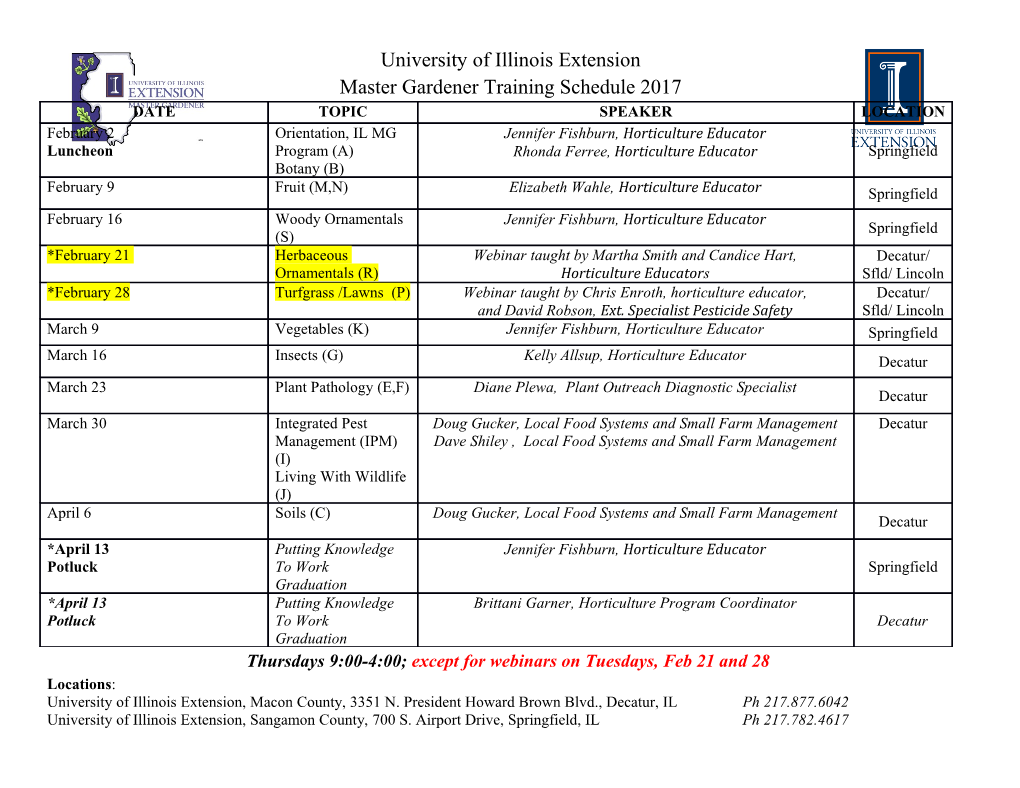
G*Power 3.1 manual March 1, 2017 This manual is not yet complete. We will be adding help on more tests in the future. If you cannot find help for your test in this version of the manual, then please check the G*Power website to see if a more up-to-date version of the manual has been made available. Contents 21 Wilcoxon signed-rank test: Means - difference from constant (one sample case) 50 1 Introduction2 22 Wilcoxon signed-rank test: (matched pairs) 52 2 The G * Power calculator7 23 Wilcoxon-Mann-Whitney test of a difference be- 3 Exact: Correlation - Difference from constant (one tween two independent means 56 sample case)9 24 t test: Generic case 60 4 Exact: Proportion - difference from constant (one sample case) 11 25 c2 test: Variance - difference from constant (one sample case) 61 5 Exact: Proportion - inequality, two dependent groups (McNemar) 14 26 z test: Correlation - inequality of two independent Pearson r’s 62 6 Exact: Proportions - inequality of two independent groups (Fisher’s exact-test) 17 27 z test: Correlation - inequality of two dependent Pearson r’s 63 7 Exact test: Multiple Regression - random model 18 28 Z test: Multiple Logistic Regression 67 8 Exact: Proportion - sign test 22 29 Z test: Poisson Regression 72 9 Exact: Generic binomial test 23 30 Z test: Tetrachoric Correlation 77 10 F test: Fixed effects ANOVA - one way 24 References 81 11 F test: Fixed effects ANOVA - special, main effects and interactions 26 12 t test: Linear Regression (size of slope, one group) 31 13 F test: Multiple Regression - omnibus (deviation of R2 from zero), fixed model 33 14 F test: Multiple Regression - special (increase of R2), fixed model 36 15 F test: Inequality of two Variances 39 16 t test: Correlation - point biserial model 40 17 t test: Linear Regression (two groups) 42 18 t test: Means - difference between two dependent means (matched pairs) 45 19 t test: Means - difference from constant (one sam- ple case) 47 20 t test: Means - difference between two independent means (two groups) 49 1 1 Introduction Distribution-based approach to test selection First select the family of the test statistic (i.e., exact, F−, t−, c2, or z- G*Power (Fig.1 shows the main window of the program) test) using the Test family menu in the main window. The covers statistical power analyses for many different statisti- Statistical test menu adapts accordingly, showing a list of all cal tests of the tests available for the test family. • F test, Example: For the two groups t-test, first select the test family • t test, based on the t distribution. • c2-test and • z test families and some • exact tests. G*Power provides effect size calculators and graphics options. G * Power supports both a distribution-based and a design-based input mode. It contains also a calculator that supports many central and noncentral probability distribu- tions. G*Power is free software and available for Mac OS X and Windows XP/Vista/7/8. Then select Means: Difference between two independent means 1.1 Types of analysis (two groups) option in the Statictical test menu. G*Power offers five different types of statistical power analysis: 1. A priori (sample size N is computed as a function of power level 1 − b, significance level a, and the to-be- detected population effect size) 2. Compromise (both a and 1 − b are computed as func- tions of effect size, N, and an error probability ratio q = b/a) 3. Criterion (a and the associated decision criterion are computed as a function of 1 − b, the effect size, and N) Design-based approach to the test selection Alterna- tively, one might use the design-based approach. With the 4. Post-hoc (1 − b is computed as a function of a, the pop- Tests pull-down menu in the top row it is possible to select ulation effect size, and N) • the parameter class the statistical test refers to (i.e., 5. Sensitivity (population effect size is computed as a correlations and regression coefficients, means, propor- − function of a, 1 b, and N) tions, or variances), and 1.2 Program handling • the design of the study (e.g., number of groups, inde- pendent vs. dependent samples, etc.). Perform a Power Analysis Using G * Power typically in- volves the following three steps: The design-based approach has the advantage that test op- tions referring to the same parameter class (e.g., means) are 1. Select the statistical test appropriate for your problem. located in close proximity, whereas they may be scattered across different distribution families in the distribution- 2. Choose one of the five types of power analysis available based approach. 