshop on Knowledge Discovery from Data Streams The rapid growth in information science and technology in general and the com- plexity and volume of data in particular have introduced new challenges for the research community. Databases are growing incessantly and many sources pro- duce data continuously. In most real world applications, the process generating the data is not strictly stationary. In many cases, we need to extract some sort of knowledge from this continuous stream of data. Examples include customer click streams, telephone records, large sets of web pages, multimedia data, scientific data, and sets of retail chain transactions. These sources are called data streams. Learning from data streams is an incremental task that requires incremental al- gorithms that take drift into account. The goal of this workshop is to promote an interdisciplinary forum for re- searchers who deal with sequential learning, anytime learning, real-time learn- ing, online learning, temporal and spatial learning, etc. from data streams. The workshop papers present significant contributions in learning decision trees, asso- ciation rules, clustering, preprocessing, feature selection, etc. from data streams and related themes. This is the fourth workshop in this series. The workshop web page is avail- able at http://www.machine-learning.eu/iwkdds-2006/. There were 17 sub- missions. The program committee accepted 9 full papers, 4 short papers and 1 poster paper. This year the workshop has a special emphasis on learning from sensor net- works in ubiquitous environments. In that direction, the workshop includes also an invited talk, by Hillol Kargupta, one of the leading researchers in distributed mining data streams. We would like to thank the ECML/PKDD 2006 organizers, the authors of the submitted papers, and the members of the Program Committee for all efforts to make this workshop possible. J. Gama, R. Klinkenberg and J. Aguilar Organization Program Chairs Jo˜aoGama, University of Porto, Portugal Ralf Klinkenberg, University of Dortmund, Germany Jes´usS. Aguilar-Ruiz, University of Seville, Spain Program Committee Michaela Black, University of Ulster, Coleraine, Northern Ireland, UK Andre Carvalho, University of Sao Paulo, Brazil Pedro Domingos, University of Washington, Seattle, WA, USA Francisco Ferrer, University of Seville, Spain Mohamed Gaber, Monash University, Victoria, Australia