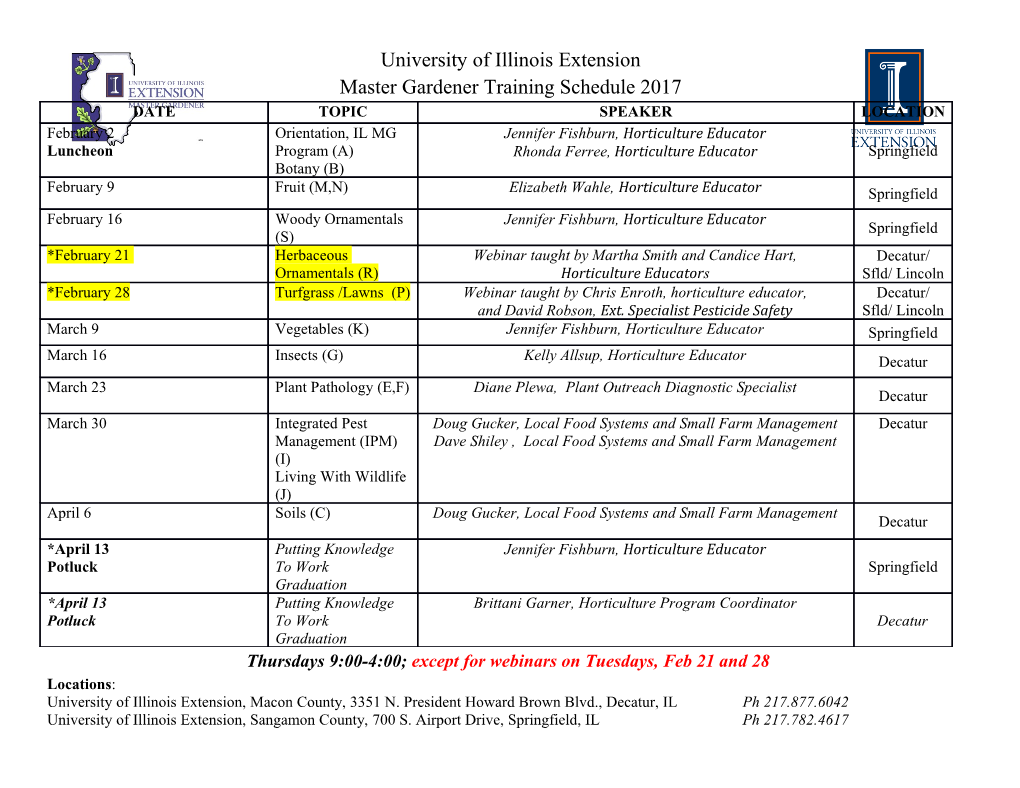
UAV IMAGERY FOR TREE SPECIES CLASSIFICATION IN HAWAI‘I: A COMPARISON OF MLC, RF, AND CNN SUPERVISED CLASSIFICATION A THESIS SUBMITTED TO THE GRADUATE DIVISION OF THE UNIVERSITY OF HAWAI‘I AT MĀNOA IN PARTIAL FULFILLMENT OF THE REQUIREMENTS FOR THE DEGREE OF MASTER OF ART IN GEOGRAPHY November 2020 By Derek James Ford Thesis Committee: Qi Chen, Chairperson Yi Qiang Clay Trauernicht Keywords: convolutional neural network, deep learning, supervised classification, UAV, tree species, remote sensing, forest ecology i © Copyright 2020 By Derek James Ford ii ACKNOWLEDGEMENTS I’d like to thank everyone involved in this project including my graduate advisor Dr. Qi Chen, whose expertise and encouragement to pursue exciting research topics was crucial for my thesis development, committee members Dr. Clay Trauernicht and Dr. Yi Qiang, Natural Area Reserves specialist James Harmon, and the entire department of Geography faculty, staff and students. I am fortunate to have been able to experience the diversity of interests and perspectives within the department, and I feel more fortunate now than ever, to have been able to do so in-person and less than six feet away! I’d also like to thank everyone who I’ve had the opportunity to work with during my Master’s degree studies, including Dr. Kirsten Oleson and all the Oleson Lab members, Dr. Brian Szuster, and Dr. David Beilman. The work opportunities you provided tremendously increased my depth and breadth of knowledge in GIS and remote sensing for a variety of applications, an invaluable addition to my formal coursework. I’d finally like to thank everyone involved in my life in general for your direct or indirect encouragement for me to pursue my interests. I’d especially like to thank my lovely wife Tessie, my loving family and friends, and of course my dog and two cats. You all have good qualities and values which I admire and work towards, and you also remind me to enjoy every step of the way. iii ABSTRACT Very-high resolution unmanned aerial vehicle (UAV) imagery coupled with emergent automated classification methods show great promise for fast and affordable remote sensing analysis. Tree species classification through remote sensing has traditionally been limited by spatial resolution of satellite imagery, or cost and logistics associated with aerial imagery collection. In this study, the use of red-green-blue (RGB) UAV imagery was assessed for supervised classification of multiple tree species within a tropical wet forest in Hawai‘i characterized by high species diversity and limited site accessibility. Three classifiers were tested: maximum likelihood classifier (MLC), random forest (RF), and convolutional neural network (CNN) U-Net. MLC and RF were additionally tested with the addition of texture statistics. U-Net achieved highest overall accuracy of 71.2%, compared to MLC with 48.1% and RF with 52.1%. MLC and RF both benefited from the addition of texture statistics. This study presents a novel comparison of three important classifier types and their capabilities with an emergent remote sensing data source. Findings from this study are consistent with those of recent studies and suggest that easily-acquirable RGB UAV