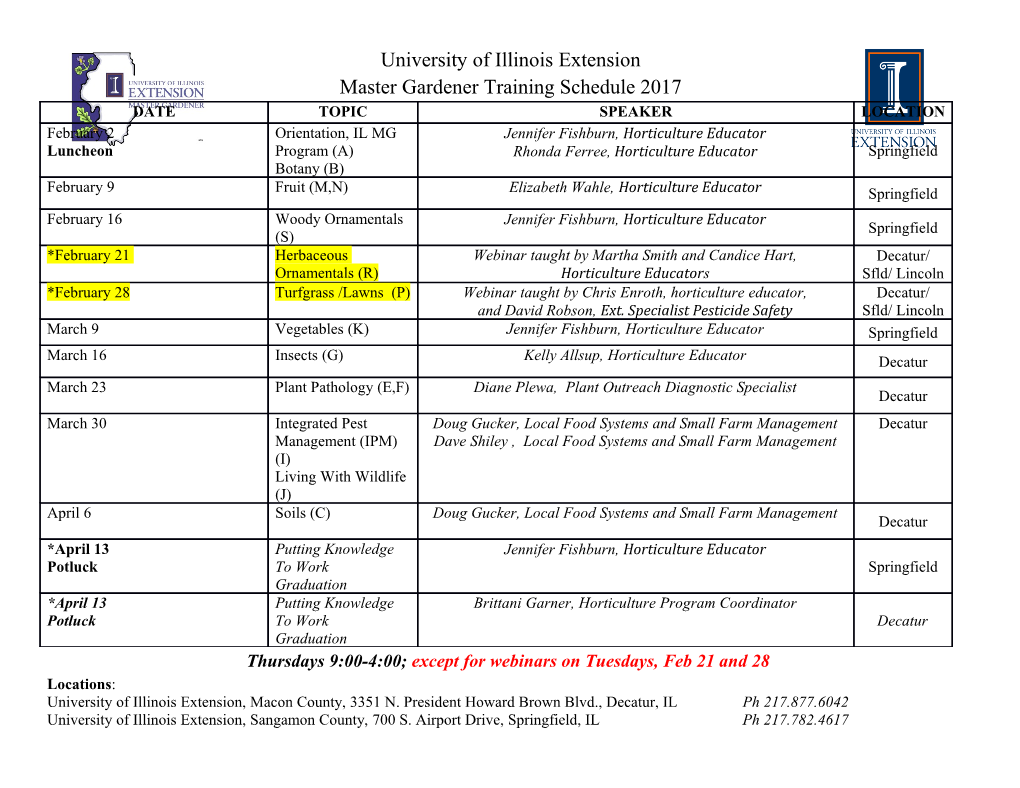
NBER WORKING PAPER SERIES ASSESSING EXTERNAL VALIDITY Hao Bo Sebastian Galiani Working Paper 26422 http://www.nber.org/papers/w26422 NATIONAL BUREAU OF ECONOMIC RESEARCH 1050 Massachusetts Avenue Cambridge, MA 02138 November 2019 We thank Matias Cattaneo, Guido Kuersteiner, and Owen Ozier for their very valuable comments. The views expressed herein are those of the authors and do not necessarily reflect the views of the National Bureau of Economic Research. NBER working papers are circulated for discussion and comment purposes. They have not been peer- reviewed or been subject to the review by the NBER Board of Directors that accompanies official NBER publications. © 2019 by Hao Bo and Sebastian Galiani. All rights reserved. Short sections of text, not to exceed two paragraphs, may be quoted without explicit permission provided that full credit, including © notice, is given to the source. Assessing External Validity Hao Bo and Sebastian Galiani NBER Working Paper No. 26422 November 2019, Revised June 2020 JEL No. C18,C52,C93 ABSTRACT In designing any causal study, steps must be taken to address both internal and external threats to its validity. Researchers tend to focus primarily on dealing with threats to internal validity. However, once they have conducted an internally valid analysis, that analysis yields an established set of findings for the specific case in question. As for the future usefulness of that result, however, what matters is its degree of external validity. In this paper we provide a formal, general exploration of the question of external validity and propose a simple and generally applicable method for evaluating the external validity of randomized controlled trials. Although our method applies only to RCTs, the issue of external validity is general and not restricted to RCTs, as shown in our formal analysis. Hao Bo Department of Economics University of Maryland Tydings Hall College Park, MD 20740 [email protected] Sebastian Galiani Department of Economics University of Maryland 3105 Tydings Hall College Park, MD 20742 and NBER [email protected] 1. Introduction In designing any causal study, steps must be taken to address both internal and external threats to its validity (see Campbell, 1957, and Cook and Campbell, 1979). Researchers tend to focus primarily on threats to internal validity, i.e., determining whether it is valid to infer that, within the context of a particular study, the differences in the dependent variables are caused by the differences in the relevant explanatory variables. External validity, on the other hand, concerns the extent to which a causal relationship holds over variations in persons, settings, and time. It is important to underscore the fact at the outset that external validity does not extend to modifications in the treatment, although in practice, researchers often try to generalize their results by conflating the two levels of generalization into a question of external validity. Randomized controlled trials solve the problem of selection bias in the identification of causal effects. Thus, theoretically, cause-effect constructs identified by means of randomized controlled trials are internally valid, that is, they permit the identification of causal effects for the population from which the random sample used in the estimation was drawn. The outcomes of such experiments are interesting in their own right, but researchers sometimes explicitly assume external validity (EV), i.e., that the internally valid estimates obtained for one population can be extrapolated to other populations. In fact, it is not uncommon that after researchers have established a cause-and-effect relationship in a specific population, they proceed to discuss its implications based on the assumption that this relationship is generally valid. In this paper, we formalize the concept of external validity and show that in general, it is unlikely that any given study will be externally valid in any general sense. This is one reason why Manski (2013) says that the current practice of policy analysis “hides uncertainty”. Once researchers have conducted an internally valid analysis, that analysis yields an established set of findings for the specific case in question. As for the future 1 usefulness of that result, however, what matters is its degree of EV. The most commonly held view in this regard is that the EV problem hinges on assumptions about the relationship between the population for which internally valid estimates have been obtained and another, different population. Apart from researchers who are focusing on EV in a specific context, many researches either ignore the EV problem altogether or approach it subjectively. In this paper, we provide a formal and general reflection on the EV problem and propose a simple and generally applicable