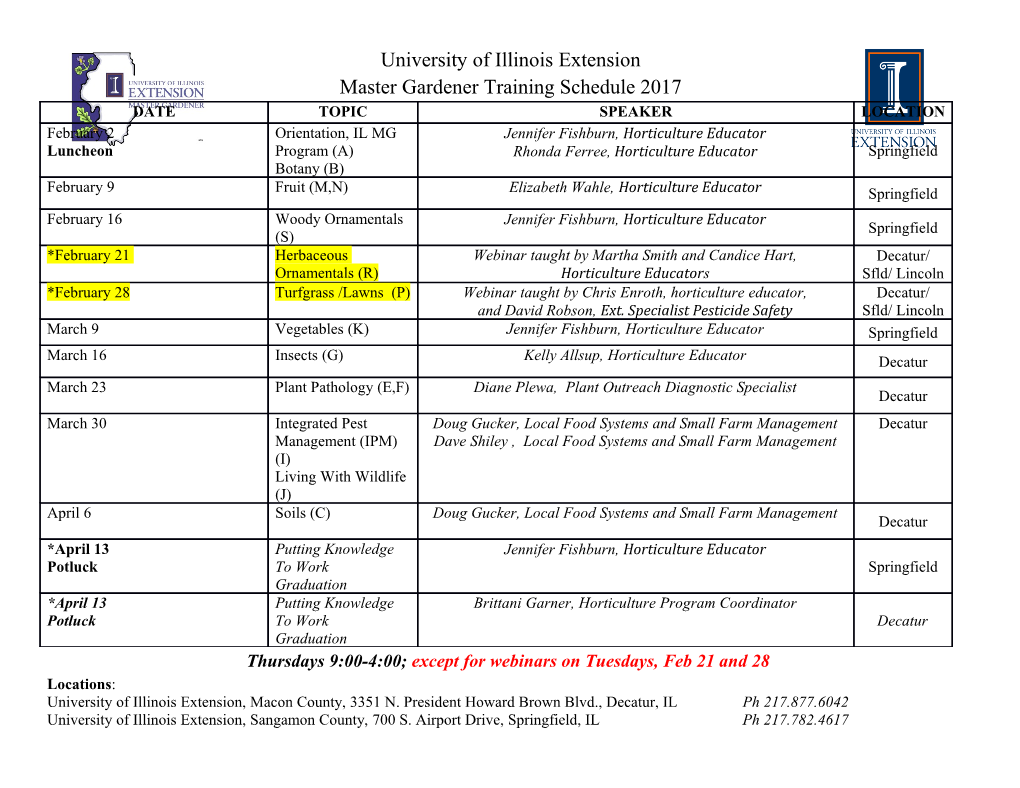
Iowa State University Capstones, Theses and Retrospective Theses and Dissertations Dissertations 1968 Analysis and prediction of crop yields for agricultural policy purposes Richard Kidd Perrin Iowa State University Follow this and additional works at: https://lib.dr.iastate.edu/rtd Part of the Agricultural and Resource Economics Commons, and the Agricultural Economics Commons Recommended Citation Perrin, Richard Kidd, "Analysis and prediction of crop yields for agricultural policy purposes" (1968). Retrospective Theses and Dissertations. 3689. https://lib.dr.iastate.edu/rtd/3689 This Dissertation is brought to you for free and open access by the Iowa State University Capstones, Theses and Dissertations at Iowa State University Digital Repository. It has been accepted for inclusion in Retrospective Theses and Dissertations by an authorized administrator of Iowa State University Digital Repository. For more information, please contact [email protected]. This dissertation has been microiihned exactly as received 6 8-14,814 PEKRIN, Bichard Kidd, 1937- ANALYSIS AND PREDICTION OF CROP YIELDS FOR AGRICULTURAL POLICY PURPOSES. Iowa State University, Pb.D., 1968 Economics, agricultural University Microfilms, Inc., Ann Arbor, Michigan ANALYSIS #D PREDICTION CE CROP YIELDS FOR AGRICUIiTURÂI. POLICY PURPOSES by Richard Kidd Perrin A Dissertation Submitted to the Graduate Faculty in Partial Fulfillment of The Requirements for the Degree of DOCTOR CF HIILOSOHIY Major Subject; Agricultural Economics Approved Signature was redacted for privacy. Ina ChareeCharge of MaiorMajor Work Signature was redacted for privacy. Head of Major Department Signature was redacted for privacy. Dean Iowa State University Of Science and Technology Ames, Iowa 1968 ii TABLE CP cmTENTS Page I. INTRODUCTim AND OBJECTIVES I II. FRAMEWORK FOR ANALYSIS, PREDICTION AND POLICY DECISIONS 4 III. WEATHER AND CROP YIELDS 42 IV. STATISTICAL ESTIMATION PROCEDURES 64 V. ESTIMATION OF DATA FOR THE STUDY 84 VI. ESTIMATES GF PRODUCTIF FUNCTIONS 109 VII. STOCHASTIC YIELD FORECASTS 150 VIII. EVALUATION <F POLICY IMPLICATIONS 169 IX, CONCLUSIONS AND LIMITATIONS CF THE STUDY 189 X. LITERATURE CITED 197 XI. ACKNOWLEDGEMENTS 205 XII. APPENDIX 206 1 I. INTRODUCTION âND OBJECTIVES Frederick V. Waugh (111) has quoted-the January 27, 1967, issue of the Washington Post as follows. "Economic policy is in essence an endeavor to grapple with the persistent uncertainties of the future, to utilize knowledge effectively in achieving the conflicting goals of national welfare," This statement is as true for agricultural policy as for economic policy in general. The purpose of this study is to bring quantitative economic tools to bear on the analysis and prediction of crop yields as they are related to agricultural policy. The thesis of this study is that such tools, by increasing our knowledge of aggregate crop yields, can reduce the "persistent uncertainties of the future" and thus lead to more informed policy decisions. To demonstrate the usefulness of yield predictions so derived, simple policy models will be developed and applied. The success or failure of any farm program depends in large part on the accuracy of aggregate yield predictions made a year and more in advance. It is apparent that the trend in the yields of most crops has been sharply upward in recent years, but considerable controversy exists regarding the relative contributions of various technological improvements and weather to these yield increases, An objective yield prediction procedure requires not only an evalua­ tion of the relative effects of these factors on yields, but also a projection of the future levels which each factor may be expected to assume. Errors in the projection of these levels impart a random element into any yield forecasting procedure, and the prediction of 2 weather in the years ahead is particularly subject to such error. This study will attempt to quantify past weather and technological factors, analyze their effects on yields, predict future crop yields based on projected levels of these factors and will also derive estimates of the parameters of the random element associated with these yield predictions. The objectives of this study may therefore be outlined as follows. 1. To obtain estimates of aggregate physical production functions for the yields of various crops in specified states, considering various technological factors and a newly developed meterological weather index as inputs. 2. To obtain stochastic yield projections through 1971, based upon the estimated production functions and projected inputs, with weather as a stochastic input. 