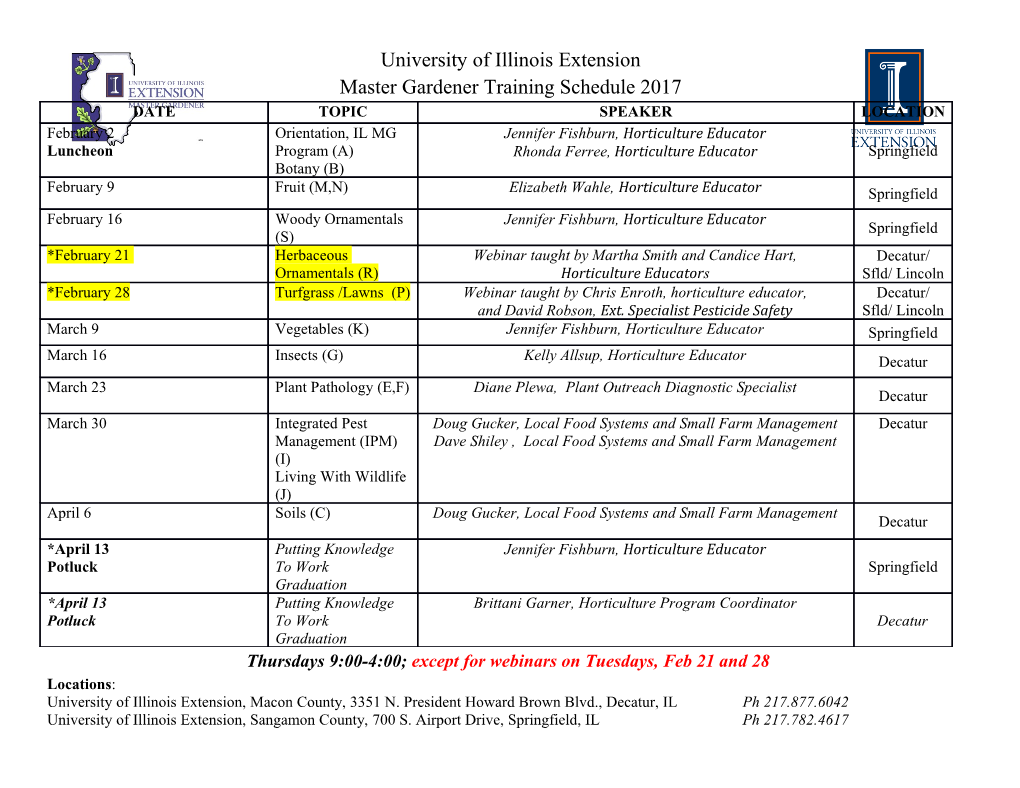
GEOProcessing 2014 : The Sixth International Conference on Advanced Geographic Information Systems, Applications, and Services Spatio Temporal Density Mapping of a Dynamic Phenomenon Stefan Peters, Liqiu Meng Department of Cartography Technische Universität München München, Germany e-mail: [email protected], [email protected] Abstract— Visualizing density and distribution information is a can depend on point’s attribute value. In order to discern the key support for understanding spatio-temporal phenomena density distribution, these graphic variables can be iteratively represented by point data. However, the temporal information adapted to the given map scale, but still the occlusion of is not yet adequately handled in existing density map neighboring points cannot be always desirably avoided. The approaches. In this paper, we propose a novel approach for the density value of each point can be obtained by counting all creation of a 2D density surface - using contour intervals – for points within a buffer around the point or within a grid cell dynamic points or phenomena. Our method is based on a the point is located in. rainbow color scheme, which enables the user to visually In the following, the density estimation and map extract spatio-temporal changes of point density and principles are shortly presented and the state of the art of distribution. Furthermore, we present various possibilities for density maps with static or dynamic data is given. extending and improving our approach. A. Kernel Density Estimation (KDE) Keywords-spatio temporal density map; rainbow color scheme; visual analysis; dynamic data. The Kernel Density Estimation (KDE) [5] is a classic method widely used to determine densities of individual points that represent a continuous surface. The KDE I. INTRODUCTION approach is described in detail in [5, 6, 7]. The standard Visualization helps to investigate and understand KDE, a normal distribution function, uses a Gaussian kernel. complex relationships in a spatial context. Maps account as A certain bandwidth (search radius) is defined for the one of the most powerful visualization forms. They represent kernels, located around each point. For each cell of an geographic information in abstract ways that support the underlying grid (defined by a certain resolution) a density identification of spatial patterns and the interpretation of value is calculated and hence a smooth surface is provided spatial phenomena. [8]. The bandwidth value strongly influences the density Furthermore, the visual presentation and analysis of surface [9]. A formula for an optimal bandwidth is proposed dynamic data and dynamic phenomena is currently a hot by Silverman [6]. Kernel density estimates have been used research topic [1]. for cluster detection in various fields, such as crime analysis. Hence, in today’s society, the need for data abstraction Kwan [10] uses geovisualization of activity patterns in along with the growing amount of available digital geodata is space–time and displays the results as a continuous density rapidly increasing. One reasonable way of abstracting data is surface. She applies the density estimation as a method of provided by density maps [2]. geovisualization to find patterns in human activities related Density maps can be applied for point data in various to other social attributes. Assent, Krieger, Müller, and Seidl fields, for instance, in physical or human geography, [11], Krisp and Špatenková [12], and Maciejewski, et al. [13] geology, medicine, economy or biology [3, 4]. How to investigate the classic kernel density estimation and define it present the density for dynamic data/phenomena is, however, as a visual clustering method. In these works, KDE maps not yet adequately addressed. were created in order to visually provide a better overview In this paper, we introduce a novel density mapping and insight into the given data. approach for spatially and temporally changing data. B. Contour lines and intervals In the next section, the state of the art related to density maps, in particular, an overview of approaches considering A common technique to map point densities calculated the dynamics of movement data in the density visualization using KDE are isopleth maps with filled contour intervals. is given. In the section afterwards, our own approach is The term “isopleth map” (isopleth = equal in quantity and described in detail, followed by implementation processes, number), refers to one of two types of isoline maps (also discussions of the results and a conclusion. called isarithmic or contour maps). In the first type of isoline maps each contour line indicates a constant rate or ratio II. DENSITY MAPS - STATE OF THE ART derived from the values of a buffer zone or kernel area. In this sense, the continuous density surface is derived from an One of the most straight forward ways to visualize point originally discrete surface. In the other type of isoline maps density is a scatter plot or a dot map. Graphic variables for (commonly referred to “isometric map”), contour lines point symbols, such as