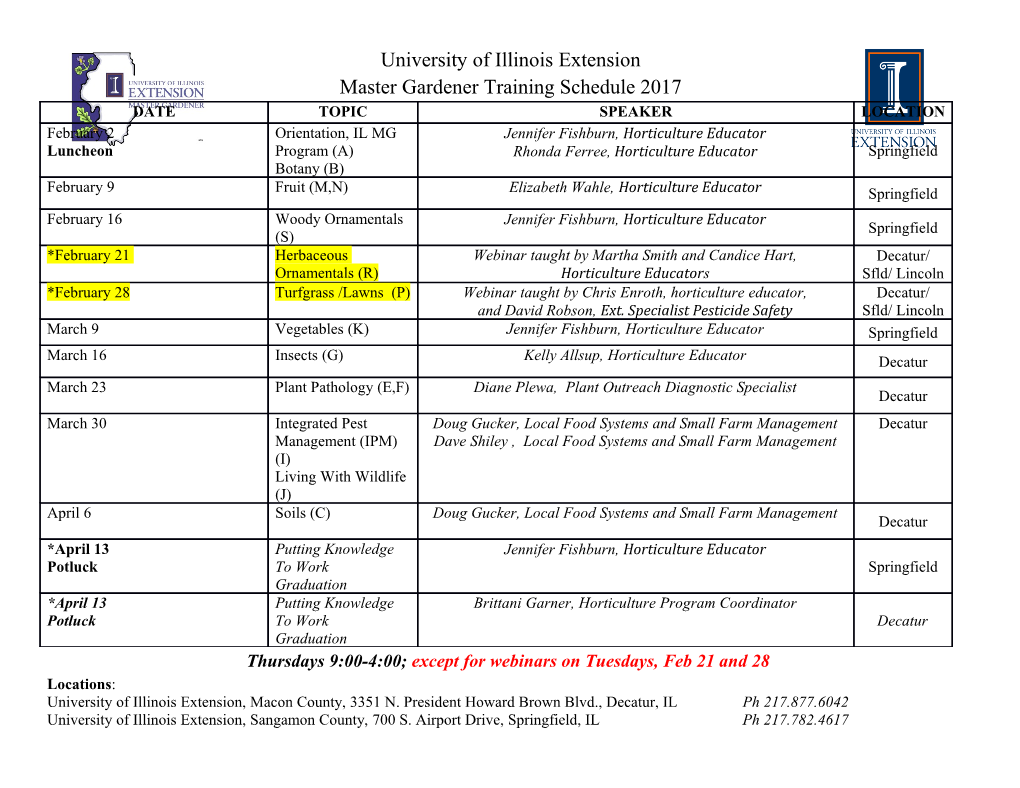
Nanophotonics 2020; 9(7): 1579–1599 Review article Xuewei Feng, Xinke Liu* and Kah-Wee Ang* 2D photonic memristor beyond graphene: progress and prospects https://doi.org/10.1515/nanoph-2019-0543 Received December 24, 2019; revised February 3, 2020; accepted 1 Introduction February 4, 2020 With the emergence of the Internet-of-Things (IoT), the Abstract: Photonic computing and neuromorphic com- vast increase in the amount of data workloads creates puting are attracting tremendous interests in breaking new challenges in the need to improve computation speed the memory wall of traditional von Neumann architec- and energy efficiency. However, the instructions transfer ture. Photonic memristors equipped with light sensing, latency between processor and memory that put on a fun- data storage, and information processing capabilities are damental limitation for modern computers known as the important building blocks of optical neural network. In memory wall [1]. In order to break the memory wall, one the recent years, two-dimensional materials (2DMs) have revolutionary approach is, to make use of the neuromor- been widely investigated for photonic memristor applica- phic computing with resistive switching memories (mem- tions, which offer additional advantages in geometry scal- ristors) that aims to carry out calculations in situ where ing and distinct applications in terms of wide detectable the data are located [2–8]. The memristor, a combined spectrum range and abundant structural designs. Herein, word of memory and resistor, is a non-volatile memory the recent progress made toward the exploitation of 2DMs whose resistance state could be programmed by the beyond graphene for photonic memristors applications applied voltage it has experienced [2, 5]. This approach are reviewed, as well as their application in photonic is similar to the computing scheme in the human brain, synapse and pattern recognition. Different materials and where information is processed in biological neural net- device structures are discussed in terms of their light tune- works (BNNs) of neurons and synapses, without any able memory behavior and underlying resistive switching physical separation between computation and memory mechanism. Following the discussion and classification [3, 7]. By building the memristors into large-scale cross- on the device performances and mechanisms, the chal- bar arrays to form artificial neural network (ANN), they lenges facing this rapidly progressing research field are can perform efficient neuromorphic computing to enable discussed, and routes to realize commercially viable 2DMs artificial intelligence functions, such as pattern storage photonic memristors are proposed. and image recognition [8]. However, for a single-layer neural network, in Keywords: photonic memristor; neuromorphic computing; general, the number of artificial synapses is the square photonic synapse. of the number of artificial neurons. For example, a fully interconnected network of 104 neurons requires 108 syn- apses, which is approaching the limit of the state-of-the- art VLSI technology. The interplay between photonics and ANNs offers the advantages of parallel processing and massive interconnection capabilities for the design *Corresponding authors: Xinke Liu, College of Materials Science of large-scale photonic neuromorphic computers and Engineering, Shenzhen University, 3688 Nanhai Ave, Shenzhen 518060, P.R. China, e-mail: [email protected]. [9–12]. Because light beams can pass through a three-­ https://orcid.org/0000-0002-3472-5945; and Kah-Wee Ang, dimensional space without interfering with each other, Department of Electrical and Computer Engineering, National optical systems can provide higher space-bandwidth- University of Singapore, 4 Engineering Drive 3, Singapore 117583, product operation and ultrafast propagation speed than Singapore, e-mail: [email protected]. https://orcid.org/0000- their electronic counterparts. These unique properties of 0003-1919-3351 Xuewei Feng: Department of Electrical and Computer Engineering, optics have stimulated great interest for designing pho- National University of Singapore, 4 Engineering Drive 3, Singapore tonic ANNs, which can perform neuromorphic functions 117583, Singapore. https://orcid.org/0000-0002-1463-8270 at high speed. Moreover, combining photonic stimuli Open Access. © 2020 Xinke Liu and Kah-Wee Ang et al., published by De Gruyter. This work is licensed under the Creative Commons Attribution 4.0 Public License. 