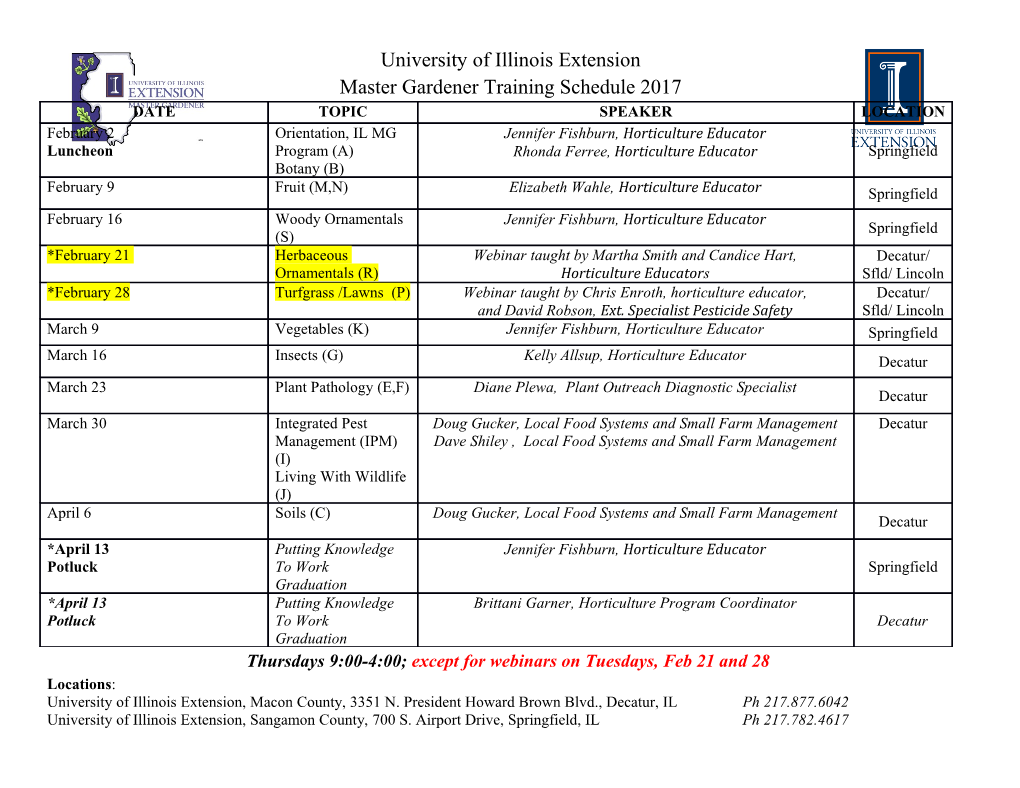
Belief Revision in Dynamic Abducers through Meta-Abduction THESIS Presented in Partial Fulfillment of the Requirements for the Degree Master of Science in the Graduate School of The Ohio State University By Vivek Bharathan Graduate Program in Computer Science and Engineering The Ohio State University 2010 Master's Examination Committee: Dr. John Josephson, Advisor Professor B. Chandrasekaran, Advisor Copyright by Vivek Bharathan 2010 Abstract Abduction machines (or abducers) infer to the best explanation for the data presented to them, and may accumulate beliefs (the conclusions of the inferences) about the world. An abducer‟s beliefs are justified as being the best explanation in contrast with alternative hypotheses. However, the best explanation available to (achievable by) an abducer need not be the true explanation for a variety of reasons including: incomplete search for alternative explanations, insufficient data, and inadequate background knowledge for evaluating alternatives. In light of this fallibility, algorithms were investigated for detecting and correcting errors, for dynamic abducers, by comparing a range of alternative algorithms with regard to effectiveness and computational costs. Dynamic abducers interpret an incoming information stream by producing their best explanations at any point in time. They accumulate new beliefs, and update older beliefs, in the light of new information. In the present work, algorithms were developed for detecting and correcting errors in the accumulated beliefs of such abducers. These algorithms treat the problem of identifying errors as meta-abduction, where certain anomalies that occur during processing are explained as resulting from specific mistakes in previous abductive processing. Errors are then corrected, and beliefs revised, by adopting alternative explanations. A brute-force algorithm for this meta-abduction is computationally intractable, so heuristics were developed with prospects of improving performance. Since a priori mathematical analysis of the algorithms using these heuristics did not reveal ii useful bounds, simulation experiments were conducted, using a specimen domain. The domain was that of multi-object tracking, where an abduction machine, over time, attempts to maintain the track history of mobile entities, based on sensor reports. The experimental results suggest that, using only the heuristics that were investigated, belief revision by meta-abduction enables only small improvements in correctness, and is computationally expensive. iii Vita 1997................................................................Padma Seshadri Bala Bhavan Senior Secondary School 2001................................................................B.E. Computer Science and Engineering, Sri Venkateswara College of Engineering 2002-2003 .....................................................Graduate Teaching Associate, Department of Computer Science and Engineering, The Ohio State University 2003-2008 .....................................................Graduate Research Associate, Department of Computer Science and Engineering, The Ohio State University Publications Vivek Bharathan and John. R. Josephson, "Belief Revision controlled by Meta- Abduction", Special Issue of Logic Journal of IGPL (Oxford University Press), Abduction, Practical Reasoning and Creative Inferences in Science, edited by L. Magnani, 2006 (Volume 14) Vivek Bharathan and John Josephson, “Detecting and Correcting Mistakes in Information iv Fusion”, in proceedings of The Army Science Conference, 2006 Vivek Bharathan and John Josephson, "An Abductive Framework for Level One Information Fusion", in proceedings of The 9th International Conference on Information Fusion, 2006 John Josephson and Vivek Bharathan, "Abductive Inference Engine for Entity Re- identification and Tracking", in proceedings of The Army Science Conference, 2004 Fields of Study Major Field: Computer Science and Engineering Specialization: Artificial Intelligence v Table of Contents Abstract ............................................................................................................................... ii Vita ..................................................................................................................................... iv Table of Contents ............................................................................................................... vi List of Tables ..................................................................................................................... ix List of Figures ..................................................................................................................... x Chapter 1: Introduction ...................................................................................................... 