imagery contains the necessary information for fine-grained classification at the species level, especially when utilizing a CNN. iv TABLE OF CONTENTS Acknowledgements……………………………………………………………………………...iii Abstract…………………………………………………………………………………………..iv Table of Contents………………………………………………………………………………...v List of Tables…………………………………………………………………………………...viii List of Figures……………………………………………………………………………………ix Chapter 1. Introduction…………………………………………………………………………1 Chapter 2. Review of Literature………………………………………………………………..6 2.1 Remote Sensing Data Sources………………………………………………………………...6 2.1.1 Satellites………………….…………………………………………………………5 2.1.2 Manned Aircraft……………………………………………………………………10 2.1.3 UAV…………………………………….…………………………………………..12 2.2 Image Classification………………………………………………………………………….24 2.3 Remote Sensing Tree Species Classification in Hawaii……..………………………………28 Chapter 3. Materials and Methods.............................................................................................30 3.1 Study Area…………………………………………………………………………………...30 3.1.1 Site Description……………………………………………………………………30 3.1.2 Description of Vegetation…………………………………………………………34 v 3.2 Data Collection………………………………………………………………………………43 3.3 Data Processing………………………………………………………………………………47 3.4 Class Selection……………………………………………………………………………….54 3.5 Reference Data……………………………………………………………………………….55 3.6 Texture Statistics……………………………………………………………………………..58 3.7 Classification Methods……………………………………………………………………….61 3.7.1 Maximum Likelihood Classification (MLC)……….………………………………61 3.7.2 Random Forest (RF)……………………………………………………………….62 3.7.3 Convolutional Neural Network (CNN) U-Net………………………….………….65 3.8 Accuracy Assessment………………………………………………………………………..73 Chapter 4. Results........................................................................................................................76 4.1 Accuracy Assessment………………………………………………………………………..76 4.1.1 Overall Accuracy (OA) and Kappa Coefficient……………………………………76 4.1.2 Use of Texture Measures…………………………………………………………..77 4.1.3 Class Performances………………………………………………………………..79 4.1.4 Visual Inspection…………………………………………………………………..83 4.2 U-Net Optimization………………………………………………………………………….85 Chapter 5. Discussion..................................................................................................................87 vi 5.1 Texture Measures…………………………………………………………………………….87 5.2 Classifier Performances……………………………………………………………………...88 5.3 Class Considerations…………………………………………………………………………89 5.3.1 Class Selection……………………………………………………………………..89 5.3.2 Class Performances………………………………………………………………..90 5.4 U-Net Optimization………………………………………………………………………….93 Chapter 6. Conclusion.................................................................................................................94 Supplementary Material……………………………………………………………………….98 References...................................................................................................................................105 vii LIST OF TABLES Table 1 List of satellite systems which provide near-global coverage and are commonly used for tree species classification………………………………………………………………………….9 Table 2 List of studies using UAV imagery for tree species or vegetation classification…....