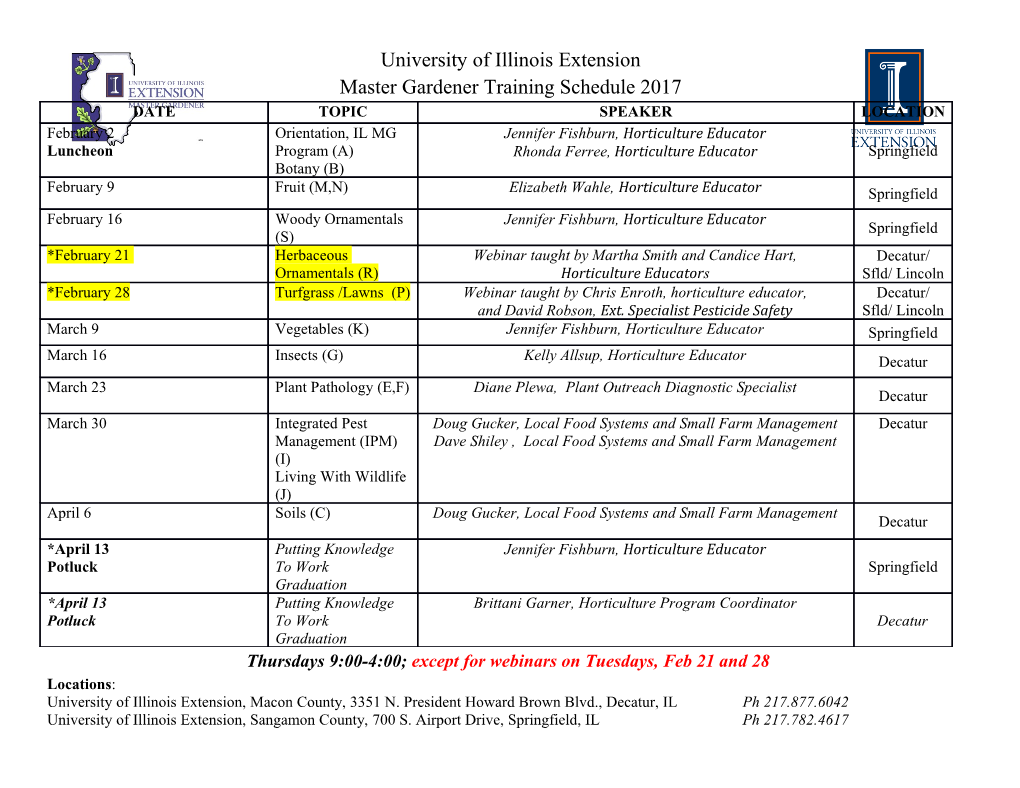
A Indexing Methods for Approximate Dictionary Searching: Comparative Analysis Leonid Boytsov The primary goal of this paper is to survey state of the art indexing methods for approximate dictionary searching. To improve understanding of the field, we introduce a taxonomy that classifies all methods into direct methods and sequence-based filtering methods. We focus on infrequently updated dictionaries, which are used primarily for retrieval. Therefore, we consider indices that are optimized for retrieval rather than for update. The indices are assumed to be associative, i.e., capable of storing and retrieving auxiliary information, such as string identifiers. All solutions are lossless and guarantee retrieval of strings within a specified edit distance k. Benchmark results are presented for the practically important cases of k = 1, 2, 3. We concentrate on natural language datasets, which include synthetic English and Russian dictionaries, as well as dictionaries of frequent words extracted from the ClueWeb09 collection. In addition, we carry out experiments with dictionaries containing DNA sequences. The paper is concluded with a discussion of benchmark results and directions for future research. Categories and Subject Descriptors: F.2.2 [Analysis of algorithms and problem complexity]: Non- numerical algorithms and problems—Pattern matching; H.3.3 [Information storage and retrieval]: In- formation search and retrieval General Terms: Algorithms, Experimentation, Performance Additional Key Words and Phrases: approximate searching, Levenshtein distance, Damerau-Levenshtein distance, frequency distance, neighborhood generation, q-gram, q-sample, metric trees, trie, k-errata tree, frequency vector trie, agrep, NR-grep. ACM Reference Format: ACM J. Exp. Algor. 0, 0, Article A ( 00), 92 pages. DOI = 10.1145/0000000.0000000 http://doi.acm.org/10.1145/0000000.0000000 As computers are used in an ever-widening variety of lexical processing tasks, the problem of error detection and correction becomes more critical. Mere volume, if nothing else, will prevent the employment of manual detection and correction procedures. Damerau [1964] 1. INTRODUCTION Detection and correction of errors is a challenging task, which gave rise to a vari- ety of approximate search algorithms. At present, these algorithms are used in spell- checkers [Zamora et al. 1981; Kukich 1992; Brill and Moore 2000; Wilbur et al. 2006], Author’s address: 11710 Old Georgetown Rd, Unit 612, North Bethesda, MD, 20852, USA; e-mail: [email protected]. Permission to make digital or hard copies of part or all of this work for personal or classroom use is granted without fee provided that copies are not made or distributed for profit or commercial advantage and that copies show this notice on the first page or initial screen of a display along with the full citation. Copyrights for components of this work owned by others than ACM must be honored. Abstracting with credit is per- mitted. To copy otherwise, to republish, to post on servers, to redistribute to lists, or to use any component of this work in other works requires prior specific permission and/or a fee. Permissions may be requested from Publications Dept., ACM, Inc., 2 Penn Plaza, Suite 701, New York, NY 10121-0701 USA, fax +1 (212) 869-0481, or [email protected]. c 00 ACM 1084-6654/00/-ARTA $10.00 DOI 10.1145/0000000.0000000 http://doi.acm.org/10.1145/0000000.0000000 ACM Journal