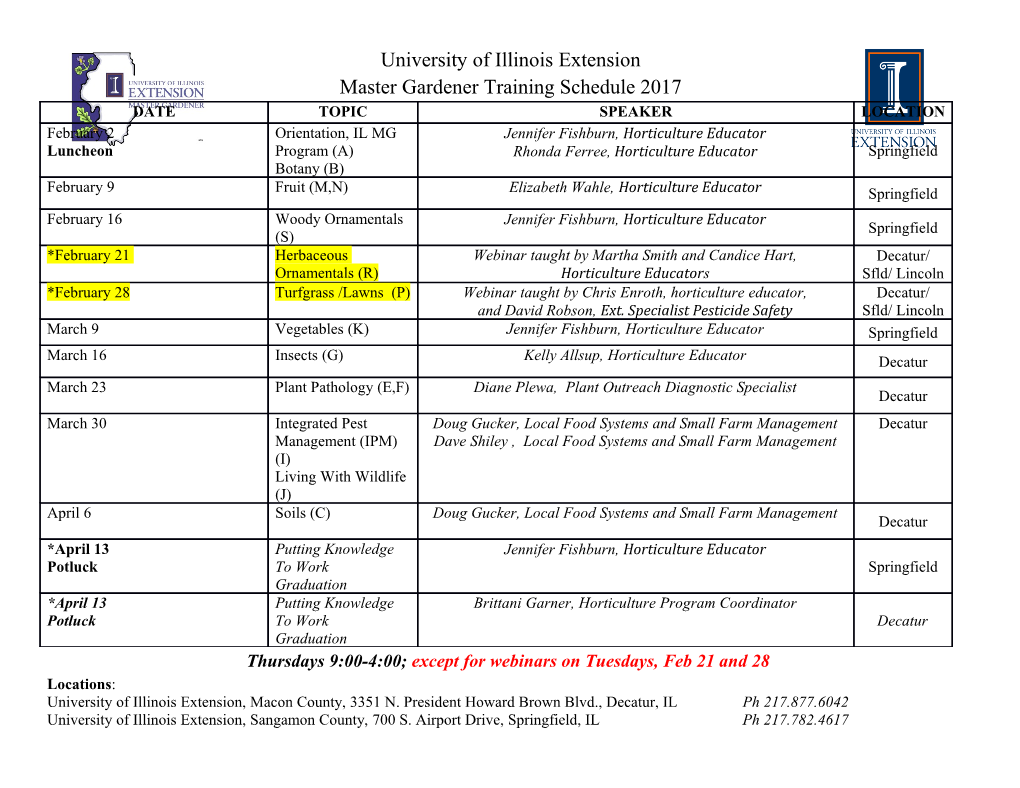
“You Can’t Sit With Us”: Exclusionary Pedagogy in AI Ethics Education Inioluwa Deborah Raji Morgan Klaus Scheuerman Razvan Amironesei Mozilla Foundation Information Science, University of CADE, University of San Francisco [email protected] Colorado Boulder [email protected] [email protected] ABSTRACT the call to add ethics to CS curricula [28], there has been an ongoing Given a growing concern about the lack of ethical consideration call to “make engineers ethical.” in the Artificial Intelligence (AI) field, many have begun toques- However, there have also been noted observations of the failure tion how dominant approaches to the disciplinary education of of the approach of inserting ethics education into CS curriculum— computer science (CS)—and its implications for AI—has led to the either referenced throughout or in a standalone course—as a lim- current “ethics crisis”. However, we claim that the current AI ethics ited intervention for improving the outcomes of the discipline [9]. education space relies on a form of “exclusionary pedagogy,” where Although an important step towards informing more socially con- ethics is distilled for computational approaches, but there is no scious system builders, it is becoming clear that proposals anchored deeper epistemological engagement with other ways of knowing to developing individual morality and understanding falls short of that would benefit ethical thinking or an acknowledgement ofthe resulting in any noticeable changes to the way in which students limitations of uni-vocal computational thinking. This results in conduct research and develop applications for deployment once indifference, devaluation, and a lack of mutual support between they leave the classroom [35]. This is made even more evident by CS and humanistic social science (HSS), elevating the myth of the consistency with which such crises continue to occur [81]. technologists as "ethical unicorns" that can do it all, though their Incidents of algorithmic misuse, unethical deployments, or harm- disciplinary tools are ultimately limited. Through an analysis of ful bias cannot be addressed by developing moral integrity at an computer science education literature and a review of college-level individual level. We argue that this is because the individual scope course syllabi in AI ethics, we discuss the limitations of the episte- of current educational approaches neglects the fact that the cur- mological assumptions and hierarchies of knowledge which dictate rent issues are more likely the result of collective failure, and more current attempts at including ethics education in CS training and institutionalized practices accepted within the field of computer explore evidence for the practical mechanisms through which this science, rather than moments of individual judgement. In fact, such exclusion occurs. We then propose a shift towards a substantively challenges are inherently interdisciplinary, requiring the cooper- collaborative, holistic, and ethically generative pedagogy in AI edu- ation of stakeholders of varying expertise in business, law, and cation. other domains in order to meaningfully address in the real world [23]. If anything, to rush CS students through heavily condensed ACM Reference Format: and simplified overviews of broad ethical understanding and then Inioluwa Deborah Raji, Morgan Klaus Scheuerman, and Razvan Amironesei. position them to be the primary arbiter of change confuses the 2021. “You Can’t Sit With Us”: Exclusionary Pedagogy in AI Ethics Education. situation. This promotes the engineer’s natural inclination towards In ACM Conference on Fairness, Accountability, and Transparency (FAccT seeing themselves as a solitary saviour, to the detriment of the ’21), March 3–10, 2021, Virtual Event, Canada. ACM, New York, NY, USA, 11 pages. https://doi.org/10.1145/3442188.3445914 quality of the solution and in spite of the need for other disciplinary perspectives. Therefore, in order to address the social impact of technical 1 INTRODUCTION systems, including AI, we need to revisit the way we think about Over the last few years, alongside the rise of public scrutiny of the norms of AI ethics education, and in particular address the the role of artificial intelligence (AI) in reifying and amplifying tendency towards an “exclusionary” pedagogy, that further siloes social inequalities, machine learning educators have begun to ac- CS perspectives to challenges from the necessary consideration of knowledge the necessity of an ethics curricula in computer science other approaches. This is a required first step towards the genuine programs. Khari Johnson of VentureBeat called it a “fight for the interdisciplinary collaboration necessary to meaningfully address soul of machine learning” [44]. From the ACM ethics charter [2], to the