method for evaluating the external validity of randomly controlled trials (RCTs). In this paper we define external validity as the stability of the conditional distribution p(outcomes | treatment) across different populations. We then formalize the degree to which we can make judgments about a new population (density) generated as a subpopulation from an overarching population that also generates the “original” population for which there is an internally valid estimate. Without loss of generality, assume that we have data that allows estimation of the joint distribution p(outcomes, treatment). We then have p(outcomes, treatment) = p(outcomes | treatment) × p(treatment). We say that there is external validity if, for other data with a potentially different joint distribution of outcomes and treatment, the conditional distribution p(outcomes | treatment) stays the same. Our definition of external validity is the same as that of Janzing, Peters, and Schölkopf (2017). Admittedly, this seems quite stringent. It might be thought that, even with a moderate change of p(outcome | treatment) across different populations, external validity could be maintained. But what exact degree of change in p(outcome | treatment) leads to EV or external invalidity cannot be precisely defined. We need an operationalizable definition of EV, so, in line with a small body of literature (Janzing, Peters, and Schölkopf, 2017), we err on the side ofcaution, although we admit that there are other ways of defining EV that provide interesting and important insights, e.g., Meager (2019). Based on our theoretical framework, we then propose two alternative measures of external validity. To the best of our knowledge, we are the first to propose formal mathematical definitions of external validity and, on that basis and in the context of an RCT, to propose purely data-driven measures related to theoretical constructs. 2 The measures of EV we propose in this paper can take advantage of multiple trials to evaluate the degree to which certain empirical conclusions are valid across different populations. Needless to say, ultimately, the external validity of all causal estimates is established by replication in other datasets (Angrist, 2004).2 We would like to determine whether a given study or a given set of studies can be generalized to other populations in general.3 In order to do that, we propose a method that applies to RCTs, but it should be noted that the issue of external validity is general and not restricted to RCTs, as shown in our formal and general reflection below. The rest of this paper is structured as follows. In Section 2, we provide a formal and general reflection on the EV problem. Based on the model described in that section, in Section 3 we propose a simple and generally applicable method for assessing the external validity of RCTs. Finally, we present final remarks. 2. External Validity A single experiment (or a set of experiments regarded as a single experiment)4 allows us to arrive at a point estimate for the population of cause-effect parameters. Assessing the EV of one causal parameter entails estimating treatment effects as a function of different populations. Thus, evaluating the EV of an internally valid estimate of a cause-effect parameter entails assessing a distribution of cause-effect 2 In the areas of labor and development economics, a number of studies use similar multi- country strategies to generalize cause-and-effect constructs. For example, Cruces and Galiani (2007) examine the effects of fertility on labor outcomes in three countries; Dehejia, Pop- Eleches, and Samii (2019) examine the causal effects of sibling sex composition on fertility and labor supply across many countries and years and characterize how its effects vary in terms of available covariates; Banerjee et al. (2015) study microcredit in six countries; Galiani et al. (2017) study the effects of sheltering the poor in three countries; Gertler et al. (2015) study health promotion in four countries; and Dupas et al. (2016) examine the effects of opening savings accounts in three different countries. 3 For example, Deaton (2010) writes: “We need to know when we can use local results, from instrumental variables, from RCTs, or from nonexperimental analyses, in contexts other than those in which they were obtained.” 4 When we have a set of experiments and we reach a conclusion from them, we have to find a way to aggregate their outcomes from the experiments so they can be regarded as a single experiment (correspondingly, behind that single experiment there would be a single population formed by a mixture of the populations underlying the different original experiments). However, in this paper we do not discuss
Details
-
File Typepdf
-
Upload Time-
-
Content LanguagesEnglish
-
Upload UserAnonymous/Not logged-in
-
File Pages30 Page
-
File Size-