3. To derive simple decision models to demonstrate the useful­ ness of the stochastic yield projections in meeting specified agri­ cultural policy goals. This study is a pilot study in that the crops and states con­ sidered are limited to com in Illinois and Iowa, grain sorghum in Kansas and Nebraska and wheat in Kansas, Nebraska and North Dakota, Time-series data on past yields will be regressed on observed weather data, fertilizer application rates and other technological inputs to provide estimates of yield production functions. Estimates of the errors associated with weather predictions will provide a basis for approximating probable errors associated with yield predictions as 3 derived from these production functions. Should the results of the present study warrant more comprehensive studies of a similar nature, the procedures developed and the results obtained will provide useful guidelines for such future analyses. 4 II. FRAMEWORK FOR ANALYSIS, PREDICTION AND. POLICY DECISIONS The three primary objectives of this study relate to the estima­ tion of aggregate physical production functions for crop yields, the derivation of stochastic yield projections based on these estimates and the development and application of simple decision models incor­ porating the projections so derived. The necessary theoretical framework for these objectives is developed in this section, Ao Aggregate Physical Production Functions 1. The nature of aggregate physical production functions Production functions are mathematical equations representing the transformation of one set of goods (factors of production) into another set of goods (products). As with other economic models, the usefulness of production functions derives from the fact that they are abstractions from reality and as such are more comprehensible. In the abstraction process, a large number of factors are ignored, leaving a residual portion of production accounted for by the combined affects of the ignored factors. The smaller this residual, in general, the more useful is the model in representing the production process. Frequently one of the factors is of special concern, and the product or output may be arbitrarily measured in terms of average output per unit input of that factor. In this study, output per acre of land is expressed _as a function of per acre inputs such as fertilizer application and moisture availability. 5 Aggregate production functions represent the relationship between output and inputs summed over several producing units. The selection of the producing unit is somevdiat arbitrary and related to the purpose of the investigation. A producing unit may be con­ sidered to be one square foot of soil, so that a function expressing yield per acre as a function of per acre inputs could properly be considered an aggregate production function. Generally in the literature, however, aggregate studies refer to aggregations over firms, and in this study we aggregate over all farm firms within specified regions. It is important to stress at this point that the level of aggregation affects to a considerable degree the nature of the production function which can adequately represent the production process. The following example will serve to illustrate. Suppose we let the function Y = a + bX represent the transformation of applied nitrogen into bushels of corn when the nitrogen is distributed evenly over one acre of land. This relationship implies either that all other factors of production are held constant or that there are no other significant factors which need to be taken into account. We know, however, that there will be marked differences in soil structure, soil fertility and possibly micro-climate within the acre of land being considered. As a result, we would expect the "aggregate" function Y = a + bX to differ from any of the functions representing the production process on, say, a particular square yard of land within that acre. It may further be the case that, due to soil 6 structure, yield on one particular square yard may be sensitive to canpaction, while for the acre as a whole this "factor" may be ignored as relatively unimportant. Analogously, we might find that factors which are very significant in explaining yield on a particular field can be ignored in describing the average production on all fields in a state or district. It should be clear then that an aggregate function describing the average production process in a region may be of little relevance in describing the production process on a particular farm or a particular field, and vice-versa. Historically, aggregate production functions have generally been formulated to express the relationship between the dollar value of various factors and the dollar value of output. The intent of this study, however, is to formulate production functions describing the transformation of physically measured inputs into physically measured
Details
-
File Typepdf
-
Upload Time-
-
Content LanguagesEnglish
-
Upload UserAnonymous/Not logged-in
-
File Pages236 Page
-
File Size-