1580 X. Feng et al.: 2D photonic memristor: progress and prospects with electronic ones in synaptic devices can enable the integration density. The three-terminal lateral photonic integration of synaptic functions with biometric sensing memristor typically exploits the photo-generated charge elements such as vision, auditory, and olfactory sensors trapping/detrapping on the floating gate, which provides [13–15]. more design flexibility and new functionality. Beyond The photonic ANNs put a new requirement to the this device-level emulation of synaptic dynamics, these optoelectronic devices and resistive switching materials 2DMs photonic synaptic devices have also been used to that can store reasonable amount of data while combining build photonic ANNs. Subsequently, pattern recognition with synaptic functions to realize in-memory compute. tasks have been verified by these ANNs, where image pre- Recently, two-dimensional materials (2DMs) have processing and colour-mixed pattern classification are emerged as resistive switching materials, which provide applied [13, 14]. a feasible way to fabricate ultrathin memristive synapses Here, we have reviewed the progress in this fast-­ [16–23]. 2DMs typically refer to crystalline materials con- evolving area that has been achieved over the past few sisting of a single layer of atoms, including semimetallic years, and highlighted the issues that need to be addressed graphene, insulating hexagonal boron nitride (h-BN), toward their practical application for future photonic semiconducting transition metal dichalcogenides (TMDs), ANNs. In the following sections, we have divided the black phosphorus (BP) and group IV mono-chalcogenides realization of photonic memory into two different configu- (e.g. SnS2, SnSe, GeSe and GeS) etc. [24]. The atomic thin- rations, i.e. vertical and lateral structures. Both configura- ness of 2DMs further allows for device miniaturization, tions will be dealt with separately, with a highlight on the high-density crossbar array realization, and synergistic switching mechanism and device performance built from heterostructured devices through van de Waals coupling 2DMs. Finally, the challenges facing this rapidly progress- [25–27]. Nowadays, most of the reported neuromorphic ing research field are discussed and routes to realize com- devices with synaptic functions use electrical signals mercially viable photonic ANNs are proposed. With our to stimulate the device. However, recent studies reveal focus on photonic memristors in 2DMs, we recommend that these 2DMs and their hybrid heterostructures are an readers to refer to some important reviews [8, 10, 46–60] ideal platform for non-volatile photonic memory owing to in the field for some basic understanding of memristors, their strong light-matter interactions and significant pho- electronic, and photonic synaptic devices based on a togenerated charge trapping derived from their very large hybrid of materials systems including 2DMs, organic and surface-to-volume ratio [13, 14, 28–44]. Moreover, the transition metal oxides (TMOs), and the application on abundant material types cover a broad electromagnetic ANNs and neuromorphic computing. spectrum from ultraviolet to infrared, thus 2DMs based photonic memristor shows broad applications, such as image sensors for artificial vision [14], light-gated memris- tor for logic operation [45], and photonic neural network 2 2DMs preparation methods for neuromorphic systems [13]. Owing to the exceptional potential in optoelectronic The growth of large-area, high-quality 2DMs is perhaps one promised by the new class of 2D materials, considerable of the most challenging tasks. In general, the production efforts have been made to demonstrate and realize their methods can be classified either as top-down, where bulk applications in photonic memory and photonic synapse. layered materials are directly exfoliated to yield mono- Beyond electronic stimuli, in novel 2DMs based photonic and few layer flakes or bottom-up, where individual flakes memristors and synaptic devices, either light signal or are grown or synthesized on substrates [61, 62]. The most gate voltage is typically used for the programming/erasing common methods of top-down synthesis are mechanical operation, and electric source–drain voltage is used for exfoliation and chemical exfoliation such as solution pro- read-out current. The synaptic weight is encoded into cessing; as for bottom-up approach, the most commonly the conductance of the 2DMs channel. Up to now, both used method is chemical vapor deposition (CVD). the two-terminal photonic memristor and three-terminal CVD is a promising method to realize large scale floating gate photonic memory have been extensively growth [24, 63, 64]. The synthesis process depends on the studied mainly based on molybdenum disulphide (MoS2), properties of substrate, the temperature and the atomic tungsten diselenide (WSe2) and BP. The two-terminal­ gas flux. To prepare 2D TMDs, several CVD techniques vertical memristor, leveraging on the Schottky
Details
-
File Typepdf
-
Upload Time-
-
Content LanguagesEnglish
-
Upload UserAnonymous/Not logged-in
-
File Pages21 Page
-
File Size-