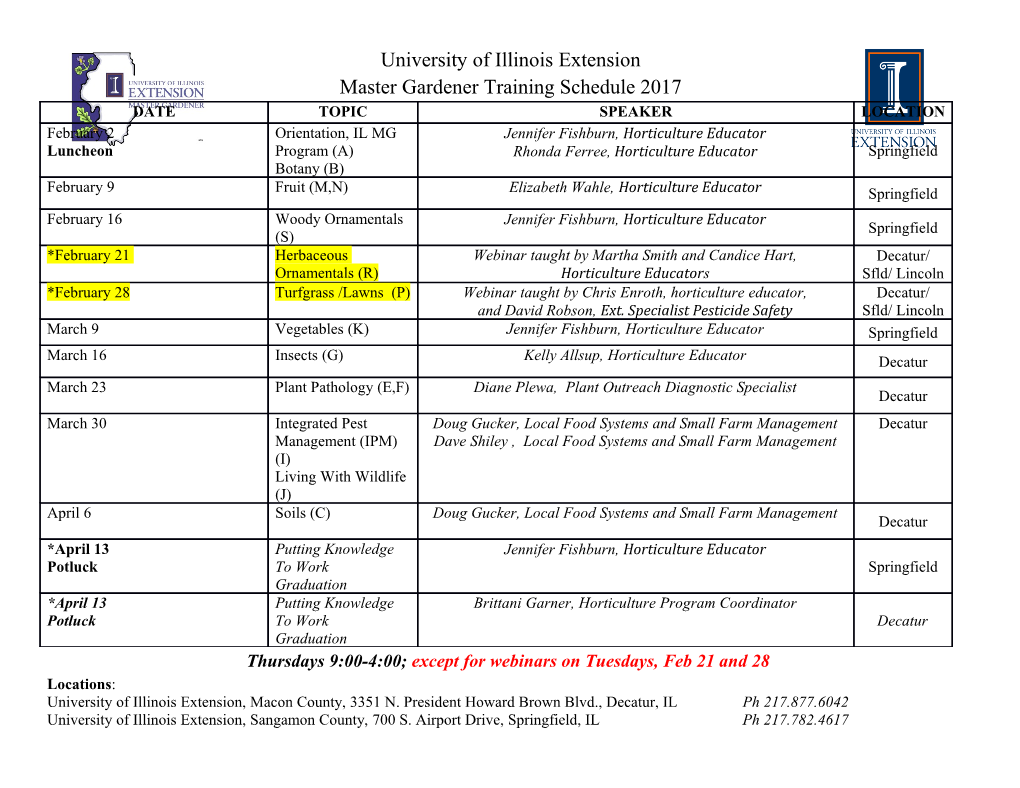
Graph Homomorphism Convolution Hoang NT 1 2 Takanori Maehara 1 Abstract important extension of machine learning as it generalizes In this paper, we study the graph classification learning methods from Euclidean data to non-Euclidean problem from the graph homomorphism perspec- data. This branch of machine learning not only deals with tive. We consider the homomorphisms from F to learning irregular data but also provides a proper means to G, where G is a graph of interest (e.g. molecules combine meta-data with their underlying structure. There- or social networks) and F belongs to some family fore, geometric learning methods have enabled the appli- of graphs (e.g. paths or non-isomorphic trees). cation of machine learning to real-world problems: From We show that graph homomorphism numbers pro- categorizing complex social interactions to generating new vide a natural invariant (isomorphism invariant chemical molecules. Among these methods, graph-learning and F-invariant) embedding maps which can be models for the classification task have been the most impor- used for graph classification. Viewing the ex- tant subject of study. pressive power of a graph classifier by the F- d Let X be the space of features (e.g., X = R for some indistinguishable concept, we prove the universal- positive integer d), Y be the space of outcomes (e.g., Y = ity property of graph homomorphism vectors in f0; 1g), and G = (V (G);E(G)) be a graph with a vertex approximating F-invariant functions. In practice, set V (G) and edge set E(G) ⊆ V (G) × V (G). The graph by choosing F whose elements have bounded tree- classification problem is stated follow1. width, we show that the homomorphism method is efficient compared with other methods. Problem 1 (Graph Classification Problem). We are given a set of tuples f(Gi; xi; yi): i = 1;:::;Ng of graphs Gi = (V (Gi);E(Gi)), vertex features xi : V (Gi) !X , 1. Introduction and outcomes yi 2 Y. The task is to learn a hypothesis h such that h((G ; x )) ≈ y . 2 1.1. Background i i i In many fields of science, objects of interest often exhibit Problem1 has been studied both theoretically and empiri- irregular structures. For example, in biology or chemistry, cally. Theoretical graph classification models often discuss molecules and protein interactions are often modeled as the universality properties of some targeted function class. graphs (Milo et al., 2002; Benson et al., 2016). In multi- While we can identify the function classes which these the- physics numerical analyses, methods such as the finite ele- oretical models can approximate, practical implementations ment methods discretize the sample under study by 2D/3D- pose many challenges. For instance, the tensorized model meshes (Mezentsev, 2004; Fey et al., 2018). In social stud- proposed by (Keriven & Peyre´, 2019) is universal in the ies, interactions between people are presented as a social space of continuous functions on bounded size graphs, but arXiv:2005.01214v2 [cs.LG] 2 Jul 2020 network (Barabasi´ et al., 2016). Understanding these irregu- it is impractical to implement such a model. On the other lar non-Euclidean structures have yielded valuable scientific hand, little is known about the class of functions which can and engineering insights. With recent successful develop- be estimated by some practical state-of-the-art models. To ments of machine learning on regular Euclidean data such address these disadvantages of both theoretical models and as images, a natural extension challenge arises: How do we practical models, we need a practical graph classification learn non-Euclidean data such as graphs or meshes? model whose approximation capability can be parameter- Geometric (deep) learning (Bronstein et al., 2017) is an ized. Such a model is not only effective in practice, as we can introduce inductive bias to the design by the afore- 1RIKEN Center for Advanced Intelligence Project, Tokyo, mentioned parameterization, but also useful in theory as a Japan 2Tokyo Institute of Technology, Tokyo, Japan. Correspon- framework to study the graph classification problem. dence to: Hoang NT <[email protected]>. In machine learning, a model often introduces a set of as- Proceedings of the 37 th International Conference on Machine Learning, Vienna, Austria, PMLR 119, 2020. Copyright 2020 by 1This setting also includes the regression problem. the author(s). 