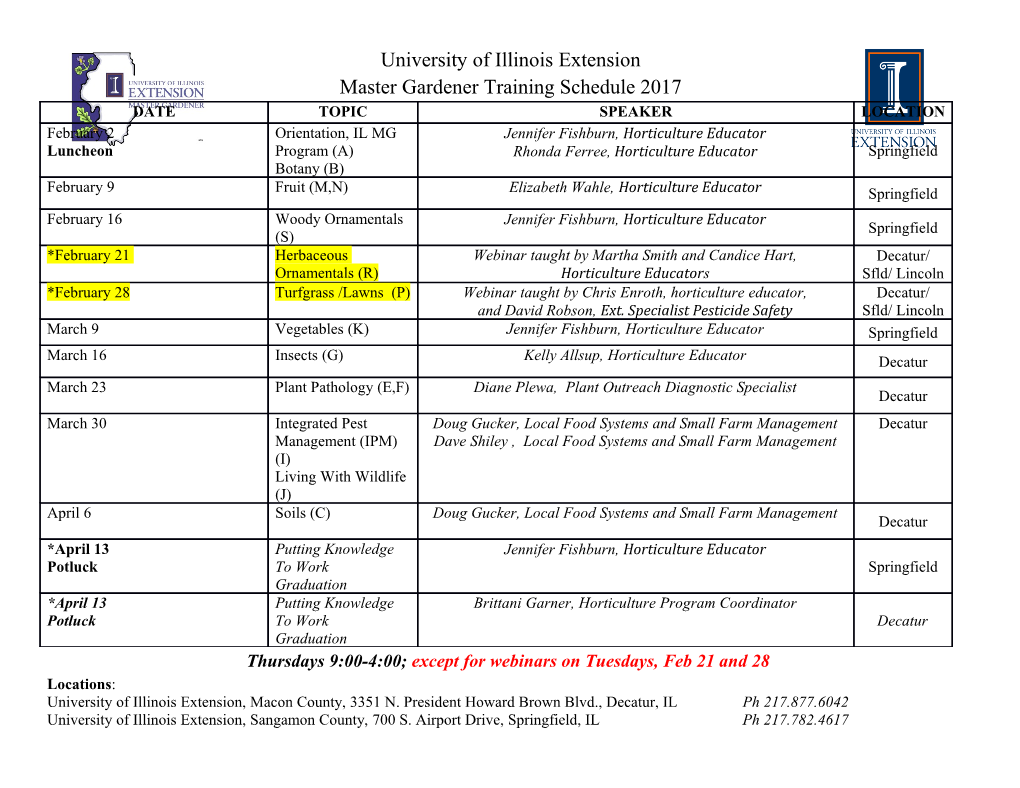
Journal of Marine Systems 139 (2014) 261–275 Contents lists available at ScienceDirect Journal of Marine Systems journal homepage: www.elsevier.com/locate/jmarsys Modelling dinoflagellates as an approach to the seasonal forecasting of bioluminescence in the North Atlantic Charlotte L.J. Marcinko a,b,⁎, Adrian P. Martin b,JohnT.Allenc a Ocean and Earth Sciences, University of Southampton, University of Southampton Waterfront Campus, Southampton SO14 3ZH, UK b National Oceanography Centre, European Way, Southampton SO14 3ZH, UK c School of Earth and Environmental Sciences, University of Portsmouth, Burnaby Building, Burnaby Road, Portsmouth, Hampshire PO1 3QL, UK article info abstract Article history: Bioluminescence within ocean surface waters is of significant interest because it can enhance the study of subsur- Received 30 January 2014 face movement and organisms. Little is known about how bioluminescence potential (BPOT) varies spatially and Received in revised form 19 May 2014 temporally in the open ocean. However, light emitted from dinoflagellates often dominates the stimulated biolu- Accepted 30 June 2014 minescence field. As a first step towards forecasting surface ocean bioluminescence in the open ocean, a simple Available online 6 July 2014 ecological model is developed which simulates seasonal changes in dinoflagellate abundance. How forecasting seasonal changes in BPOT may be achieved through combining such a model with relationships derived from Keywords: Bioluminescence observations is discussed and an example is given. The study illustrates a potential new approach to forecasting Dinoflagellate BPOT through explicitly modelling the population dynamics of a prolific bioluminescent phylum. The model Ecosystem developed here offers a promising platform for the future operational forecasting of the broad temporal changes Model in bioluminescence within the North Atlantic. Such forecasting of seasonal patterns could provide valuable infor- Forecast mation for the targeting of scientific field campaigns. Atlantic © 2014 Natural Environment Research Council (NERC). Published by Elsevier B.V. This is an open access article under the CC BY license (http://creativecommons.org/licenses/by/3.0/). 1. Introduction 2000 km) or forecasting changes in its spatial distribution on time scales of the order of days (Ondercin et al., 1995; Shulman et al., 2003, 2011, Many marine organisms are capable of producing visible light, known 2012). Models have been based upon empirical relationships between as bioluminescence (Haddock et al., 2010; Widder, 2010). Biolumines- bioluminescence and other biogeochemical environmental variables cence is ubiquitous throughout the world's oceans and in certain condi- such as chlorophyll (Ondercin et al, 1995) or the advancement of the tions, highly visible displays of blue light (474–476 nm) can be bioluminescence field through physical advective and diffusive process- stimulated via hydrodynamic stresses induced by surface and sub- es (Shulman et al, 2003, 2011). These models have had limited success surface movement (e.g. Fig. 1). In situ measurement of bioluminescence in simulating the distribution of the bioluminescence field (reviewed offers a number of applications for assessing the wider ocean environ- in Marcinko et al., 2013a). The lack of success indicates that the distribu- ment (Herring and Widder, 2001; Moline et al., 2007). This includes the tion of bioluminescent organisms will not always coincide with that of study of subsurface physical dynamics such as internal waves (Kushnir the phytoplankton dominating the chlorophyll signal or be correlated et al., 1997) and the evaluation of fish stocks using intensified cameras with physical properties. The ecological and behavioural dynamics of at night to identify fish schools (Churnside et al., 2012; Squire and the bioluminescent organisms themselves must be considered for the Krumboltz, 1981). Thus, the ability to forecast its occurrence in surface forecasting of bioluminescence to be improved (Shulman et al., 2012). waters and to assess when the illumination of near-surface motion may Bathyphotometer measurements of flow stimulated biolumines- occur is of significant interest. cence provide a measure of the bioluminescent potential (BPOT) of a Previous attempts to model bioluminescence have focused upon volume of water. Field measurements indicate that dinoflagellates are simulating its distribution over relatively large spatial scales (1000– a major source of bioluminescence and that their light emissions often dominate the BPOT measured in the surface ocean (Batchelder and Swift, 1989; Lapota et al., 1992; Latz and Rohr, 2005; Neilson et al., 1995; Seliger et al., 1962). Although only a small proportion of dinofla- gellates are known to be bioluminescent (around 70 out of approxi- ⁎ Corresponding author. Tel.: +44 2380 596338. E-mail addresses: [email protected] (C.L.J. Marcinko), [email protected] mately 1500 species) a number of studies have found positive (A.P. Martin), [email protected] (J.T. Allen). associations between BPOT and total dinoflagellate cell abundance (i.e. http://dx.doi.org/10.1016/j.jmarsys.2014.06.014 0924-7963/© 2014 Natural Environment Research Council (NERC). Published by Elsevier B.V. This is an open access article under the CC BY license (http://creativecommons.org/licenses/by/ 3.0/). 