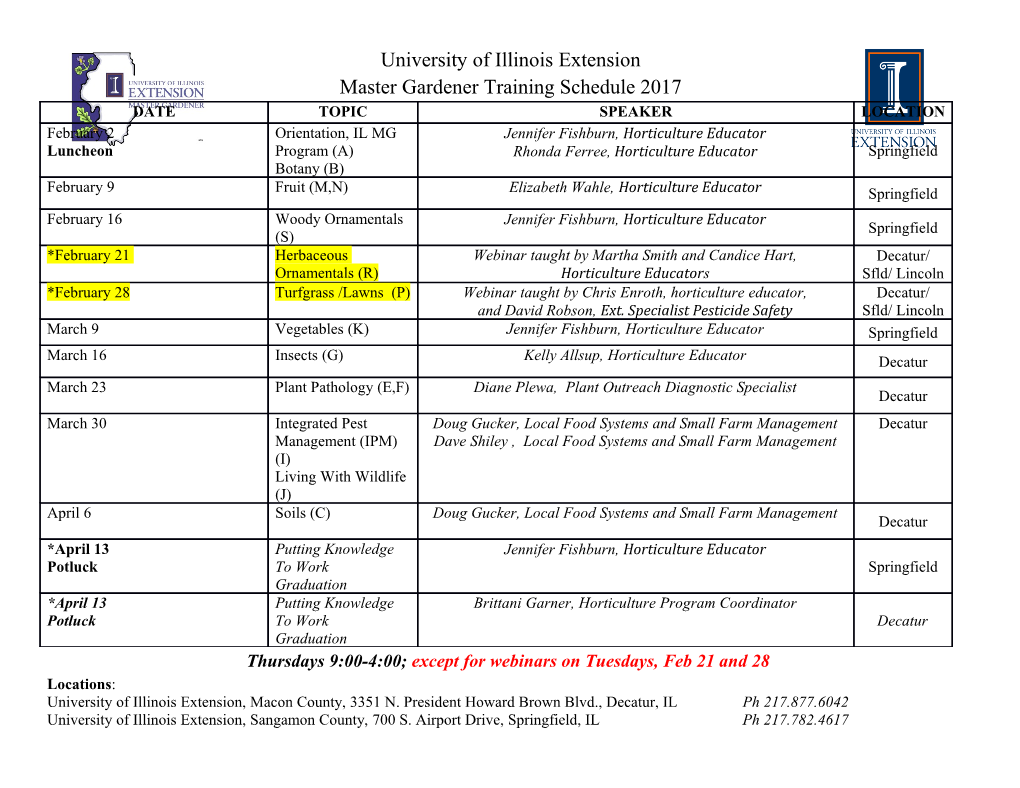
Chapter 5. Least Squares Estimation - Large-Sample Properties In Chapter 3, we assume u x N(0; 2) and study the conditional distribution of given j X. In general the distribution of u x is unknown and even if it is known, the unconditional j 1 b n distribution of is hard to derive since = (X X) X y is a complicated function of xi . 0 0 f gi=1 Asymptotic (or large sample) methods approximate sampling distributions based on the limiting experiment thatb the sample size n tendsb to in…nity. A preliminary step in this approach is the demonstration that estimators converge in probability to the true parameters as the sample size gets large. The second step is to study the distributional properties of in the neighborhood of the true value, that is, the asymptotic normality of . The …nal step is to estimate the asymptotic variance which is necessary in statistical inferences such as hypothesis testingb and con…dence interval (CI) construction. In hypothesis testing, it is necessaryb to construct test statistics and derive their asymptotic distributions under the null. We will study the t-test and three asymptotically equivalent tests under both homoskedasticity and heteroskedasticity. It is also standard to develop the local power function for illustrating the power properties of the test. This chapter concentrates on asymptotic properties related to the LSE. Related materials can be found in Chapter 2 of Hayashi (2000), Chapter 4 of Cameron and Trivedi (2005), Chapter 4 of Hansen (2007), and Chapter 4 of Wooldridge (2010). 1 Asymptotics for the LSE We …rst show that the LSE is CAN and then re-derive its asymptotic distribution by treating it as a MoM estimator. 1.1 Consistency It is useful to express as 1 1 1 =b (X0X) X0y = (X0X) X0 (X + u) = +(X0X) X0u: (1) To show is consistent,b we impose the following additional assumptions. Assumptionb OLS.10: rank(E[xx0]) = k. Assumption OLS.20: y = x0 + u with E[xu] = 0. Email: [email protected] 1 Note that Assumption OLS.1 implicitly assumes that E x 2 < . Assumption OLS.1 is the 0 k k 1 0 large-sample counterpart of Assumption OLS.1, and Assumptionh i OLS.20 is weaker than Assumption OLS.2. p Theorem 1 Under Assumptions OLS.0, OLS.1 , OLS.2 and OLS.3, . 0 0 ! p p Proof. From (1), to show , we need only to show that (X X) b1X u 0. Note that ! 0 0 ! 