2h can be a machine learning model with a given training set. Graph Homomorphism Convolution sumptions, which is known as inductive bias. These as- In this paper, we focus on simple undirected graphs without sumptions help narrow down the hypothesis space while edge weights for simplicity. The extension of all our results maintaining the validity of the learning model subject to to directed and/or weighted graphs is left as future work. the nature of the data. For example, a natural inductive bias for graph classification problems is the invariant to 1.3. Related Works the permutation property (Maron et al., 2018; Sannai et al., 2019). We are often interested in a hypothesis h that is in- There are two main approaches to construct an embedding: graph kernels and graph neural networks. In the following variant to isomorphism, i.e., for two isomorphic graphs G1 paragraphs, we introduce some of the most popular methods and G2 the hypothesis h should produce the same outcome, which directly related to our work. For a more comprehen- h(G1) = h(G2). Therefore, it is reasonable to restrict our attention to only invariant hypotheses. More specifically, sive view of the literature, we refer to surveys on graph neu- we focus on invariant embedding maps because we can con- ral networks (Wu et al., 2019) and graph kernels (Gartner¨ , struct an invariant hypothesis by combining these mappings 2003; Kriege et al., 2019). with any machine learning model designed for vector data. Consider the following research question: 1.3.1. GRAPH KERNELS Question 2. How to design an efficient and invariant em- The kernel method first defines a kernel function on the bedding map for the graph classification problem? space, which implicitly defines an embedding ρ such that the inner product of the embedding vectors gives a kernel 1.2. Homomorphism Numbers as a Classifier function. Graph kernels implement ρ by counting methods or graph distances (often exchangeable measures). There- A common approach to Problem1 is to design an embed- fore, they are isomorphism-invariant by definition. ding3 ρ:(G; x) 7! ρ((G; x)) 2 Rp, which maps graphs to vectors, where p is the dimensionality of the representation. The graph kernel method is the most popular approach to Such an embedding can be used to represent a hypothesis study graph embedding maps. Since designing a kernel for graphs as h((G; x)) = g(ρ((G; x)) by some hypothesis which uniquely represents graphs up to isomorphisms is as g : Rp !Y for vectors. Because the learning problem on hard as solving graph isomorphism (Gartner¨ et al., 2003), vectors is a well-studied problem, we can focus on designing many previous studies on graph kernels have focused on and understanding graph embedding. proposing a solution to the trade-off between computational efficiency and representability. A natural idea is to compute We found that using homomorphism numbers as an invariant subgraph frequencies (Gartner¨ et al., 2003) to use as graph embedding is not only theoretically valid but also extremely embeddings. However, counting subgraphs is a #W[1]-hard efficient in practice. In a nutshell, the embedding for a problem (Flum & Grohe, 2006) and even counting induced graph G is given by selecting k pattern graphs to form a subgraphs is an NP-hard problem (more precisely it is an fixed set F, then computing the homomorphism numbers #A[1]-hard problem (Flum & Grohe, 2006)). Therefore, from each F 2 F to G. The classification capability of methods like the tree kernel (Collins & Duffy, 2002; Mahe´ the homomorphism embedding is parameterized by F. We & Vert, 2009) or the random walk kernel (Gartner¨ et al., develop rigorous analyses for this idea in Section2 (without 2003; Borgwardt et al., 2005) restrict the subgraph family to vertex features) and Section3 (with vertex features). be some computationally efficient graphs. Regarding graph Our contribution is summarized as follows: homomorphism,G artner¨ et al. and also Mahe´ & Vert studied a relaxation which is similar to homomorphism counting • Introduce and analyze the usage of weighted graph ho- (walks and trees). Especially, Mahe´ & Vert showed that the momorphism numbers with a general choice of F. The tree kernel is efficient for molecule applications. However, choice of F is a novel way to parameterize the capabil- their studies limit to tree kernels and it is not known to what ity of graph learning models compared to choosing the extend these kernels can represent graphs. tensorization order in other related work. • Prove the universality of the homomorphism vector More recently, the graphlet kernel (Shervashidze et al., 2009; in approximating F-indistinguishable functions. Our Przuljˇ et al., 2004) and the Weisfeiler-Lehman kernel (Sher- main proof technique is to check the condition of the vashidze et al., 2011; Kriege et al., 2016) set the state-of-the- Stone-Weierstrass theorem. art for benchmark datasets (Kersting et al., 2016). Other sim- ilar kernels with novel modifications to the distance function, • Empirically demonstrate our theoretical findings with such as Wasserstein distance, are also proposed (Togninalli synthetic and benchmark datasets. Notably, we show et al., 2019). While these kernels are effective for bench- that our methods perform well in graph isomorphism mark datasets, some are known to be not universal (Xu et al., test compared to other machine learning models. 2019; Keriven & Peyre´, 2019) and it is difficult to address 3Not to be confused with “vertex embedding”.
Details
-
File Typepdf
-
Upload Time-
-
Content LanguagesEnglish
-
Upload UserAnonymous/Not logged-in
-
File Pages15 Page
-
File Size-