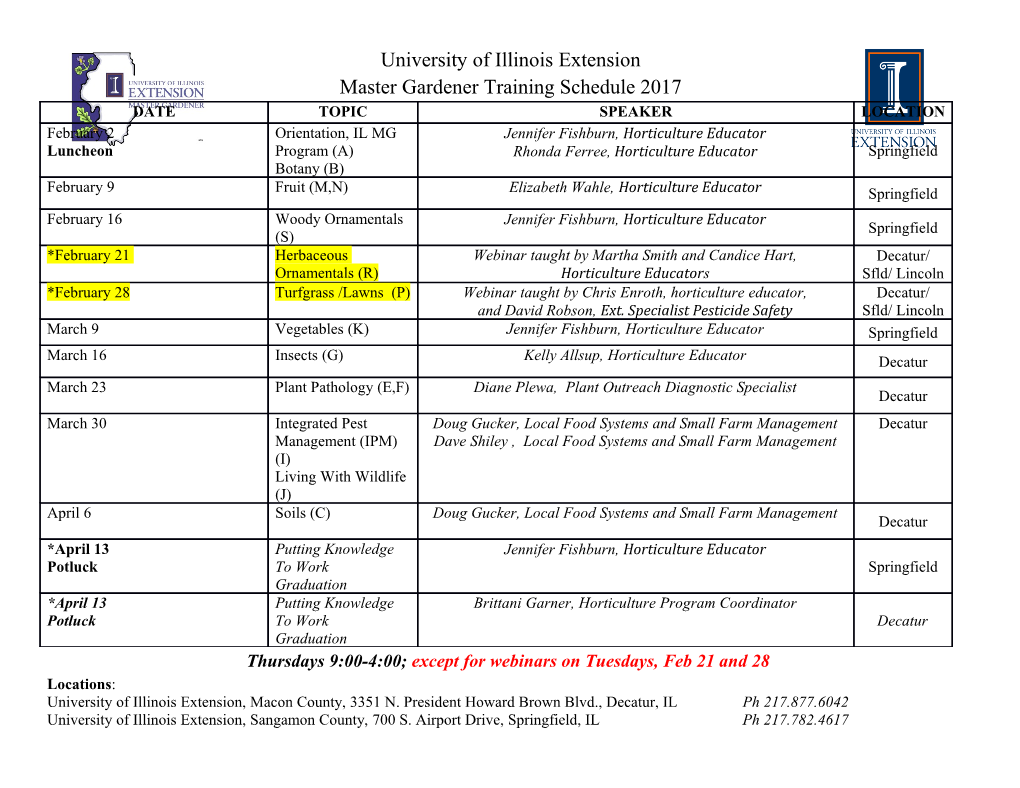
Liao et al. Zool. Res. 2021, 42(4): 492−501 https://doi.org/10.24272/j.issn.2095-8137.2021.141 Article Open Access 3DPhenoFish: Application for two- and three- dimensional fish morphological phenotype extraction from point cloud analysis Yu-Hang Liao1,#, Chao-Wei Zhou2,3,#, Wei-Zhen Liu1, Jing-Yi Jin4, Dong-Ye Li4, Fei Liu2, Ding-Ding Fan2, Yu Zou4, Zen-Bo Mu2, Jian Shen5, Chun-Na Liu6, Shi-Jun Xiao1,2,4,*, Xiao-Hui Yuan1,*, Hai-Ping Liu2,* 1 Department of Computer Science, Wuhan University of Technology, Wuhan, Hubei 430070, China 2 Institute of Fisheries Science, Tibet Academy of Agricultural and Animal Husbandry Sciences, Lhasa, Tibet 850000, China 3 Key Laboratory of Freshwater Fish Reproduction and Development (Ministry of Education), College of Fisheries, Southwest University, Chongqing 402460, China 4 Jiaxing Key Laboratory for New Germplasm Breeding of Economic Mycology, Jiaxing, Zhejiang 314000, China 5 Huadian Tibet Energy Co., Ltd, Lhasa, Tibet 851415, China 6 China Institute of Water Resources and Hydropower Research, Beijing 100038, China ABSTRACT obtained in a visualized manner. Intuitive fine-tuning Fish morphological phenotypes are important of key points and customized definitions of resources in artificial breeding, functional gene phenotypes are also allowed in the software. Using mapping, and population-based studies in 3DPhenoFish, we performed high-throughput aquaculture and ecology. Traditional morphological phenotyping for four endemic Schizothoracinae measurement of phenotypes is rather expensive in species, including Schizopygopsis younghusbandi, terms of time and labor. More importantly, manual Oxygymnocypris stewartii, Ptychobarbus dipogon, measurement is highly dependent on operational and Schizothorax oconnori. Results indicated that experience, which can lead to subjective the morphological phenotypes from 3DPhenoFish phenotyping results. Here, we developed exhibited high linear correlation (>0.94) with manual 3DPhenoFish software to extract fish morphological measurements and offered informative traits to phenotypes from three-dimensional (3D) point cloud discriminate samples of different species and even data. Algorithms for background elimination, for different populations of the same species. In coordinate normalization, image segmentation, key summary, we developed an efficient, accurate, and point recognition, and phenotype extraction were customizable tool, 3DPhenoFish, to extract developed and integrated into an intuitive user morphological phenotypes from point cloud data, interface. Furthermore, 18 key points and traditional which should help overcome traditional challenges in 2D morphological traits, along with 3D phenotypes, including area and volume, can be automatically Received: 24 April 2021; Accepted: 05 July 2021; Online: 06 July 2021 This is an open-access article distributed under the terms of the Foundation items: This work was supported by the National Natural Creative Commons Attribution Non-Commercial License (http:// Science Foundation of China (32072980) and Key Research and creativecommons.org/licenses/by-nc/4.0/), which permits unrestricted Development Projects in Tibet (XZ202001ZY0016N, XZ201902NB02, non-commercial use, distribution, and reproduction in any medium, XZNKY-2019-C-053) # provided the original work is properly cited. Authors contributed equally to this work Copyright ©2021 Editorial Office of Zoological Research, Kunming *Corresponding authors, E-mail: [email protected]; yuanxiaohui@ Institute of Zoology, Chinese Academy of Sciences whut.edu.cn; [email protected] manual measurements. 3DPhenoFish can be used volume, are difficult to measure manually. With the continued for research on morphological phenotypes in fish, development of computer vision technology, sensor-based 3D including functional gene mapping, artificial reconstruction methods have been increasingly used in life selection, and conservation studies. 3DPhenoFish is sciences and agriculture (Comba et al., 2018), as well as in plant and livestock research. For example, mango and apple an open-source software and can be downloaded for size measurement (Gongal et al., 2018; Wang et al., 2017) free at https://github.com/lyh24k/3DPhenoFish/tree/ and guava detection and pose estimation (Lin et al., 2019) master. have been solved using 3D computer vision techniques. In Keywords: Fish; Phenomics; Morphology; addition, several techniques have been applied in livestock. For example, Le Cozler et al. (2019) scanned 3D images of an Point cloud; 3D scanning entire cow’s body and estimated six phenotypes: i.e., height, heart girth, chest depth, hip width, backside width, and ischial INTRODUCTION width, which showed high correlation with manual Fish morphology provides valuable data for fishery measurements. Light detection and ranging (LiDAR) and conservation management, genome association research, and cameras with depth information have been used to construct artificial breeding (López-Fanjul & Toro, 2007). In association 3D images and calculate body conformation phenotypes in studies, both genotypes and phenotypes are important data in cows (Pezzuolo et al., 2018), cattle (Batanov et al., 2019; functional gene identification. The emergence and Huang et al., 2018), and horses (Pérez-Ruiz et al., 2020). development of high-throughput genomic sequencing has These studies highlight the feasibility of using 3D information made it easy to obtain the genotypes of millions of fish; to obtain more comprehensive phenotypes for animals; however, phenotyping based on traditional manual however, the application of 3D imaging for morphological measurement is a limiting step in such studies and costly in phenotypes in fish is limited, largely due to the lack of fish- terms of labor and time. More importantly, phenotyping results specific tools to provide comprehensive 2D and 3D phenotype may be subjective as manual measurement requires training data. and experience. The lack of monitoring during and after In the current study, we developed 3DPhenoFish software manual measurement can also lead to potential phenotyping for morphological phenotype extraction using 3D point cloud batch effects and biases (Fernandes et al., 2020). Therefore, information for fish species. Its structured pipeline includes there is great demand for a high-throughput and intelligent background filtering, fish segmentation, key point recognition, technique to automatically extract morphological phenotypes and phenotype extraction. Both 2D and 3D morphological for fish studies. phenotypes represented by body length, surface area, and The advancement of computer vision technology enables volume, can be automatically extracted. In addition, morphological phenotypes to be obtained from two- 3DPhenoFish also allows users to adjust key points and dimensional (2D) images using a monocular camera define new phenotypes in an intuitive visualized manner. We (Hartmann et al., 2011). Imaging techniques have been used endemic Schizothoracinae fish species from Tibet to applied in fish recognition (Spampinato et al., 2010) and validate the 3DPhenoFish phenotyping, which showed high counting (Aliyu et al., 2017; Spampinato et al., 2008) using correlation (>0.94) to manual measurements. This software limited 2D morphological phenotypes (mainly body length) could be used to discriminate samples of different species and (Hao et al., 2015; Shah et al., 2019). For more comprehensive even different populations of the same species. morphological information, IMAFISH_ML (Navarro et al., 2016) uses multiple cameras to capture images from two MATERIALS AND METHODS directions for morphological phenotype measurements. Still, 3DPhenoFish extracts the morphological phenotypes of fish this requires the fish to be frozen and fins to be trimmed, from 3D point cloud data. The following points (Figure 1) causing irreversible damage to the fish. However, several non- describe the main data analysis pipeline steps integrated in invasive methods have been developed for living fish. For 3DPhenoFish: instance, Wang et al. (2019) developed a contour-based 1. Data acquisition: This step uses an industrial 3D scanner method for measuring the length of living fish in water. to obtain OBJ or PCD files as input for 3DPhenoFish. Fernandes et al. (2020) used a monocular camera and 2. Data pre-processing: This step removes background and computer vision technique to measure body area, length, and outliers. height in tilapia. However, these studies were designed for 3. Semantic segmentation: This step deals with the specific fish species using 2D images, and morphological segmentation of the fish head, body, and fins. phenotypes extracted via these tools are still limited. 4. Morphological phenotype extraction: This step recognizes Although 2D features provide valuable data for phenotypes, key points and extracts morphological phenotypes. fish are three-dimensional (3D) in nature and exhibit more 5. Phenotype management: This step involves defining elaborate and complex phenotypes, such as head volume and custom phenotypes and key points, which are then stored in body conformation, which are difficult to capture by 2D the database. imaging processes. These 3D traits provide informative phenotypes to describe conformation features (Zermas et al., Data acquisition 2020). However, 3D phenotypes, such as surface area and The first step in using 3DPhenoFish involves scanning 3D Zoological Research 42(4): 492−501, 2021 493 A B Point cloud acquisition g n Voxel grid filtering Remove plane
Details
-
File Typepdf
-
Upload Time-
-
Content LanguagesEnglish
-
Upload UserAnonymous/Not logged-in
-
File Pages10 Page
-
File Size-