ethical issues that continue to arise. The way in which we teach AI ethics informs the way in which practitioners are trained and re- Permission to make digital or hard copies of all or part of this work for personal or flects academic practice. Rather than exploring strategies to retrain classroom use is granted without fee provided that copies are not made or distributed AI scholars or practitioners—exposing them to a sprinkle of ethics for profit or commercial advantage and that copies bear this notice and the full citation and social science, and centering interventions on how to incor- on the first page. Copyrights for components of this work owned by others than ACM must be honored. Abstracting with credit is permitted. To copy otherwise, or republish, porate social considerations into technical expertise—we instead to post on servers or to redistribute to lists, requires prior specific permission and/or a discuss the need to think more deeply about what it would mean to fee. Request permissions from [email protected]. reset the pedagogy and practices of the field to shift away from this FAccT ’21, March 3–10, 2021, Virtual Event, Canada © 2021 Association for Computing Machinery. exclusionary default. Through a systematic analysis of over 100 AI ACM ISBN 978-1-4503-8309-7/21/03. ethics syllabi, we map the current situation and characterize some https://doi.org/10.1145/3442188.3445914 FAccT ’21, March 3–10, 2021, Virtual Event, Canada Inioluwa Deborah Raji, Morgan Klaus Scheuerman, and Razvan Amironesei suggestions for an educational reset towards a more collaborative matter may have changed since these studies, this snapshot in time— pedagogy, with hopefully more direct consequences on improving before a more recent push for ethics education in computer science both industry practice and academic norms. —highlights a longstanding disciplinary norm: learning computer science has traditionally emphasized mathematical theory and en- 2 HOW COMPUTER SCIENCE PEDAGOGY gineering practices. A focus on “programming intelligence” is a LED US TO THE ETHICS “CRISIS” well-researched cause of the high dropout rates of computer science undergraduate programs [5, 30, 36, 75]. Computer science is what We first examine the literature on how the culture of computer Clark labels “a hard-applied discipline” [14]. Those who approach science has led to the current state of ethics discussions in the computer science problems differently are pushed out of the field, field, focusing specifically on computer science education research, resulting in more homogeneous mindsets and practices within the epistemological analyses of computer science, and empirical stud- discipline and unsurprising diversity deficits [49]. ies of computer science classrooms and cultures. We then discuss An imbalance between the “technical” and the “social”—the current issues in tech ethics, primarily its insular focus on techno- “hard” and the “soft”—which prioritizes the former, has not gone solutionism that continues to prioritize computer science expertise unnoticed by other scholars. In an ethnographic study of machine and center the system itself in ethical fixes, as well as a promotion learning practitioners in industry, Forsythe witnessed the valu- of the ideal of ethical unicorns or tech saviours, ie. technologists ing of computer science skills, the devaluing of user needs, and with shallow socio-technical understanding intent on playing the the belittling of women’s work (commonly characterized as social primary role in delivering complete solutions. and soft) [33]. She posited that social science perspectives would improve AI by acting as a counter weight to address what she 2.1 Historical Retrospective on CS Education called an epistemological imbalance, though this was a view that Norms AI researchers actively resisted at the time [31]. Later on, Wagstaff In starting with computer science as a discipline, broadly, there is wrote that machine learning researchers, in their lack of training a heavy focus on what Eden identifies as three paradigms: tech- in understanding social contexts, often fail to create models that nocratic, rationalist, and scientific [24]. At its simplest, the tech- have real world applicability or merit; among many suggestions to nocratic paradigm might be described as an engineering or pro- remedy this failure, Wagstaff suggests the improved interaction and grammatic approach, which centers the skills to build computer involvement with “the outside world” when creating models [76]. programs; the rationalist paradigm might be described as a mathe- In a scathing analysis of the technocratic dominance in computer matically theoretical approach, focusing more heavily
Details
-
File Typepdf
-
Upload Time-
-
Content LanguagesEnglish
-
Upload UserAnonymous/Not logged-in
-
File Pages11 Page
-
File Size-