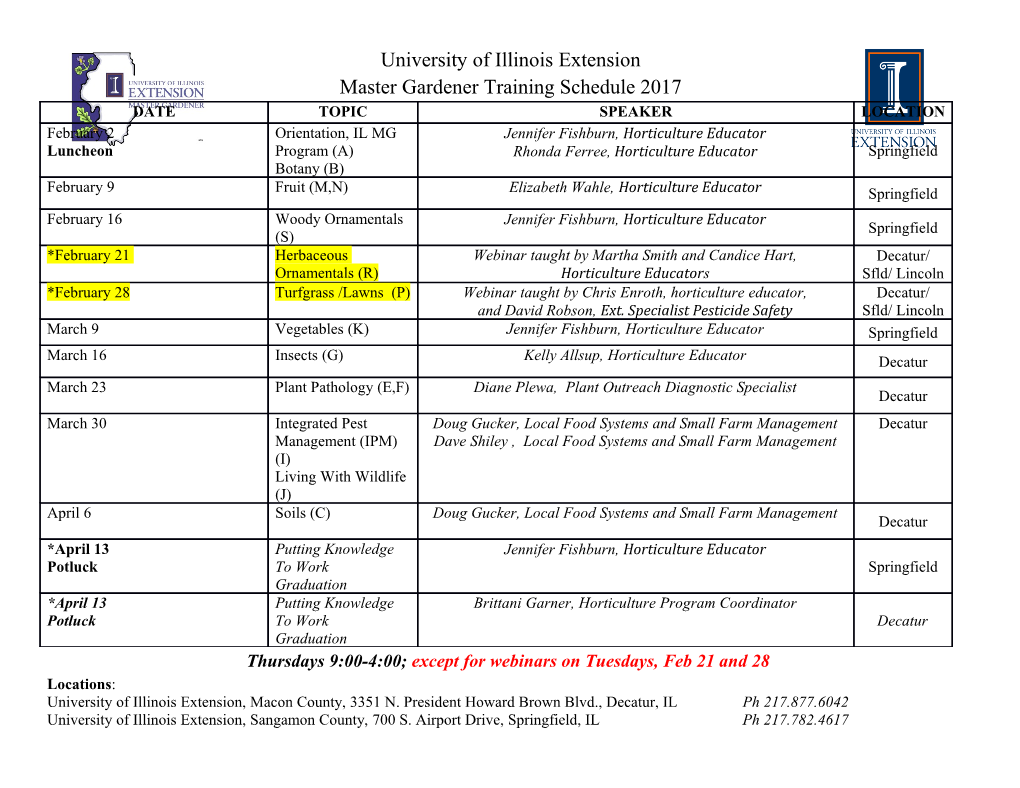
https://doi.org/10.5194/tc-2020-52 Preprint. Discussion started: 3 April 2020 c Author(s) 2020. CC BY 4.0 License. Seasonal and interannual variability of sea-ice state variables: Observations and predictions for landfast ice in northern Alaska and Svalbard 5 Marc Oggier1, Hajo Eicken1, Meibing Jin1, Knut Høyland2,3 1: International Arctic Research Center, University of Alaska Fairbanks, PO Box 757340, Fairbanks, AK 99775, USA 2: Norwegian University of Science and Technology, Høgskoleringen 7a, NO-7491 Trondheim, Norway, 3: The University Centre in Svalbard, P.O. Box 156, N-9171 Longyearbyen, Norway 10 Correspondence to: Marc Oggier ([email protected]) Abstract. Validation of sea-ice models, representation of sea-ice processes in large-scale models, and regional planning around ice use and hazards requires climatological ice property data. We summarize key ice properties, in particular temperature and salinity, representative of broader Arctic conditions, from long- 15 term observations near Utqiaġvik, Alaska and Van Mijen Fjord, Svalbard. Additionally, we simulate salinity and temperature profiles using the Los Alamos sea-ice model (CICE) in stand-alone mode, forced with meteorological data for both locations. We compare observations and model results by aggregating profiles using a degree day model and statistical analysis to create ice property climatologies, which describe the seasonal evolution of sea ice. During the growth season, the CICE model accurately replicates ice property 20 evolution for both salinity (R=0.7) and temperature (R=0.9). While the model initiates ice desalination at melt onset, and reproduces the temperature field well through melt (R=0.9), model salinities later tend towards an asymptotic value of 5‰ (R=0.3). This suggests that the model does not fully capture the desalination processes and their impact on ice physico-chemical properties during the melt season. Overall, the standard deviation of the model remains similar to the natural sea-ice variability throughout the season. Despite mismatches during 25 the melt season, the CICE model shows promise for simulating the seasonal evolution of salinity and temperature profiles, which may serve as proxies for bulk ice properties that constrain transport of heat and mass through sea ice. Our findings highlight the necessity for a large number of observations throughout the year to create an effective model benchmarking dataset. 1 https://doi.org/10.5194/tc-2020-52 Preprint. Discussion started: 3 April 2020 c Author(s) 2020. CC BY 4.0 License. 1. Introduction 30 Sea ice controls the interaction between ocean and atmosphere and is a key aspect of polar marine ecosystems (Cottier et al., 1999). Heat transport and light transmission across the ocean-ice-air interface are controlled by sea-ice properties, ice microstructure, and stratigraphy. Ice transport properties also govern the sea-ice role as a habitat for a broad range of microscopic organisms thriving in the bottommost ice layer, which in turn supports zooplankton grazers and higher trophic levels (Bluhm and Gradinger, 2008; Gradinger et al., 35 2010; Horner et al., 1992). Increasing industrial and maritime activities in the Arctic have the potential to stress the ecosystem. The risk of oil spills is of particular concern, with increasing ship traffic and continued interest