1 1.1 Dynamic Abduction .................................................................................................. 4 Chapter 2: Belief Revision in Dynamic Abducers ............................................................. 9 2.1 Detecting Errors in Dynamic Abducers .................................................................... 9 2.1.2 Trigger 2: Predictions Contradicted by Observations: ..................................... 10 2.1.3 Trigger 3: Clashing Explanations ..................................................................... 11 2.2 Correcting Errors in Dynamic Abducers ................................................................. 11 2.3 Problem Space Complexity for Belief Revision in Abductive Agents ................... 14 Chapter 3: The Abductive Approach to Multi-Object Tracking ....................................... 19 3.1 Description of the ASAS Domain ........................................................................... 19 vi 3.2 The Entity Re-identification Algorithm in Smart-ASAS ........................................ 21 3.3 An Illustrative Example of Abductive Tracking and Error Correction in Smart- ASAS ............................................................................................................................. 24 3.3.1 Initial State ........................................................................................................ 25 3.3.2 Processing Sensor Reports - Generation, Evaluation and Acceptance of Hypotheses................................................................................................................. 26 3.3.3 Revision of previous conclusions in Smart-ASAS ........................................... 28 Chapter 4: The Smart-ASAS Testbed ............................................................................... 33 4.1 The Class of Meta-Abductive Error-Correction Algorithms .................................. 36 4.1.1 The “In Denial” Algorithm ............................................................................... 37 4.1.2 The “Acknowledge Discrepancy” Algorithm ................................................... 38 4.1.3 The “Revision with Recency Heuristic” Algorithm ......................................... 39 4.1.4 The “Revision with Entrenchment Heuristic” Algorithm ................................ 40 4.2 Performance Measures for the Error-Correction Algorithms ................................. 42 Chapter 5: Experimental Results from the Simulated ASAS Domain ............................. 44 5.1 Performance of the Error-Correction Algorithms ................................................... 44 Chapter 6: Summary and Discussion ................................................................................ 69 References ......................................................................................................................... 71 vii Appendix A: An Illustrative Example of Abductive Tracking and Error Correction in Smart-ASAS with Alternative Revisions.......................................................................... 75 Appendix B: The PEIRCE Hypothesis Assembly Algorithm .......................................... 82 viii List of Tables Table 1. Meta-Abductive Belief Revision versus Abductive Processing ......................... 13 Table 2. Parameters in Complexity Analysis of Abductive Belief Revision .................... 16 ix List of Figures Figure 1. Intial State of the World .................................................................................... 25 Figure 2. Decision D and Second Report.......................................................................... 28 Figure 3. Decision D' and Third Report ............................................................................ 30 Figure 4. Final State Estimate ........................................................................................... 32 Figure 5. Performance Measure - Average Track Length ................................................ 46 Figure 6. Performance Measure - Number of Miscorrelations ......................................... 50 Figure 7. Performance Measure – Error Rate ................................................................... 52 Figure 8. Performance Measure – Efficiency .................................................................. 57 Figure 9. Actual Errors versus Observed Errors in different Revision Algorithms .......... 60 Figure 10. Performance of the BR with Recency Algorithm ............................................ 63 Figure 11. Performance of the BR with Entrenchment Algorithm ................................... 65 Figure 12. Initial State of the World ................................................................................
Details
-
File Typepdf
-
Upload Time-
-
Content LanguagesEnglish
-
Upload UserAnonymous/Not logged-in
-
File Pages100 Page
-
File Size-