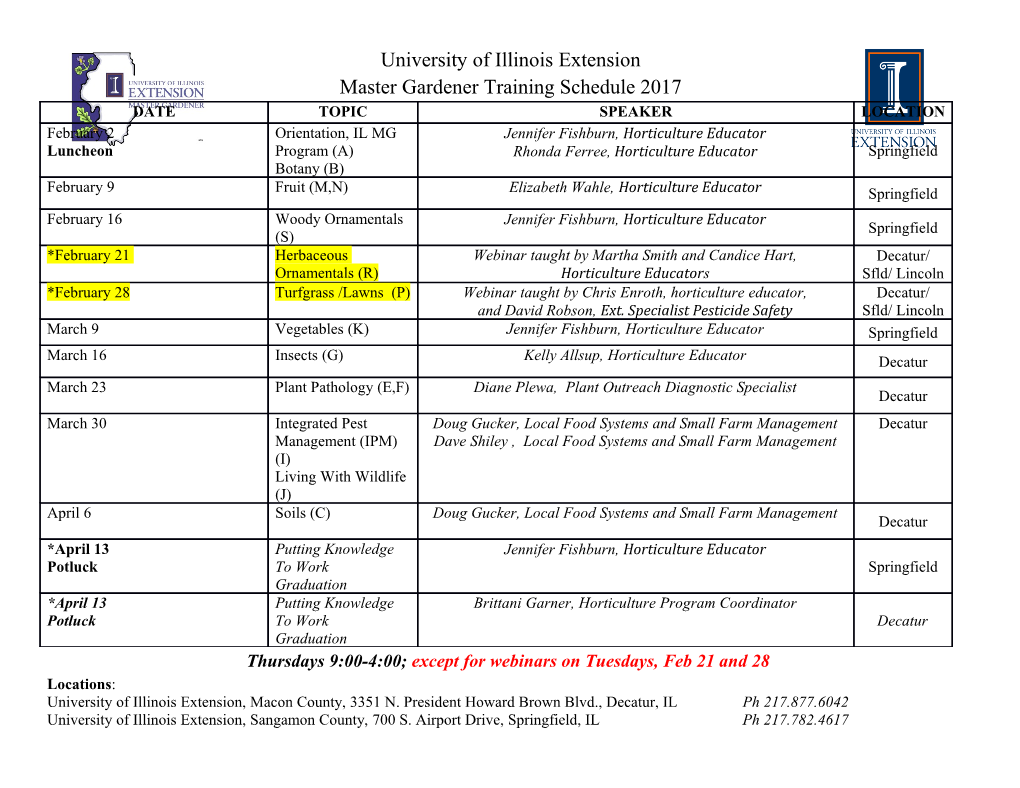
Variance Targeting for Heavy Tailed Time Series June 2012 Conference “Advances in Econometrics”, Yale SOM Jonathan Hill* Eric Renault** *University of North Carolina at Chapel Hill ** Brown University OUTLINE 1. Variance targeting in GARCH models 2. Sample variance with tail trimming 3. QMLE with (tail-trimmed) variance targeting = solution to infinite unconditional kurtosis problem 4. Trimming orthogonality conditions for GMM = solution to infinite conditional kurtosis problem 5. Monte Carlo study 1. Variance Targeting in GARCH Models • Example 1: GARCH(1,1) 1/ 2 yt1 ht1 t1 2 ht1 (yt1) ht , 0, 0, 1 Var(y ) t 1 new parameterization : ( , ), (,) Variance Targeting: • Idea = direct estimation of unconditional variance common practice: 1 T ˆ 2 (i) = estimated by sample variance: T yt T t1 (ii) (Q)MLE = applied to remaining parameters after plugging in sample variance: 2 2 ht1 yt1 ht yt1 (1 )ht 2 2 2 ˆt1,T ( ) ˆT yt1 (1 )ˆt,T ( ), (,). Cost-Benefit of variance targeting • Benefits: (i) Better finite sample performance (especially for estimation of ). (ii) Robustness to misspecification of variance equation. • Costs: (i) Efficiency loss at least if QMLE = MLE (ii) For asymptotic normality, requires a much more restrictive assumption than QMLE 4 Not only E t1 (and Eht1 ) 4 2 4 But also Eyt1 Eht1 t1 Var(ht1) Does unconditional kurtosis exist? • Existence of the unconditional fourth moment of stochastic process generating financial return data maintained interest for researchers: • He and Terasvirta (1999, ET)“Fourth Moment Structure of the GARCH(p,q) Process” R1. Existence “would enable one to see how well the kurtosis and autocorrelation (of squared returns) implied by the estimated model match the estimates obtained directly from the data” R2. Existence = far from being certain in case of volatility persistence GARCH (1,1) case: (see He and Terasvirta for general GARCH(p,q)) 1/ 2 yt1 ht1 t1 2 4 Et ( t1) 0, Et ( t1) 1, Et ( t1) 4 , 2 ht1 (yt1) ht . 4 2 2 E(yt1) 4 2 1. Conditionally normal case: 4 2 2 E(yt1) 2 ( ) 1. Pareto Tail Index • Basrak, Davis and Mikosch, SPA, 2002 “Regular variation of GARCH processes” 1/ 2 yt1 ht1 t1 2 a ht1 (yt1) ht , 0, 0, 2 / 2 P yt c c ,c Et 1 p 1 2, Eyt p 1 Var(y ) t 1 R3. Hill’s tail index estimator for daily log- returns over the period 2001-2011: 90% confidence band: SP 500 and NASDAQ : [2,3] DAX: [2, 3.5] NIKKEI : [1.8, 2.8] Conclusion: (i) Variance should be finite ( not compelling for Nikkei!) (ii)(Unconditional) moment of order 3 may exist (iii)Hard to find a series that appears to have finite unconditional moment of order 4 Hill Plots for daily log-returns on SP500 (2513 days from Jan ,1st, 2001 to Jan 1st, 2011) Multivariate