size, shape, color and transparency, (isometers) are drawn through points with directly Copyright (c) IARIA, 2014. ISBN: 978-1-61208-326-1 83 GEOProcessing 2014 : The Sixth International Conference on Advanced Geographic Information Systems, Applications, and Services measurable equal value or intensity such as terrain height or Nakaya and Yano [27] suggest a method using a space– temperature [14]. It is assumed that the data collected for time cube to visually explore the spatio-temporal density enumeration units are part of a smooth, inherently distribution of crime data in an interactive 3D GIS. In order continuous phenomenon [15]. In this paper, we only use to investigate the dynamics and density change, an contour lines to delimit the intervals (the areas between interactive use within a 3D environment is essential. contour lines). Furthermore, Langford provides a good 5) KDE for trajectories overview of density surfaces used in Geographic Information In a comprehensive review of the existing visual analysis Systems (GIS) as choropleth population density maps, [1], methods, tools and concepts of discrete objects point population density on grids, population density surfaces, and data were introduced. A section is dedicated to continuous pseudo-3D population density surfaces [16]. In several density surfaces (fields) derived from trajectories or from works as [3, 4], the KDE concept is adapted for the 3D- point-related attributes. Density maps of moving objects Space density mapping of static 3D data. were created on the basis of aggregated points of trajectories. A trajectory is understood as a function of time or a path left C. Dynamic data and density information by a moving object in space. Moving objects can be confined In the last years, we published several papers related to within a network (such as cars along streets of a traffic visual analysis of moving objects with a focus on the network) or float freely over a region (boats) or in space representation of dynamic lightning data [17, 18, 19]. In (airplanes). Spatio-temporal density maps of trajectories these works, different visualization methods were proposed were investigated in [28, 29, 30, 31]. In these approaches, the for moving lightning point data. However, density surfaces KDE method is adapted to trajectories as a function of were not yet taken into account. moving velocity and direction. The resulting density maps In the following sub-sections, an overview is given about can reveal simultaneously large-scope patterns and fine existing works related to density maps of dynamic points. features of the trajectories. This mapping idea was extended 1) KDE for dynamic points to the 3D space in [29] where the trajectory densities are A straightforward way of visualizing the density of visualized inside a space-time cube. dynamic points would be a sequence of density surfaces (one Another possibility of displaying density information of per time interval) as reported in [20]. The change of the trajectories is to use derived discrete grid cells, whereby each density in time could be better discernable by means of an cell color refers to the amount of trajectories passing through animation of these density maps. Another option would be to the cell [32, 33]. use an individual Kernel density map with a unique color scheme that fills the areas between the contour lines. Due to D. Research questions the movement of points (as for example swarms), however, In the existing 2D density maps based on KDE, the time the tinted intervals may spatially overlap and make the map is either frozen on a certain moment or confined within a reading a difficult endeavor. certain time interval. Consequently, the resulting contour 2) Dual KDE lines do not carry information of temporal changes. Although Jansenberger and Staufer-Steinocher [21] analyzed two various approaches for density visualization of trajectories different point data sets recorded within the same area, but at have been investigated, an appropriate method for 2D two different moments of time. The authors suggest a Dual- density maps of moving point clouds is still missing. Can the KDE approach, which results in a map illustrating the spatio- dynamics of spatially extended phenomena - represented by temporal density difference of the two datasets. The absolute points - be adequately expressed in a single contour map? difference is used, that is, the absolute density of the second This research question remains unsolved. In the following point data set subtracted from that of the first point data set. sections, we will tackle this question and introduce a new 3) DKDE approach termed Spatio-Temporal Density Mapping or The approach called Directed Kernel Density Estimation STDmapping. (DKDE) that is able to take the dynamics of moving points inside density maps into account was suggested in previous III. METHODOLOGY works [22, 23, 24, 25, 26]. The DKDE is applicable for A. Test data discrete moving points and it considers two moments of time.
Details
-
File Typepdf
-
Upload Time-
-
Content LanguagesEnglish
-
Upload UserAnonymous/Not logged-in
-
File Pages6 Page
-
File Size-