in offshore hydrocarbon development (Brigham, 2010; Eguíluz et al., 2016; Smith and Stephenson, 2013). Information about the state of the sea-ice cover, and key sea-ice properties relevant to transport processes and entrainment of pollutants is critical in this context as well. In addition, compilation and synthesis of existing 40 datasets and model simulations can help provide important baseline data for a range of studies concerned with air-ocean interaction, and the ecological significance of sea ice. Sea ice is a composite material, with brine trapped within the ice matrix during sea-ice growth. Under quiescent ice growth conditions typical of the Arctic, a high-porosity skeletal layer of few mm to cm thickness with fully connected mm- to sub-mm pore space makes up the bottommost ice (Petrich and Eicken, 2017). In growing 45 sea ice, brine channels several cm to dm in vertical extent develop as a result of convective overturning and drainage processes (Cole and Shapiro, 1998; Wells et al., 2011). The brine-filled pore space responds to cooling or warming through increases or decreases, respectively, in the brine volume fraction. These changes in turn affect pore microstructure and ice permeability, and impact macro-scale behaviour (Petrich and Eicken, 2017). 50 Assur (1960) and Zubov (1963) summarized years of field observations of pioneering physical and chemical sea-ice property measurements in the Arctic. Thorough analysis of sea ice led to the development of semi- empirical equations describing physical property evolution (Cox and Weeks, 1983, 1986; Leppäranta and Manninen, 1988). In addition, studies of the temporal evolution and spatial variability of sea-ice salinity and property profiles in the Arctic (e.g., Cox & Weeks, 1988a; Nakawo & Sinha, 1981) and Antarctic (Eicken, 1992; 55 Gough et al., 2012) provided further insights into processes impacting the vertical profile of sea-ice properties. This work has been expanded in recent years through the application of mush layer theory (Feltham et al., 2006; Notz and Worster, 2009) and the development of in-situ measurement approaches based on the change of sea-ice dielectric property, related to the change of pore fraction and microstructure, which in turn influences key sea-ice transport properties (Jones et al., 2012; O’Sadnick et al., 2016). 60 Driven by the need to improve representation of sea-ice processes in general circulation and Earth system models, more sophisticated sea-ice models were developed, aiming in particular to represent the seasonal sea-ice cycle more accurately (Griewank and Notz, 2015; Hunke et al., 2013). The availability of ice property data that captures the full range of variability is essential for validation of such modelling efforts. At the same time, mean and variance of key ice properties as a function of ice depth are essential in constraining the 65 analysis and simulation of biogeochemical or contaminant transport processes in sea ice (Oggier et al., 2019; Steiner et al., 2016). However, obtaining sea-ice property data representative of the temporal and spatial 2 https://doi.org/10.5194/tc-2020-52 Preprint. Discussion started: 3 April 2020 c Author(s) 2020. CC BY 4.0 License. variability are challenging due to the episodic nature of typical field measurement programs, the remoteness of field sites, and the lack of a consistent sampling protocol. Sustained observations of sea-ice property evolution at a single site, where ice conditions are comparable from year to year, are scarce. 