examples: • Example 1: DCC-GARCH(1,1): Univariate GARCH for each asset + dynamic conditional correlations: yi,t i,t 1/ 2 conditional correlation matrix : (hii,t ) Qt conditional variance matrix of t ( i,t )1in correlation targeting in the DCC equation : Qt (1 )Q t t 'Qt1 no additional problem under 4 maintained assumption E i,t ,i. Variance targeting and parsimony • DCC example Once the unconditional individual variances and correlation matrix is estimated : only individual GARCH + two more parameters and saves N = n(n+1)/2 parameters. • Example 2: VEC-GARCH(1,1) 1/ 2 yt Ht t ,ht vech(Ht ), t vech( t t ') h c A Gh , t t1 t1 vech Var(y ) [Id A G]1c. t N Variance targeting still requires unconditional finite (matricial) kurtosis 2. Sample variance with tail trimming • Univariate case: 1 T ˆ y 2 T 0 Var(y ) T t t T t1 0 4 BUT : T ˆT asymptotically normal if E(yt ) . Key idea: Tail-Trimming of returns before computing sample variance Iˆ( y) 0 y is one of the k ( y) largest T ,t t T observations among y , 1,...,T. 1 T ˆ( y) ˆ(tr) 2 ˆ( y) IT ,t 1 otherwise, T yt IT ,t T t1 ( y) kT promotes Gaussian asymptotics ( y) (tr) 0 kT /T 0 ensures unbiasedness : ˆT Var(yt ) Asymptotic distribution of estimator of unconditional variance in GARCH: 1st case: Without trimming (with finite kurtosis): Horvath, Kokoszka,Zitikis, JFEC,2006: 1 T 1 T 1 T ˆ 2 2 T yt ht ( t 1) ht T t1 T t1 T t1 0 T 0 1 1 2 0 ht ( t 1) oP (1/ T ) T t1 2 T 1 0 v2 Var Tˆ Var y 2 T E h2 Var 2 T T t 0 t t t1 2nd case: With trimming: T 2 ˆ(tr) 2 ( y) vT VarT T Var yt I yt cT t1 2 0 2 vT 1 2 2 4 Lim E h Var if E y T 0 t t t T v2 Lim T if Ey 4 , BUT v2 o(T 2 ) T T t T Same asymptotic distribution if finite kurtosis More involved in the general case because, by trimming, we lose the mds property: Pareto tails: ( y) 2 ( y) 2 kT ( y) Pyt cT d(cT ) ,2 4 T 1/ ( y) ( y) 1/ T T kT cT (d) ( y) as 0 kT T Feller (1971) (regularly varying functions): 4 ˆ( y) ( y) 4 ( y) Eyt IT ,t (cT ) LcT , L(x) o(x ), 0 Corollary 1: ( y) 4 ˆ( y) 2 and kT Eyt IT ,t o(T) Corollary 2 (with geometric -mixing): Long term variance matrix = o(T) T 2 2 ˆ( y) 2 vT Var yt IT ,t o(T ),vT o(T). t1 4 BUT : E(yt ) vT / T 4 2 4 E(yt ) vT / T bE( t ) 1 2 1 4 b E(ht ) 1 R. Geometric -mixing for y = implied by +<1 when has an absolutely continuous distribution Asymptotic distribution of tail-trimmed estimators of unconditional variance: Long-term variance matrix = o(T) Promotes asymptotic normality of tail trimmed sample variance: T (tr) 0 d ˆT (0,1) vT ,t T 2 ˆ( y) 0 insofar as : Eyt IT 0. vT, t 2 ˆ( y) 0 Always true if : T Eyt IT 0. Lighter trimming for heavier tail (feasible from estimation of tail index) 1st improvement of tail-trimmed sample variance • Peng (2001) : “Estimating the mean of a heavy tailed distribution”, Statistics & Probability Letters Characterizes the systematic finite sample bias due to trimming: 1 T ˆ(tr) 2 ˆ( y) T yt IT ,t biased by : T t1 ( y) 2 ˆ( y) kT ( y) 2 Eyt 1 IT ,t cT 2 T k ( y) P y c( y) T t T T This bias can be( y )estimated by: ˆ 2 ˆ * kT (a) R y ( y ) T k ˆ 2 T T ( y) ˆ Hill estimator based on kT largest absolute values. 