3. Provide the input parameters required for the analysis and click "Calculate". Example: In the Tests menu, select Means, then select Two inde- pendent groups" to specify the two-groups t test. Plot parameters In order to help you explore the param- eter space relevant to your power analysis, one parameter (a, power (1 − b), effect size, or sample size) can be plotted as a function of another parameter. 1.2.1 Select the statistical test appropriate for your prob- lem In Step 1, the statistical test is chosen using the distribution- based or the design-based approach. 2 Figure 1: The main window of G * Power 1.2.2 Choose one of the five types of power analysis • the required power level (1 − b), available • the pre-specified significance level a, and In Step 2, the Type of power analysis menu in the center of the main window is used to choose the appropriate analysis • the population effect size to be detected with probabil- type and the input and output parameters in the window ity (1 − b). change accordingly. In a criterion power analysis, a (and the associated deci- Example: If you choose the first item from the Type of power sion criterion) is computed as a function of analysis menu the main window will display input and output • 1-b, parameters appropriate for an a priori power analysis (for t tests for independent groups if you followed the example provided • the effect size, and in Step 1). • a given sample size. In a compromise power analysis both a and 1 − b are computed as functions of • the effect size, • N, and • an error probability ratio q = b/a. In an a priori power analysis, sample size N is computed In a post-hoc power analysis the power (1 − b) is com- as a function of puted as a function of 3 • a, Because Cohen’s book on power analysis Cohen(1988) appears to be well known in the social and behavioral sci- • the population effect size parameter, and ences, we made use of his effect size measures whenever • the sample size(s) used in a study. possible. In addition, wherever available G * Power pro- vides his definitions of "‘small"’, "‘medium"’, and "‘large"’ In a sensitivity power analysis the critical population ef- effects as "‘Tool tips"’. The tool tips may be optained by fect size is computed as a function of moving the cursor over the "‘effect size"’ input parameter field (see below). However, note that these conventions may • a, have different meanings for different tests. •1 − b, and Example: The tooltip showing Cohen’s measures for the effect •N. size d used in the two groups t test 1.2.3 Provide the input parameters required for the anal- ysis In Step 3, you specify the power analysis input parameters in the lower left of the main window. Example: An a priori power analysis for a two groups t test would require a decision between a one-tailed and a two-tailed test, a specification of Cohen’s (1988) effect size measure d un- ( − ) der H1, the significance level a, the required power 1 b of If you are not familiar with Cohen’s measures, if you the test, and the preferred group size allocation ratio n /n . 2 1 think they are inadequate for your test problem, or if you Let us specify input parameters for have more detailed information about the size of the to-be- • a one-tailed t test, expected effect (e.g., the results of similar prior studies), • a medium effect size of d = .5, then you may want to compute Cohen’s measures from • a = .05, more basic parameters. In this case, click on the Determine button to the left the effect size input field. A drawer will • (1 − b) = .95, and open next to the main window and provide access to an • an allocation ratio of n2/n1 = 1 effect size calculator tailored to the selected test. Example: For the two-group t-test users can, for instance, spec- ify the means m1, m2 and the common standard deviation (s = s1 = s2) in the populations underlying the groups to cal- culate Cohen’s d = jm1 − m2j/s. Clicking the Calculate and transfer to main window button copies the computed effect size to the appropriate field in the main window This would result in a total sample size of N = 176 (i.e., 88 observation units in each group). The noncentrality parameter d defining the t distribution under H1, the decision criterion to be used (i.e., the critical value of the t statistic), the degrees of freedom of the t test and the actual power value are also displayed. In addition to the numerical output, G * Power displays Note that the actual power will often be slightly larger the central (H0) and the noncentral (H1) test statistic distri- than the pre-specified power in a priori power analyses.
Details
-
File Typepdf
-
Upload Time-
-
Content LanguagesEnglish
-
Upload UserAnonymous/Not logged-in
-
File Pages82 Page
-
File Size-