Jo˜aoGama, University of Porto, Portugal Ray Hickey, University of Ulster, Coleraine, Northern Ireland, UK Hillol Kargupta, University of Maryland, Baltimore, MD, USA Ralf Klinkenberg, University of Dortmund, Germany Jeremy Z. Kolter, Stanford University, CA, USA Miroslav Kubat, University Miami, FL, USA Mark Last, Ben-Gurion University, Israel Mark Maloof, Georgetown University, Washington, DC, USA S. Muthu Muthukrishnan, Rutgers University and AT&T Research, USA Masayuki Numao, Osaka University, Japan Pedro Pereira Rodrigues, University of Porto, Portugal Josep Roure, Carnegie Mellon University, Pittsburgh, PA, USA Jes´usS. Aguilar-Ruiz, University of Pablo de Olavide, Spain Bernhard Seeger, University Marburg, Germany Elaine Parros Sousa, University of Sao Paulo, Brazil Min Wang, IBM Watson Research Center, Hawthorne, NY, USA Wei Wang, University of North Carolina, Chapel Hill, NC, USA Xiaoyang Wang, University of Vermont, Burlington, VT, USA Gerhard Widmer, University of Linz, Austria Philip S. Yu, IBM Watson Research Center, Yorktown Heights, NY, USA Sponsors The workshop is supported by Kdubiq - Knowledge Discovery in Ubiquitous Environments - European Coordinated Action of FP6 and Adaptive Learning Systems II Project (POSI/EIA/55340/2004). ii Table of Contents Invited Talk Peer-to-Peer Distributed Data Stream Mining and Monitoring Hillol Kargupta, University of Maryland, Baltimore, MD, USA . 1 Contribution Papers Accurate Schema Matching on Streams Szymon Jaroszewicz, Lenka Ivantysynova and Tobias Scheffer ................3 High-Order Substate Chain Prediction Based on Massive Sensor Outputs Nguyen Viet Phuong and Takashi Washio...................................13 Online Prediction of Clustered Streams Pedro Pereira Rodrigues and Joao Gama....................................23 Model Averaging via Penalized Regression for Tracking Concept Drift Kyupil Yeon, Moon Sup Song, Yongdai Kim and Cheolwoo Park. .33 Incremental Training of Markov Mixture Models Andreas Kakoliris and Konstantinos Blekas .................................47 Improving Prediction Accuracy of an Incremental Algorithm Driven by Error Margins Jos´edel Campo-Avila´ , Gonzalo Ramos-Jim´enez, Jo˜aoGama and Rafael Morales- Bueno ......................................................................57 Analyzing Data Streams by Online DFT Alexander Hinneburg, Dirk Habich and Marcel