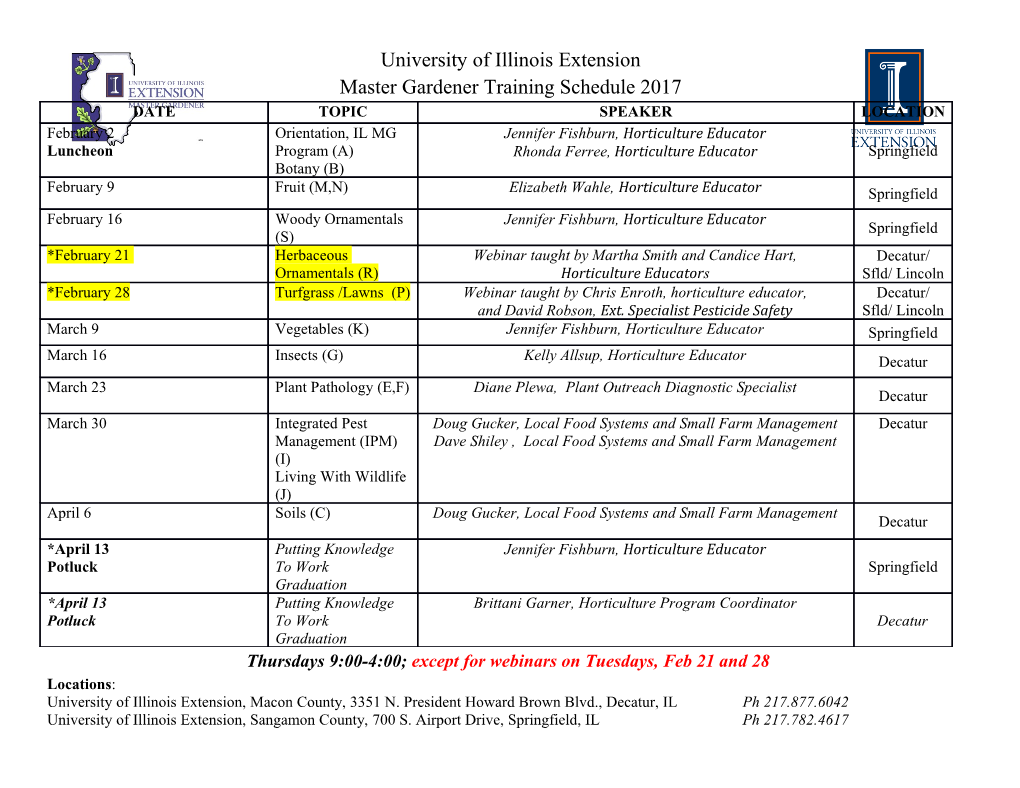
Understanding individual and collective mobility patterns from smart card records: A case study in Shenzhen The MIT Faculty has made this article openly available. Please share how this access benefits you. Your story matters. Citation Liang Liu et al. “Understanding individual and collective mobility patterns from smart card records: A case study in Shenzhen.” Intelligent Transportation Systems, 2009. ITSC '09. 12th International IEEE Conference on. 2009. 1-6. © 2010 Institute of Electrical and Electronics Engineers. As Published http://dx.doi.org/10.1109/ITSC.2009.5309662 Publisher Institute of Electrical and Electronics Engineers Version Final published version Citable link http://hdl.handle.net/1721.1/58794 Terms of Use Article is made available in accordance with the publisher's policy and may be subject to US copyright law. Please refer to the publisher's site for terms of use. Proceedings of the 12th International IEEE Conference WeBT4.4 on Intelligent Transportation Systems, St. Louis, MO, USA, October 3-7, 2009 Understanding individual and collective mobility patterns from smart card records: a case study in Shenzhen Liang Liu, Anyang Hou, Assaf Biderman, Carlo Jun Chen Ratti School of Transportation SENSEable City Lab Tongji University Massachusetts Institute of Technology Shanghai, China Cambridge, MA, USA [email protected] {liuliang, cnhanya,abider,ratti}@mit.edu Abstract—Understanding the dynamics of the inhabitants’ behavioral data have begun to emerge. For example, daily mobility patterns is essential for the planning and Rattenbury et al. [3] and Girardin et al. [4] used geo- management of urban facilities and services. In this paper, tagging patterns of photographs in Flickr to automatically novel aspects of human mobility patterns are investigated detect interesting real-world events and draw conclusions by means of smart card data. Using extensive smart card about the flow of tourists in a city. In addition, as city-wide records resolved in both time and space, we study the urban infrastructures such as buses, taxis, subways, public mean collective spatial and temporal mobility patterns at utilities, and roads become digitized, other sources of real- large scales and reveal the regularity of these patterns. We world datasets that can be implicitly sensed are becoming available. Ratti et al. [5], Reades et al.[6] and González et also investigate patterns of travel behavior at the al. [7] used cellular network data to study city dynamics individual level and show that the concentricity and and human mobility. McNamara et al. [8] used data regularity of mobility patterns. The analytical collected from an RFID-enabled subway system to predict methodologies to spatially and temporally quantify, co-location patterns amongst mass transit users. Such visualize, and examine urban mobility patterns developed sources of data are ever-expanding and offer large, in this paper could provide decision support for transport underexplored datasets of physically-based interactions planning and management. with the real world. Keywords-mobility pattern; smart card; intelligent transportation system (ITS); visualization; regularity In this paper, we introduce a novel method for understanding human mobility in dense urban area based on millions of smart card records. We show how these data I. INTRODUCTION regarding the position and intensity of digital footprint can The city never sleeps. The human movement be used to infer cultural and geographic aspects of the city constitutes the pulse of the city. Observing and modeling and reveal urban mobility pattern, which corresponds to human movement in urban environments is central to human movement in the city. traffic forecasting, understanding the spread of biological viruses, designing location-based services, and improving In particular, the main contributions of this paper are: urban infrastructure. However, little has changed since (1) demonstrating the potential of using smart card records Whyte [1] observed in his "Street Life Project" that the as data sources to gain insights into city dynamics and actual usage of New York's streets and squares clashed aggregated human behavior; (2) exploring the relationship with the original ideas of architects and city planners. A between spatiotemporal patterns of smart card usage and key difficulty faced by urban planners, virologists, and underlying city behavior and geography; and (3) studying social scientists is that obtaining large, real-world patterns in smart card usage, including an analysis of how observational data of human movement is challenging and factors such as the time of the day affect this prediction. costly [2]. We believe this work not only has direct implications In recent years, the large deployment of pervasive for the design and operation of future urban public technologies in cities has led to a massive increase in the transport systems (e.g., more precise bus/subway volume of records of where people have been and when scheduling, improved service to public transport users), but they were there. These records are the digital