262 C.L.J. Marcinko et al. / Journal of Marine Systems 139 (2014) 261–275 majority of BPOT stimulated in the surface oceans. As part of the LAMP project, this study describes the development, parameterisation and testing of an ecosystem model which simulates broad temporal changes in dinoflagellate abundance within an open ocean region of the North- east Atlantic. The study aims to illustrate how such a model could pro- vide a platform for the future operational forecasting of surface BPOT within the North Atlantic. We take a purposefully simplistic modelling approach combined with the limited available bioluminescence data to conceptualise how the forecasting of surface BPOT could be advanced in the future. 2. Study area Fig. 1. Dolphins illuminated by dinoflagellate bioluminescence at night as they swim The model aims to simulate a typical annual cycle (for the years be- through the ocean (provided by Ammonite Films). tween 2001 and 2007) within the waters surrounding the Porcupine Abyssal Plain sustained observatory; the black box shown in Fig. 2 based on the standard area E5 of the Continuous Plankton Recorder Sur- bioluminescent plus non-bioluminescent species). For instance, within vey (CPR). This region is characterised by a large spring phytoplankton the Mediterranean and Black Seas a compilation of over 3500 bloom (Ducklow and Harris, 1993) dominated by diatoms (Barlow et al., bioluminescent measurements and 1000 phytoplankton samples 1993; Lochte et al., 1993). The spring bloom is followed by the prolifer- between 1970 and 1995 showed extremely strong positive relation- ation of other organisms (Barlow et al., 1993; Lochte et al., 1993) and ships (r2 = 0.95 to 0.98; p b .05) between BPOT and dinoflagellate cell observations show that dinoflagellates contribute significantly to the abundance (Tokarev et al., 1999). Similarly, positive correlations (r = post bloom summer phytoplankton population (Dodge, 1993; Leterme 0.86–0.93; p b .05) have been found in the mouth of the Vestfjord, et al., 2005; Smythe-Wright et al., 2010). The study region was chosen Norway (Lapota et al., 1989) and the MLML programme, which con- to coincide with previous LAMP experiments (Marcinko et al., 2013b) ducted several cruises in the Irminger Sea between 1989 and 1991 and because of the availability of data required for model optimisation (Marra, 1995), found dinoflagellates were responsible for N90% of and assessment. BPOT throughout spring, summer and autumn (Swift et al., 1995). The findings of the studies described above suggest that temporal changes in dinoflagellate abundance can be used as a proxy for seasonal 3. Model description variations in BPOT. Therefore, explicitly modelling dinoflagellate popu- lation dynamics could provide a feasible approach to operational fore- A schematic of the model including the flows between state vari- casting of BPOT, where ‘operational forecasting’ is the term widely ables is shown in Fig. 3. The model contains five components used to describe the prediction of a specific event using a practical representing the interactions between nutrients, phytoplankton and means. This approach is particularly promising in non-coastal regions zooplankton. The biological ecosystem is assumed to be homogenously where bioluminescent populations are often mixed and modelling distributed within an upper mixed layer overlying a deep abiotic layer. individual species is not possible. A successful dynamical model incor- Organisms are not modelled in the deeper layer as the study is focused porating a ‘dinoflagellate’ functional group will potentially have the ca- upon interactions within the surface waters. The system is composed of pability to reproduce much of the seasonal and large scale spatial two phytoplankton types, diatoms (P1)anddinoflagellates (P2), two nu- variation of the bioluminescence field (Marcinko et al., 2013a). trients, nitrate (N) and silicate (Si), and one predator type, metazoan The modelling of dinoflagellates has largely focused on Harmful zooplankton (Z). Algal Bloom (HAB) species in specific coastal areas (Anderson, 1998; The model provides a simple structure that captures the primary in- Franks, 1997; Montagnes
Details
-
File Typepdf
-
Upload Time-
-
Content LanguagesEnglish
-
Upload UserAnonymous/Not logged-in
-
File Pages15 Page
-
File Size-