Karnstedt....................67 Early Drift Detection Method Manuel Baena-Garcia, Jos´edel Campo-Avila´ , Ra´ulFidalgo-Merino, Albert Bifet, Ricard Gavald`a and Rafael Morales-Bueno .................................77 Mining Frequent Items in a Stream using Flexible Windows Toon Calders, Nele Dexters and Bart Goethals ..............................87 iii Hard Real-time Analysis of Two Java-based Kernels for Stream Mining Rasmus Pedersen ...........................................................97 Dynamic Feature Space and Incremental Feature Selection for the Classification of Textual Data Streams Ioannis Katakis, Grigorios Tsoumakas and Ioannis Vlahavas. .107 User Constraints over Data Streams Carlos Ruiz Moreno, Myra Spiliopoulou and Ernestina Menasalvas . 117 StreamSamp DataStream Clustering Over Tilted Windows Through Sampling Baptiste Csernel, Fabrice Clerot and Georges H´ebrail . 127 Structural Analysis of the Web Pratyus Patnaik and Sudip Sanyal .........................................137 Author Index. .145 iv Peer-to-Peer Distributed Data Stream Mining and Monitoring Hillol Kargupta University of Maryland - Baltimore County, Baltimore, MD, USA (Invited Talk) Abstract: Distributed data mining (DDM) deals with the problem of analyzing distributed, possibly multi-party data by paying attention to the computing, communication, storage, and human factors-related issues in a distributed environment. Unlike the conventional off-the-shelf centralized data mining products, DDM systems are based on fundamentally distributed algorithms that do not necessarily re- quire centralization of data and other resources. DDM technology is finding in- creasing number of applications in many domains. Examples include data driven pervasive applications for mobile and embedded devices, grid-based large scale scientific and business data analysis, security and defense related applications involving analysis of multi-party possibly privacy-sensitive data, and peer-to- peer data stream mining in sensor and file-sharing networks. This talk will focus on peer-to-peer (P2P) distributed data stream mining and monitoring. It will first discuss the foundation of approximate and exact P2P algorithms for data analysis. Then it will present a class of P2P algorithms for eigen-analysis and clustering in details. The talk will end with a discussion on the future directions of research on P2P data mining. 