1 b 1 n 1 n (X X) 1X u = x x x u 0 0 n i i0 n i i i=1 ! i=1 ! Xn n X 1 1 p 1 = g xix0 ; xiui E[xix0 ] E[xiui] = 0: n i n ! i i=1 i=1 ! X X Here, the convergence in probability is from (I) the WLLN which implies n n 1 p 1 p xix0 E[xix0 ] and xiui E[xiui]; (2) n i ! i n ! i=1 i=1 X X 1 (II) the fact that g(A; b) = A b is a continuous function at (E[xixi0 ];E[xiui]). The last equality is from Assumption OLS.20. (I) To apply the WLLN, we require (i) xixi0 and xiui are i.i.d., which is implied by Assumption OLS.0 and that functions of i.i.d. data are also i.i.d.; (ii) E x 2 < (OLS.1 ) and E[ xu ] < . k k 1 0 k k 1 E[ xu ] < is implied by the Cauchy-Schwarz inequality,h1 i k k 1 1=2 1=2 E[ xu ] E x 2 E u 2 ; k k k k j j h i h i 1 which is …nite by Assumption OLS.10 and OLS.3. (II) To guarantee A b to be a continuous 1 function at (E[xixi0 ];E[xiui]), we must assume that E[xixi0 ] exists which is implied by Assumption 2 OLS.10. Exercise 1 Take the model yi = x10 i 1 +x20 i 2 +ui with E[xiui] = 0. Suppose that 1 is estimated by regressing yi on x1i only. Find the probability limit of this estimator. In general, is it consistent for 1? If not, under what conditions is this estimator consistent for 1? We can similarly show that the estimators 2 and s2 are consistent for 2. 2 p p Theorem 2 Under the assumptions of Theoremb 1, 2 and s2 2. ! ! 1 2 1=2 2 1=2 Cauchy-Schwarz inequality: For any random m n matrices X and Y, E [ X0Y ] E X E Y , k k k k k k where the inner product is de…ned as X; Y = E [ X0Y ]. b 2 1 2 1 h i k k2 2 2 If xi R, E[xixi0 ] = E[xi ] is the reciprocal of E[xi ] which is a continuous function of E[xi ] only if E[xi ] = 0. 2 6 2 Proof. Note that ui = yi x0 i = ui + xi0 xi0 b b = ui x0 : i b Thus b 2 2 0 u = u 2uix0 + xix0 (3) i i i i and b b b b 1 n 2 = u2 n i i=1 Xn n n b 1 b2 1 0 1 = ui 2 uixi0 + xixi0 n n ! n ! i=1 i=1 i=1 pX X X 2; b b b ! where the last line uses the WLLN, (2), Theorem 1 and the CMT. Finally, since n=(n k) 1, it follows that ! n p s2 = 2 2 n k ! by the CMT. b One implication of this theorem is that multiple estimators can be consistent for the population parameter. While 2 and s2 are unequal in any given application, they are close in value when n is very large. b 1.2 Asymptotic Normality To study the asymptotic normality of , we impose the following additional assumption. Assumption OLS.5: E[u4] < and E x 4 < . 1 b k k 1 h i Theorem 3 Under Assumptions OLS.0, OLS.10, OLS.20, OLS.3 and OLS.5, pn d N(0; V); ! 