footprint of also for urban planning (e.g., for transit oriented urban individual mobility pattern. As websites have evolved to development), traffic forecasting, the social sciences [9]— offer geo-located services, new sources of real-world in particular, studying how people move about a city—and 842 978-1-4244-5521-8/09/$26.00 ©2009 IEEE Authorized licensed use limited to: MIT Libraries. Downloaded on April 21,2010 at 15:09:57 UTC from IEEE Xplore. Restrictions apply. the development of novel context-based mobile services. journey, we could get the time and location of check-in In addition, we expect that similar types of analyses can be and check-out, from which we could infer the OD feature applied to other sources of urban digital traces such as of trip. Moreover, the transfer information could be those provided by parking management (e.g., San derived from the smart card data. Francisco’s SFpark) and cellular networks [7]. Our work thus emphasizes the increasing role that data mining and Right now Shenzhen Metro Phase 1 is composed by visualization techniques will play to assist the east part of line 1 and south part of line 4. The east part of aforementioned fields in analyzing traces of human line 1 is from Luo Huo Railway station to Shijiezhichuang; behavior. Our work seems to open the way to a new the south of line 4 is from Futian Port to Shaoniangong. approach to the understanding of urban systems, which we The total length of Shenzhen Metro Phase 1 is 21.866 have termed “Urban Mobility Landscapes.” Urban kilometers, and there are 19 subway stops in total (the Mobility Landscape could give new answers to long- detailed information is showed in figure 1. The land use standing questions in urban planning: how to map vehicle information is in table 2). origins and destinations? How to understand the patterns of inhabitant movement? How to highlight critical points in the urban infrastructure? What is the relationship between urban forms and flows? And so on. This paper is organized by the following sequences: in section II, we describe the data sets and the feature extraction process; in section III, we investigate the aggregated spatiotemporal patterns of urban mobility; in Figure 1. Shenzhen subway stop description [11] section IV, the individual mobility pattern is explored; in section V, we draw the conclusion and discuss the future work. TABLE II. LAND USE INFORMATION OF DIFFERENT SUBWAY STOPS II. DATASETS DESCRIPTION code name Land use The datasets used to describe urban mobility patterns 1 Luohu External transport cover two major public transport modes, i.e. bus, subway. 2 Guomao Business For smart card data, according to the survey conducted by 3 Laojie Recreation the smart card company, there are 55% passengers using 4 Dajuyuan Business (CBD) smart card in bus trip and 61% passengers using smart card in subway trip [10].The smart card data is from 5 million 5 Kexueguan government Business and recreation smart card users’ transit records for one month, through 6 HuaqiangRd December 1st, 2008 to December 31st, 2008. Every day (CBD) there are 1.5 million transit records from the users. The 7 Gangxia residential smart card data description and sample is showed in table 8 HuizhanZhongxin business 1. 9 GouwuGongyuan business 11 Xiangmihu recreation TABLE I. SMART CARD DATA DESCRIPTION 12 Chegongmiao business Field Memo 13 Zhuzilin Ex- transport Date December 5th , 2008 14 Qiaochengdong residential Time 15:02:36 15 Huaqiaocheng residential CardId Anonymous unique user card id 16 Shijiezhichuang transport Tradetype Different transit behavior: 31- bus 17 Futiankouan Ex-transport boarding, 21- subway check-in, 22- 18 Fumin residential subway check-out 19 ShimingZhongxin government terminalid For bus, it represents bus id; for subway, 21 Shaoniangong government it represents the stop id III. AGGREGATED SPATIOTEMPORAL MOBILITY trademoney Final fare payment after discount (cent) PATTERN Tradevalue The full payment/the original fare (cent) transfer Transfer discount (cent) Before exploring the implications of urban mobility landscape to urban planning, we discuss temporal and spatiotemporal patterns and highlight how these patterns From the smart card data, we could infer two major reflect underlying cultural and spatial characteristics of public transit modes: bus and subway. For bus journey, we Shenzhen. could get the boarding time and travel fare; for the subway 843 Authorized licensed use limited to: MIT Libraries. Downloaded on April 21,2010 at 15:09:57 UTC from IEEE Xplore. Restrictions apply. A. Temporal patterns of public transit passengers B. Spatiotemporal patterns of subway passengers Through statistics of smart card records of the bus and Through detailed analysis of daily check-in and check- subway in different day, their temporal mobility patterns out in different subway stops, we could get the could be inferred. The temporal pattern of bus trip and spatiotemporal patterns of subway travel. The proportion subway trip is showed in figure 1.
Details
-
File Typepdf
-
Upload Time-
-
Content LanguagesEnglish
-
Upload UserAnonymous/Not logged-in
-
File Pages7 Page
-
File Size-