1 2 ÙÖØ ËÑ ÅØ Ò ÓÒ ËØÖÑ× ½ ¾ ¾ ËÞÝÑÓÒ ÂÖÓ×ÞÛÞ ¸ ÄÒÁÚÒØÝ×ÝÒÓÚ ¸ÌÓ× Ë«Ö ½ ÆØÓÒÐ ÁÒרØÙØ Ó Ì Ð ÓÑÑÙÒ ØÓÒ׸ ÏÖ×Û¸ ÈÓÐÒ¸ ×׺ÙѺ Ù ¾ ÀÙÑ ÓÐØ¹ÍÒÚ Ö×ØØ ÞÙ ÖÐÒ¸ ÖÑÒÝ Ú ÒØÝ×ݸ×Ö ÒÓÖÑ Ø ºÙ¹ ÖÐ Òº ר֨º Ï Ö ×× Ø ÔÖÓÐ Ñ Ó ÑØ Ò! ÑÔ Ö ØÐÝ Ó Ù¹ Ñ ÒØ × Ñ× Ó Ø ×ØÖ Ñ× Ò ÐÖ! Ø× ׺ ÁÒ×ØÒ ¹Ð Ú Ð × Ñ ÑØ Ò! Ð!ÓÖØÑ× ÒØ Ý Ð# ÐÝ ÓÖÖ ×ÔÓÒ Ò × ØÛ Ò Ø¹ ØÖÙØ × Ý ÕÙÒØ ÝÒ! Ø ×ÑÐÖØÝ Ó Ø Ö ÓÖÖ ×ÔÓÒÒ! ÚÐ٠׺ ÀÓÛ¹ Ú Ö¸ Ü Ø Ð ÙÐØÓÒ Ó Ø × ×ÑÐÖØ × Ö ÕÙÖ × ÔÖÓ ××Ò! Ó ÐÐ Ø× Ö ÓÖ×&Û × Ò ×Ð ÓÖ Ø ×ØÖ Ñ׺ Ï Ú× ×Ø ÑØ Ò! Ð!ÓÖØÑ ØØ Ù× × ÓÒÐÝ ×ÑÐÐ ×ÑÔÐ Ó Ö ÓÖ׸ Ò × Ý Ø !ÙÖÒØ ØÓ ÑØ Ø ÑÓר ×ÑÐÖ ØØÖÙØ × ÛØ ! ÔÖÓÐØÝº Ì Ñ ØÓ Ò ÔÔÐ ØÓ ÒÝ !Ú Ò ´ ÓÑÒØÓÒ Ó µ ×ÑÐÖØÝ Ñ ØÖ × ØØ Ò ×ØÑØ ÖÓÑ ×ÑÔÐ ÛØ ÓÙÒ ÖÖÓÖ) Û ÔÔÐÝ Ø Ð!ÓÖØÑ ØÓ × Ú ÖÐ Ñ ØÖ ׺ Ï !Ú Ö!ÓÖÓÙ× ÔÖÓ Ó Ó Ø Ñ ØÓ ³× ÓÖÖ ØÒ ×× Ò Ö ÔÓÖØ ÓÒ ÜÔ ÖÑ Ò Ø× Ù×Ò! ÐÖ! Ø× ׺ ½ ÁÒØÖÓ ÙØ ÓÒ ÁÒ ÑÒÝ ÔÖØÐ ×ØÙØÓÒ׸ Ø ÑÒÒ ÔÖÓ ××× Ö ÔÖ ÝÔÖÔÖÓ¹ ××Ò ×ØÔ Ò Û ÑÙÐØÔÐ ØÖÓÒÓÙ× ØÖÒרÓÒ Ø×× Ö ÒØ¹ ÖØ ÒØÓ ×ÒÐ Ø ÛÖÓÙ׺ ÁÒØÖØÓÒ ÖÕÙÖ× Ø ÒØ¬ØÓÒ Ó ×¹ ÑÒØ ÓÖÖ×Ô ÓÒÒ× ØÛÒ ØØÖÙØ× ÖÓ×× ÑÙÐØÔÐ ØÖÒרÓÒ Ø× ×Ñ× ¾º ÁÒ ÔÖØÐ ÔÔÐØÓÒ× ØØ ÓØÒ ÒÚÓÐÚ ÐÖ Ø×׸ ×Ñ ÑØÒ × ØÓÙר׏ ÓØÒ ÙÖØÖ ÒÖ Ý ÆØ Ó ÙÑÒØØÓÒº Ê×Ö ÖÚÓÐÚ× ÖÓÙÒ Ø ÕÙרÓÒ ÓÛ ×Ñ ÑØÒ Ò ×ÙÔ¹ Ô ÓÖØ¸ ÓÖ ÚÒ ÙØÓÑØ¸ «ØÚÐݺ ËÑ ÑØÒ ÐÓÖØÑ× ÒÖÐÐÝ ÑØ ÐÑÒØ× Ó Ø ×ØÒØ ×Ñ× ×Ù ØØ ×ÓÑ ×ÑÐÖØÝ ÖØÖÓÒ × ÑÜÑÞº ËѹÐÚÐ ÑØÖ× ÙØÐÞ ×ÑÐÖØÝ ÖØÖÓÒ ØØ ÖÖ× ØÓ ×Ñ ÒÓÖÑØÓÒ ×Ù × ØØÖÙØ ÒÑ׸ ×ÖÔØÓÒ׸ ÓÖ Ø ×Ñ׳ רÖÙ¹ ØÙÖº ÍÒÓÖØÙÒØÐݸØÚÐÐ ×Ñ ÒÓÖÑØÓÒ × ÓØÒ ÆØº ×× Ö× Ò Û ØØÖÙØ ÒÑ× Ö ÓÔÕÙ Ò ÐÙ× ÓÒ ØÖ ×ÑÒØ× Ò ÓÒÐÝ ÓÙÒ Ý Ò×Ô ØÒ Ø ØØÖÙØ×³ ÚÐÙ׺ ÁÒ×ØÒ¹ÐÚÐ ×Ñ ÑØÖ× ÕÙÒØÝ Ø ×ÑÐÖØÝ Ó ØØÖÙØ× Ý ÓÑÔÖÒ ÔÖÓÔ ÖØ× Ó ØÖ ÚÐÙ׺ ÁÒ ÓÖÖ ØÓ ÙÖÒØ ØØ Ø ÔÖÓ Ù ÑÔÔÒ Ò Ø ÑÜÑÞ× Ø Ó×Ò ×ÑÐÖØÝ ÙÒØÓÒ¸ Ò×ØÒ¹ÐÚÐ ÑØÖ× Ò ØÓ ÜÖ× Ø Ðר ÓÒ ÒØÖ Ô×× ÓÚÖ Ø Ø×׺ ÓÑÔÐØ Ô×× × ÙÒÖ×ÓÒÐ ÓÖ ØÖÒרÓÒÐ Ø×× ØØ ÖÓÖ ¹×Ô Ø ×ØÖÑ× × ØÝ Ó ÙÖ¸ ÓÖ Ò×ØÒ¸ Ò ØÐÓÑÑÙÒØÓÒ Ò ÒÒ ÔÔÐØÓÒ× Ò Ò ×ÐÝ ÖØÐ Ò׸ Ò ÖÓÛÒØÓ Ø ÓÖÖ Ó ÙÒÖ× Ó ØÖÝØ×º Ì ¹Ó ×ÓÐÙØÓÒ Ó ÔÖÓ ××Ò 3 ÓÒÐÝ ×ÑÐÐ ×ÑÔÐ Ó ØÖÒרÓÒ× Ö×ÙÐØ× Ò ÐÓ×× Ó ÒÝ ÙÖÒØ ÓÒ Ø ÓÔØÑÐØÝ Ó Ø ÔÖÓ Ù ÑØº ÃÒÓÛÒ ÑØÓ × ØØ ÙØÐÞ ×ÑÔÐÒ Ó ÒÓØ ÙÖÒØ ÒÝ ÔÖÓÔ ÖØ× Ó Ø ÖØÖÚ ÑÔÔÒ׺ Ï ÓÖÑÐÞ Ø ÑØÒ ÔÖÓÐÑ Ò ÛÝ ØØ × ÓØ ÑØÑØÐÐÝ ÖÓÖÓÙ× Ò ÐÓ×ÐÝ Ø ØÓ ÔÖØÐ ÔÔÐØÓÒ׺ Ï Ú× ×Ñ ÑØÒ ÐÓÖØÑ¸ Û× ÙÖÒØ ØÓ ÔÖÓ Ù ÒÖ¹ÓÔØÑÐ ÑØ Û ØÐ Ø ØÖÑ ÒÖ¹ÓÔØÑÐ Ý ÑÒ× Ó ÔÖÓÐר ÓÙÒ× ÓÒ ØØÖÙØ ×ÑÐÖØÝº Ù×ÙÐ ×ÑÐÖØÝ ÑØÖ ÓÖ Ø ÑØÒ ÔÖÓÐÑ Ø Ò × × ÖÕÙÖ¹ ÑÒØ ÓÖ ÒÝ ×ÑÔÐÒ ÐÓÖØÑ¸ Ò ×ÒÒ ×Ù ÔÖÓÐѹ×Ô ¬ ÑØÖ ÐÖÐÝ × ÐÐÒÒ ××Ù Ò Ø×к ÌÖÓÖ¸ Û ×Ò ÓÙÖ ×ÓÐÙØÓÒ ØÓ ÒÖ ÛØ Ö×Ô Ø ØÓ Ø ×ÑÐÖØÝ ÖØÖÓÒ ÛØ ÓÑÒØÓÒ× Ó ×ÚÖÐ ×ѹÐÚÐ Ò Ò×ØÒ¹ÐÚÐ ÖØÖ Ò
Details
-
File Typepdf
-
Upload Time-
-
Content LanguagesEnglish
-
Upload UserAnonymous/Not logged-in
-
File Pages149 Page
-
File Size-