1 k ( y ) 1 (a) 1 T y ˆ i ( y) Log (a) k y ( y ) T i1 k T (a) ( y) y ( y ) component kT in order statistics of absolute values : kT (a) (a) (a) y1 y2 .... yT . Improved estimator of conditional variance based on Peng (2001): * (tr) ˆ * ˆT ˆT RT Pros and cons of Peng’s improvement • Pro 1: Avoids a systematic finite sample under- estimation of unconditional variance • Pro 2: Provides asymptotic normality without the problematic maintained assumption: T 2 ˆ( y) 0 Eyt IT 0. vT Con: The correction term is not asymptotically independent from the main term * (tr) ˆ * ˆT ˆT RT (tr) asymptotic distribution of ˆT We propose a new estimator: by under-correction for the gap, we obtain an asymptotically normal estimator with the same distribution limit as the initial one: ~( y) ˆ 2 ˆ * kT (a) R y~( y ) T k ˆ 2 T T ~( y) ( y) (a) (a) T k / k y~( y ) / y ( y ) 0 T T k k T T ( y) ˆ Hill estimator based on even more kT largest absolute values. Price to pay for this simpler bias- corrected estimator: Need to maintain the assumption: T 2 ˆ( y) ~( y) Eyt IT IT 0. vT we will work under this maintained assumption , or No-bias correction + the corresponding stronger assumption: T 2 ˆ( y) 0 Eyt IT 0. vT 3. QMLE with (tail-trimmed) variance targeting 1/ 2 2 yt1 ht1 t1,ht1 (yt1) ht . 1 , /, (,)', ( ,')' T 1/ 2 0 d T ˆT (0, Id3 ) vT NW of T 1 1 SW of T J K E( 4 ) 1 SE of t J 1 J 1KK' J 1 T 2 vT /T 1 ht ht 1 ht ht J E 2 , K E 2 ht ' ht R1. Trimming has no impact on asymptotic distribution of QML-VT when finite kurtosis (Francq,Horvath,Zakoian,JFEC, 2011) R2. In case of infinite kurtosis, the asymptotic variance of GARCH parameters (α,) (SE block) is smaller: 1 1 * T 1 1 K' J J K R3. Price to pay for variance targeting = now in terms of rate of convergence BUT Two directions in the parameter space with root-T rate of convergence: 1 2 ' J K * , () ()' () 0 T 0 ()'ˆT 0 vT 0 4 1 T ()'ˆT 0,E( t ) 1' J 1 (1,0)' ()' J K 1 1 1 (1,1)' ()' J K 1 J K 2 1 4. Trimming orthogonality conditions for GMM QML score (with re-parameterization for variance targeting): y2 1 h () 2 t t et () 1st () 1 ht () ht () 4 May need tail-trimming if E(t ) since then Gaussian QML ( Hall and Yao, 2003) is not asymptotically normal and has a slow rate of convergence R1.
Details
-
File Typepdf
-
Upload Time-
-
Content LanguagesEnglish
-
Upload UserAnonymous/Not logged-in
-
File Pages44 Page
-
File Size-