70 In this study, we assembled ice properties (temperature, salinity, ice thickness) and meteorological data for two landfast ice sites representative of broader Arctic conditions: Utqiaġvik (formerly Barrow), Alaska, and Van Mijen Fjord, Svalbard. From these data, we derived an ice property climatology along with an evaluation of seasonal and interannual variability. Drawing on this data, we assess the ability of a standard Earth system model to replicate key aspects of the seasonal cycle as well as interannual variability. We chose the Los 75 Alamos sea-ice model (CICE; Hunke et al., 2013) for its capability to be run both in fully coupled atmosphere- land global climate models and as a standalone model. We evaluated the variability from observations and model results, with a focus on properties such as porosity and permeability, which are relevant for biogeochemical processes (Miller et al., 2015) and contaminant transport (Maus et al., 2015; Petrich et al., 2006). 80 2. Methods We analyzed ice core data from two different locations, representative of a broader range of quiescent growth conditions of Arctic first-year sea ice: Utqiaġvik, located at the northern tip of Alaska and Van Mijen Fjord on the west coast of the Svalbard Archipelago, 60 km south of Longyearbyen. At each location, field measurements and ice sampling were part of sustained ice observation programs (Druckenmiller et al., 2009; 85 Ervik et al., 2014; Høyland, 2009). In this study, we consider data collected during the ice seasons from 1997/98 through 2017/18. 2.1. Study sites 2.1.1. Utqiaġvik, Alaska The field sites were located 2 to 5 km southwest of Point Barrow, in a location protected from alongshore ice 90 movement and deformation, resulting in a level landfast ice cover growing undisturbed throughout the entire length of the landfast ice season (Figure 1–a). Data were derived from ice cores and in situ measurements of ice and snow thickness, and temperature profiles through the depth of the snow and ice cover at a mass balance site (MBS) installed from the 2006/07 through the 2015/16 ice seasons (Druckenmiller et al., 2009). While the position of the station varied slightly from year to year, the sites were representative of level, 95 undeformed, landfast sea ice formed under calm conditions (Figure 1). Sampling dates were scheduled to capture and follow the fundamental changes in sea-ice state throughout the winter and spring: young first-year sea ice in January, mature sea ice from April until mid-May, and desalination and melt through mid-June. Station locations are shown in Figure 1—a. Our analysis includes 180 ice cores, with 106 cores collected during 42 growth season sampling events, and 74 ice cores for a total of 41 melt season sampling events. The 100 National Oceanic and Atmospheric Administration (NOAA) records meteorological data at Will Rogers Memorial Airport station (PABR) in Utqiaġvik. The airport is located approximately 10 km southwest of the MBS location. Temperatures between the airport and the coring site are well correlated (Figure 2). 3 https://doi.org/10.5194/tc-2020-52 Preprint.
Details
-
File Typepdf
-
Upload Time-
-
Content LanguagesEnglish
-
Upload UserAnonymous/Not logged-in
-
File Pages28 Page
-
File Size-