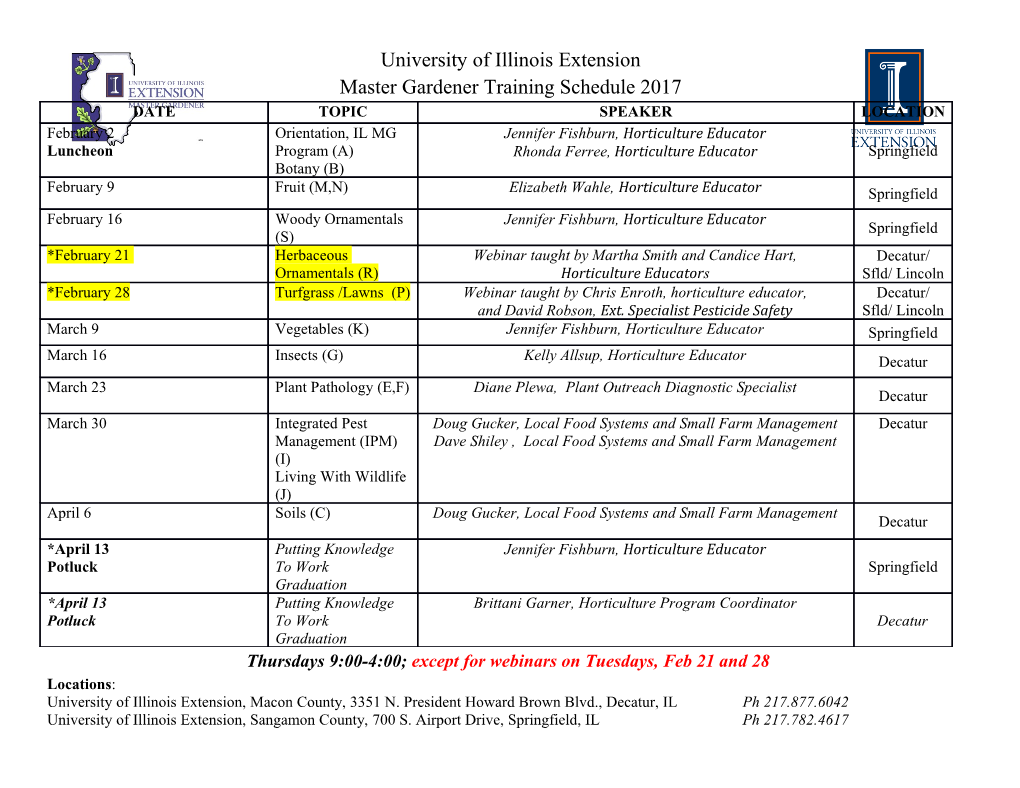
Fisher Information in Censored Samples from Univariate and Bivariate Populations and Their Applications Dissertation Presented in Partial Fulfillment of the Requirements for the Degree Doctor of Philosophy in the Graduate School of The Ohio State University By Lira Pi, M.S. Graduate Program in Statistics The Ohio State University 2012 Dissertation Committee: Haikady N. Nagaraja, Advisor Steven N. MacEachern Omer¨ Ozt¨ urk¨ c Copyright by Lira Pi 2012 Abstract This research explores many analytical features of Fisher information (FI) in censored samples from univariate as well as bivariate populations and discusses their applications. The FI in censored samples is utilized to obtain the asymptotic variance of the associated maximum likelihood estimator (MLE) in censored samples and to assess Asymptotic Rel- ative Efficiency (ARE) of an estimator. We primarily focus on the FI contained in Type-II censored samples. The FI plays a significant role in determining an optimal sample size in a life-testing experiment while taking the expected duration of the experiment into account. In Chapter 2 we investigate the linkage between unfolded and folded distributions in terms of FI in order statistics and in Type-II censored samples for symmetric distributions. For instance, exponential distribution with mean θ can be viewed as the folded distribution arising from a Laplace distribution with location parameter at zero and scale parameter θ. We exploit this connection to simplify the efforts in finding the FI in order statistics and in Type-II censored samples from an unfolded distribution that is symmetric about zero. We have shown that 4n − 3 independent computations of the expectations of special functions of order statistics from the folded distribution are needed to obtain FI in all single order statistics and all Type-II (right or left or doubly) censored samples for all random samples of size m up to n. We use this efficient approach to find the FI in order statistics and Type- II censored samples from the Laplace distribution using the expectations of functions of exponential order statistics that can be easily obtained. ii We present in Chapter 3 the FI matrix (FIM) in censored samples from a mixture of two exponentials when the mixing proportion θ is unknown and when it is known. We consider a mixture of two exponentials with pdf given by θαe−αx + (1 − θ)βe−βx (x > 0). It is proved that every entry of the FIM is finite. As closed form expressions do not exist we pursue the simulation approach to generate reliable, close approximations to the elements of the FIM. However we found that the determinants of FIM are almost zero for any as- sumed values of α, β, and θ when n is small. This supports the general knowledge that a very large sample is needed for a precise estimation of parameters in a finite mixture of exponential distributions. Let Xi:n be the ith order statistic and Y[i:n] be its concomitant obtained from a random sample from the absolutely continuous Block-Basu (1974) bivariate exponential random variable (X; Y ) with parameters λ1; λ2, and λ12. For this model, Chapter 4 provides ex- pressions for the elements of the FIM in censored samples f(Xi:n;Y[i:n]; 1 ≤ i ≤ rg and studies the growth pattern of the FI relative to the total FI in the sample on λ1; λ2, and λ12 as r=n changes in (0,1). This is done for small and large sample sizes. The results show that the FI on λ1, the parameter associated with X, is always greater than the FI on λ2, associated with the concomitant Y . We calculate the FI per unit of experimental duration to suggest optimal sample sizes for life-testing experiments. We describe its implications on the design of censored trials. In all of our investigations we also consider left and doubly censored samples. iii Dedicated to my husband, Hyeong-Tak and to my parents, Jae-Ho and Yong-Sook iv ACKNOWLEDGEMENTS I gratefully acknowledge the favor of several people who mitigate the toughness of aca- demic career at the Ohio State. My research and thesis have been well developed under the delicate advice and super- vision of Dr. Nagaraja. He inspired me with confidence and independent thinking about problems of mathematical statistics. As time goes on, I was more impressed by his good personality and enthusiasm to help students. He also provided me with a desirable insight and it makes a large influence on my view of life. For this and everything else I am sincerely grateful to him. I would also like to take this opportunity to express my appreciation to Dr. Ozturk and Dr. MacEachern. Their valuable comments during candidacy and final oral exams helped my narrow view of research expand. I would like to thank the department of Statistics for providing the facilities to work and for its continuous financial support all along. I would like to thank all my professors at Ewha Womans University, Seoul, Korea for encouraging me to pursue Ph.D degree in USA. In particular Dr. So paid his attention to my academic career and gave me expert advice on research. I gratefully thank my parents Jae-Ho and Yong-Sook and my sister Soo-Jin for en- couraging me with their profound praying. Also I would like to thank my parents in law Su-Dong and Jung-Ae. Their belief in my abilities kept driving me to work harder towards my goal. I thank my husband Hyeong-Tak for his constant support. There is no suitable v word to sufficiently express appreciation of all he has done for my academic career. His love and affection have helped me overcome some of the toughest days of my life and I will be forever indebted to him for that. Last but not least, I should thank my lord, God. His spirit has been always accompanying by me and guiding me onto the right path. vi Contents Page ABSTRACT . ii Dedication . iv Acknowledgments . .v List of Tables . .x List of Figures . xiii 1. Introduction . .1 1.1 Fisher Information in Order Statistics and their Concomitants . .1 1.1.1 Properties of Concomitants . .1 1.1.2 Fisher Information in Univariate Censored samples . .2 1.1.3 Fisher Information in Bivariate Censored samples . .3 1.1.4 Use of Fisher Information in Censored samples . 