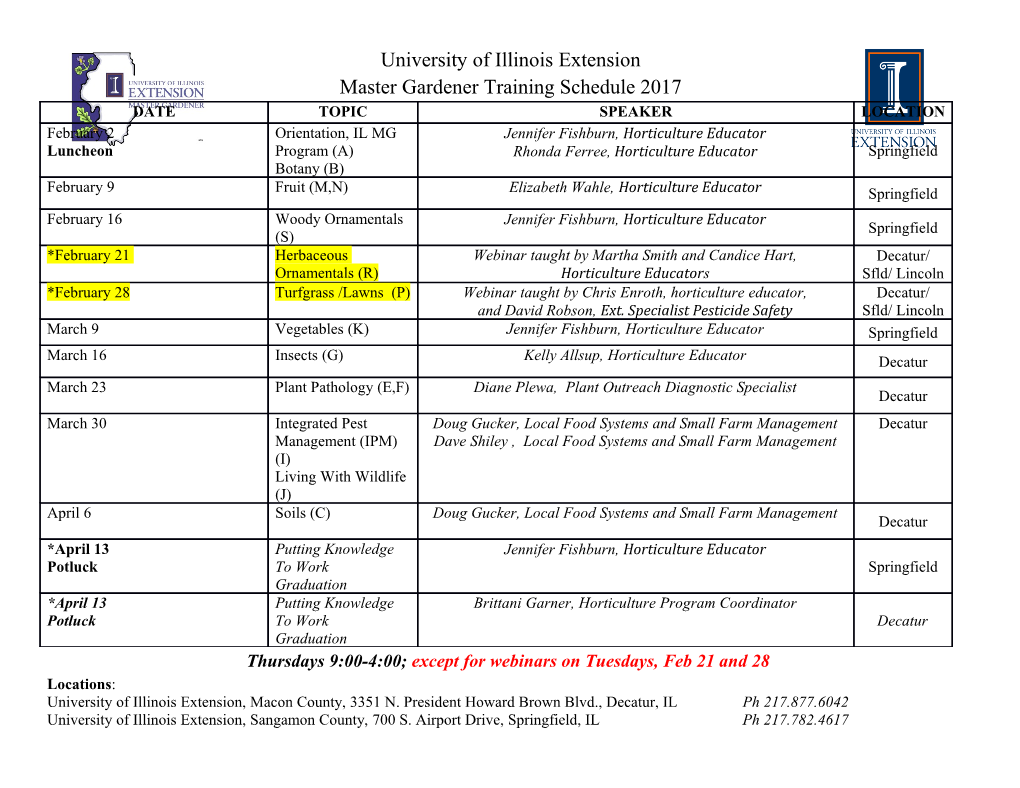
14 10/25/2010 14.1 Vector Spaces Definition 14.2 (See Definition on p. 190). A vector space is a non- empty set V of objects, called vectors, equipped with an addition operation \+" Let us recall the following basic properties about Rn: and scalar (=R or maybe C) multiplication \·" satisfying all of the properties above: i.e. For all u; v; w 2 V and a; b 2 R; Theorem 14.1 (See middle of p. 27). For all x; y; z 2 Rn and a; b 2 R; 1. Associativity of addition: u + (v + w) = (u + v) + w: 1. Associativity of addition: x + (y + z) = (x + y) + z: 2. Commutativity of addition: v + w = w + v: 2. Commutativity of addition: y + z = z + y: 3. Identity element of addition: There is a element 0 2 V such that 3. Identity element of addition: 0 + y = y for all y: 4. Inverse elements of addition: −y + y = 0 for all y 2 Rn: 0 + v = v for all v 2 V: 5. Distributivity of scalar multiplication with respect to vector addi- tion: a(y + z) = ay + az: 4. Inverse elements of addition: −v + v = 0 for all v 2 V: 6. Distributivity of scalar multiplication with respect to field addition (In fact −v = (−1) · v:) (a + b)y = ay + by: 5. Distributivity of scalar multiplication with vector addition: 7. Compatibility of scalar multiplication with the multiplication on R a(by) = (ab)y. a · (v + w) = a · v + a · w: 8. Identity element of scalar multiplication 1y = y for all y 2 Rn: 6. Distributivity of scalar multiplication with respect to field addi- We now turn these properties into a definition. tion (a + b) · v = a · v + b · v: 7. Compatibility of scalar multiplication with the multiplication on R a · (b · v) = (ab) · v: 8. Identity element of scalar multiplication 1 · v = v for all v 2 V: Example 14.3. The infinite blackboard with geometric addition and scalar mul- tiplication. a + b (a + b) + c = a + (b + c) a b b + c c Fig. 14.1. Checking the graphical vector addition is associative. Example 14.4 (The Main Umbrella Example). Let D be a non-empty set and let V = V (D) denote all functions, f : D ! R: For f; g 2 V and λ 2 R we define f + g and λ · f by (f + g)(t) = f (t) + g (t) (addition in R), (λ · f)(t) = λf (t) (multiplication in R): It can now be checked that V is a vector space so that functions have now become vectors! Essentially all other examples of vector spaces we give will be related to an example of this form. Example 14.5. R3 = fx : f1; 2; 3g ! Rg and more generally Rn = fx : f1; 2; : : : ; ng ! Rg : Example 14.6. The vector space of 2 × 2 matrices; M2×2 = fA : A is a 2 × 2 { matrix g = fA : f(1; 1) ; (1; 2) ; (2; 1) ; (2; 2)g ! Rg : This can be generalized. Definition 14.7 (Subspaces are like Las Vegas). Let V be a vector space. A non-empty subset, H ⊂ V; is a subspace of V if H is closed under addition and scalar multiplication. Note, if H is a subspace and v 2 H; then 0 = 0·v 2 H: [What happens in Vegas stays in Vegas and 0 2 Vegas.] Proposition 14.8. Every subspace H of a vector space, V; is a vector space!.
Details
-
File Typepdf
-
Upload Time-
-
Content LanguagesEnglish
-
Upload UserAnonymous/Not logged-in
-
File Pages2 Page
-
File Size-