….18 Table 3 Training data per-class pixel count and median frequency values assigned for class weights…………………………………………………………………………………………...73 Table 4 Overall accuracy (OA) and kappa coefficient scores for the three classifiers...………...77 Table 5 UA and PA for top-performing versions of each classifier…………...………….……..81 viii LIST OF FIGURES Figure 1 Study area within the Pūpūkea-Paumalu Forest Reserve on O‘ahu, Hawai‘i………….32 Figure 2 Forest type mapping for Kaua‘i and O‘ahu (Little & Skolmen 1989)…………………33 Figure 3 Strawberry Guava………………………………………………………………………39 Figure 4 Ironwood………………………………………………………………………………..40 Figure 5 Eucalypt……………………………………………………………………..………….40 Figure 6 African Tulip……………………………………………………………….…………..41 Figure 7 Uluhe…………………………………………………………………………………...41 Figure 8 'Ōhi'a lehua………………………………………………………………….………….42 Figure 9 Trumpet Tree…………………………………………………………………..……….42 Figure 10 Bare ground………………………………………………………………..………….43 Figure 11 DJI Mavic Pro UAV used for data collection……………………………...…………45 Figure 12 Mapping flight planning in the DroneDeploy app………………………..…………..46 Figure 13 Adding images to Agisoft workspace……………….……………………………..….48 Figure 14 After image alignment in Agisoft………………….………………………………….49 Figure 15 Dense cloud production in Agisoft……………………………………………………50 Figure 16 Horizontal view of the dense cloud…………………………………………..……….51 ix Figure 17 Magnified view of dense cloud……………………………………………………….51 Figure 18 DSM generated in Agisoft………………………………………………...…………..52 Figure 19 Orthomosaic generated in Agisoft………………………………………………...…..53 Figure 20 Study area………………………………………………………………….………….57 Figure 21 Pixel value frequencies for each class…………………………...……………………58 Figure 22 Depiction of MLC classifier feature space (“Maximum Likelihood Classifier”, 1999).…………………………………………………………………………………………….62 Figure 23 Simplified depiction of RF classifier design………………………………………….65 Figure 24 Example U-Net architecture, adapted from Ronneberger et al. (2015)……………….68 Figure 25 Input image sizes tested……………………………………………………………….70 Figure 26 User’s Accuracy (UA) for MLC and RF without and with texture measures………...78 Figure 27 Producer’s Accuracy (PA) for MLC and RF without and with texture measures…….78 Figure 28 True versus predicted class pixel counts for each classifier…………………..………82 Figure 29 Example classification outputs from MLC and RF before and after the addition of texture data ………………………………………………………………………………..……..83 Figure 30 Example classification outputs from optimized MLC, RF and U-Net.………...……..84 Figure 31 Whole study area classification outputs from optimized MLC, RF and U-Net………85 x CHAPTER 1. INTRODUCTION Forests and natural areas across the globe provide invaluable ecosystem services which humans and many other species depend on for their survival. These services include but are not limited to: climate regulation, waste treatment, production of food and raw materials, and cultural or recreational enjoyment (Krieger 2001). Biodiversity within such areas has been identified as a major component of healthy ecosystems which have the capacity to deliver maximum ecosystem services (Gamfeldt et
Details
-
File Typepdf
-
Upload Time-
-
Content LanguagesEnglish
-
Upload UserAnonymous/Not logged-in
-
File Pages134 Page
-
File Size-