10 1.2 Univariate Models . 11 1.2.1 Relationship between Folded and Unfolded populations . 11 1.2.2 A Mixture of Finite Exponential populations . 12 1.3 Bivariate Exponential Models . 13 1.4 Motivation and Summary of Work . 14 2. Connections between Fisher Information in Type-II Censored samples from Folded and Unfolded populations . 16 2.1 Introduction . 16 2.2 Fisher Information in a Single Order statistic from Unfolded population . 19 vii 2.3 Connection between Fisher Information in Type-II Censored samples from Folded and Unfolded populations . 33 2.4 An Illustrative Example . 44 3. Fisher Information from a Mixture of Finite Exponential distributions and its Type-II censored samples . 52 3.1 Introduction . 52 3.2 Fisher Information in Type-II Censored samples from a Mixture of Two Exponentials with Unknown p ....................... 53 3.3 Fisher Information in Type-II Censored samples from a Mixture of Two Exponentials with Known θ ........................ 58 3.4 Application and Numerical Integration . 59 4. Fisher Information in Type-II censored samples from Block-Basu Bivariate Ex- ponential distribution . 69 4.1 Introduction . 69 4.2 Block and Basu Bivariate Exponential distribution . 70 4.3 Fisher Information in Type-II Censored samples from BBVE . 72 4.3.1 Right Censored Samples . 72 4.3.2 Left Censored Samples . 83 4.3.3 Limiting Fisher Information Matrix . 91 4.4 Computations . 93 4.4.1 Right Censored Samples - Finite Sample Case . 93 4.4.2 Limiting FIM for Right Censored Samples . 101 4.4.3 Left and Doubly Censored Samples . 103 5. Conclusion . 105 5.1 Concluding Remarks . 105 5.2 Future Work . 106 Bibliography . 108 Appendix A. Notations and Abbreviations . 111 A.1 Symbols . 111 A.2 Abbreviations . 112 A.3 Distributions . 112 viii Appendix B. R codes . 113 B.1 Numerical Integration . 113 B.2 Simulation . 115 ix List of Tables Table Page f 2.1 Ir:m from Laplace(0, 2) for 1 ≤ r ≤ m ≤ n when n = 10 using (2.1.1) . 45 2.2 The values of ab1:i; c1:i; d1:i; e1:1; and k1:i for the Exp(2) distribution in Lemma 2.2.1 for 1 ≤ i ≤ 10 ......................... 46 2.3 The values of abr:m from Exp(2) parent for 1 ≤ r ≤ m ≤ 10 ........ 47 2.4 The values of cr:m from Exp(2) for 1 ≤ r ≤ m ≤ 10 ............ 47 2.5 The values of dr:m from Exp(2) for 1 ≤ r ≤ m ≤ 10 ............ 47 2.6 The values of er:m from Exp(2) for 1 ≤ r ≤ m ≤ 10 ............ 48 f 2.7 Ir:m from Laplace(0, 2) using Theorem 2.2.1 for 1 ≤ r ≤ m ≤ 10 ..... 48 f 2.8 I1···r:m from Laplace(0, 2) using Theorem 2.3.1 for 1 ≤ r ≤ m ≤ 10 .... 49 2.9 Proportional FI from Laplace (0, 2) . 50 2.10 ARE values for Laplace (0, 2) distribution . 51 3.1 I(X; α; β; θ) from MExp(15, 1, θ) with known θ and unknown θ ...... 59 3.2 I(X; α; β; θ) from MExp(2, 1, θ) with known θ and unknown θ ....... 60 3.3 I(X; α; β; θ) from MExp(15, 2, θ) with known θ and unknown θ .....
Details
-
File Typepdf
-
Upload Time-
-
Content LanguagesEnglish
-
Upload UserAnonymous/Not logged-in
-
File Pages132 Page
-
File Size-