of Experimental Algorithmics, Vol. 0, No. 0, Article A, Publication date: 00. A:2 Leonid Boytsov computer-aided translation systems (e.g., Trados1), optical character recognition sys- tems [Ford et al. 2001], spoken-text recognition and retrieval systems [Vintsyuk 1968; Velichko and Zagoruyko 1970; Ng and Zue 2000; Glass 2003], computational biology [Gusfield 1999; Ozturk and Ferhatosmanoglu 2003; Kahveci et al. 2004], phonology [Covington 1996; Kondrak 2003], and for phonetic matching [Zobel and Dart 1996]. Approximate dictionary searching is important to computer security, where it can be used to identify “weak” passwords susceptible to a dictionary attack [Manber and Wu 1994a]. Another important application is a detection of similar domain names that are registered mostly for typo-squatting [Moore and Edelman 2010]. This is also fre- quently required in disputes over almost identical domain names, particularly when brand names are involved.2 Along with other sources of corrupt data, such as recognition of spoken and printed text, a lot of errors are attributable to the human factor, i.e., cognitive or mechanical errors. In particular, English orthography is notorious for its inconsistency and, there- fore, poses significant challenges. For instance, one of the most commonly misspelled words is “definitely”. Though apparently simple, it is often written as “definatly” [Collins 2009]. An example of a frequent mechanical error is reversal of adjacent characters, known as a transposition. Transpositions account for 2-13 percent of all misspelling errors [Peterson 1986]. Therefore, it is important to consider transposition-aware methods. Search methods can be classified into on-line and off-line methods. On-line search methods include algorithms for finding approximate pattern occurrences in a text that cannot be preprocessed and indexed. A well-known on-line search algorithm is based on the dynamic programming approach [Sellers 1974]. It is O (n m) in time and O(n) in space, where n and m are the lengths of a pattern and a text,· respectively. The on- line search problem was extensively studied and a number of algorithms improving the classic solution were suggested, including simulation of non-deterministic finite automata (NFA) [Wu and Manber 1992b; Navarro and Raffinot 2000; Navarro 2001b], simulation of deterministic finite automata (DFA) [Ukkonen 1985b; Melichar 1996; Kurtz 1996; Navarro 1997b], and bit-parallel computation of the dynamic program- ming matrix [Myers 1999; Hyyro¨ 2005]. However, the running time of all on-line search methods is proportional to the text size. Given the volume of textual data, this approach is inefficient. The necessity of much faster retrieval tools motivated development of methods that rely on text pre- processing to create a search index. These search methods are known as off-line or indexing search methods. There are two types of indexing search methods: sequence-oriented methods and word-oriented methods. Sequence-oriented methods find arbitrary substrings within a specified edit distance. Word-oriented methods operate on a text that consists of strings divided by separators. Unlike sequence-oriented methods, they are designed to find only complete strings (within a specified edit distance). As a result, a word- oriented index would fail to find the misspelled string “wiki pedia” using “wikipedia” as the search pattern (if one error is allowed). In the case of natural languages – on which we focus in this survey – this is a reasonable restriction. The core element of a word-oriented search method is a dictionary. The dictionary is a collection of distinct searchable strings (or string sequences) extracted from the text. Dictionary strings are usually indexed for faster access. A typical dictionary index allows for exact search and, occasionally, for prefix search. In this survey, we review 1http://trados.com 2A 2006 dispute on domains telefonica.cl´ and telefonica.cl resulted in court canceling the “accented” domain version, because it was too similar to the original one [Zaliznyak 2006]. ACM Journal of Experimental Algorithmics, Vol. 0, No. 0, Article A, Publication date: 00. Indexing Methods for Approximate Dictionary Searching: Comparative Analysis A:3 associative methods that allow for an approximate dictionary search. Given the search pattern p and a maximum allowed edit distance k, these methods retrieve all dictionary strings s (as well as associated data) such that the distance between p and s is less than or equal to k. One approach to sequence-oriented searching also relies on dictionary matching. During indexing, all unique text substrings with lengths in a given interval (e.g., from 5 to 10) are identified. For each unique substring s, the indexing algorithm compiles the list of positions where s occurs in the text. Finally, all unique substrings are combined into a dictionary, which is used to retrieve occurrence lists efficiently. Although this method is not space efficient, it can be used for retrieval of DNA sequences. The problem of index-based approximate string searching, though not as well stud- ied as on-line string searching, attracted substantial attention from practitioners and scientists. There are many experimental, open source, and commercial word-oriented indexing applications that support approximate searching: Glimpse [Manber and Wu 1994b], dtSearch3, to name but a few. Other examples of software that employ approx- imate dictionary searching are spell-checkers Ispell [Kuenning et al. 1988] and GNU Aspell,4 as well as spoken-text recognition systems: SUMMIT [Zue et al. 1989; Glass 2003],5 Sphinx [Siegler et al. 1997],6 and HTK [Woodland et al. 1994].7. Commonly used indexing approaches to approximate dictionary searching include the following: — Full and partial neighborhood generation [Gorin 1971; Mor and Fraenkel 1982; Du and Chang 1994; Myers 1994; Russo and Oliveira 2005]; — q-gram indices [Angell et al. 1983; Owolabi and McGregor 1988; Jokinen and Ukko- nen 1991; Zobel et al. 1993; Gravano et al. 2001; Carterette and Can 2005; Navarro et al. 2005]; — Prefix trees (tries) [James and Partridge 1973; Klovstad and Mondshein 1975; Baeza- Yates and Gonnet 1990; Ukkonen 1993; Cole et al. 2004; Mihov and Schulz 2004]; — Metric space methods [Baeza-Yates and Navarro 1998; Navarro et al. 2002; Fredriks- son 2007; Figueroa and Fredriksson 2007]. There exist several good surveys on this topic [Hall and Dowling 1980; Peterson 1980; Kukich 1992; Owolabi 1996; Navarro 2001a; Navarro et al. 2001; Sung 2008], yet, none addresses the specifics of dictionary searching in sufficient detail. We aim to fill this gap by presenting a taxonomy of state of the art indexing methods for approx- imate dictionary searching and experimental comparison results.
Details
-
File Typepdf
-
Upload Time-
-
Content LanguagesEnglish
-
Upload UserAnonymous/Not logged-in
-
File Pages93 Page
-
File Size-