1 1 b 2 where V = Q Q with Q = E [xixi0 ] and = E xixi0 ui . Proof. From (1), 1 1 n 1 n pn = xix0 xiui : n i pn i=1 ! i=1 ! X X b 3 Note …rst that 1=2 1=2 2 2 4 1=2 4 4 1=2 E xix0 u E xix0 E u E xi E u < ; (4) i i i i k k i 1 h i h i where the …rst inequality is from the Cauchy-Schwarz inequality, the second inequality is from the Schwarz matrix inequality,3 and the last inequality is from Assumption OLS.5. So by the CLT, n 1 d xiui N (0; ) : pn ! i=1 X 1 n p Given that n xix Q, i=1 i0 ! P d 1 pn Q N (0; ) = N(0; V) ! by Slutsky’stheorem. b 0 2 1 0 In the homoskedastic model, V reduces to V = Q . We call V the homoskedastic covariance matrix. Sometimes, to state the asymptotic distribution of part of as in the residual regression, we partition Q and as b Q Q Q = 11 12 ; = 11 12 : (5) Q21 Q21 ! 21 22 ! Recall from the proof of the FWL theorem, 1 1 1 1 Q11 :2 Q11 :2Q12Q22 Q = 1 1 1 ; Q22 :1Q21Q11 Q22 :1 ! 1 1 where Q11:2 = Q11 Q12Q22 Q21 and Q22:1 = Q22 Q21Q11 Q12. Thus when the error is ho- 2 1 2 1 1 moskedastic, n AV ar = Q , and n ACov ; = Q Q12Q . We can also 1 11:2 1 2 11:2 22 derive the general formulas in the heteroskedastic case, but these formulas are not easily inter- pretable and so less useful.b b b Exercise 2 Of the variables (yi; yi; xi) only the pair (yi; xi) are observed. In this case, we say that yi is a latent variable. Suppose yi = xi0 + ui; E[xiui] = 0; yi = yi + vi; where vi is a measurement error satisfying E[xivi] = 0 and E[yivi] = 0. Let denote the OLS coe¢ cient from the regression of yi on xi. b 3 Schwarz matrix inequality: For any random m n matrices X and Y, X0Y X Y . This is a special form k k k k k k of the Cauchy-Schwarz inequality, where the inner product is de…ned as X; Y = X0Y . h i k k 4 (i) Is the coe¢ cient from the linear projection of yi on xi? (ii) Is consistent for ? (iii) Findb the asymptotic distribution of pn . 1.3 LSE as a MoM Estimator b The LSE is a MoM estimator. The corresponding moment conditions are the orthogonal conditions E [xu] = 0; where u = y x . So the sample analog is the normal equation 0 1 n xi yi x0 = 0; n i i=1 X 2 the solution of which is exactly the LSE. Now, M = E [xix ] = Q, and = E xix u , so i0 i0 i pn d N (0; V) ; ! the same as in Theorem 3. Note that theb asymptotic variance V takes the sandwich form. The larger the E [xixi0 ], the smaller the V. Although the LSE is a MoM estimator, it is a special MoM estimator because it can be treated as a "projection" estimator. We provide more intuition on the asymptotic variance of below. Consider a simple linear regression model b yi = xi + ui; where E[xi] is normalized to be 0. From introductory econometrics courses, n x y i i Cov(x; y) = i=1 = ; Pn x2 V ar(x) i d b i=1 P d and under homoskedasticity, 2 AV ar = : nV ar(x) b @E[xu] So the larger the V ar(x), the smaller the AV ar .
Details
-
File Typepdf
-
Upload Time-
-
Content LanguagesEnglish
-
Upload UserAnonymous/Not logged-in
-
File Pages35 Page
-
File Size-