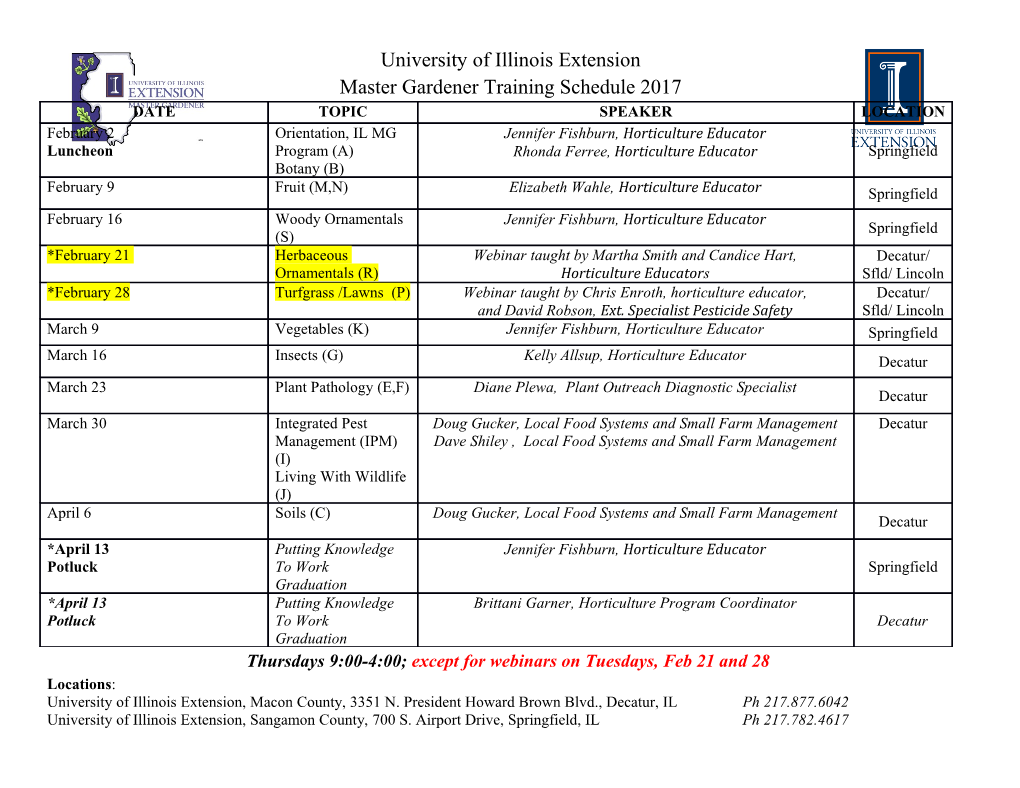
A framework to offer high value manufacturing through self-reconfigurable manufacturing systems Victor Manuel Cedeno-Campos A thesis submitted in partial fulfilment of the requirements for the degree of Doctor of Philosophy The University of Sheffield Faculty of engineering Department of Automatic Control and Systems Engineering August 2016 Abstract The High Value Manufacturing (HVM) sector is vital for developed countries due to the creation of innovative products with advanced technology that cannot be reproduced at the same cost and time with traditional technology. The main challenge for HVM is to rapidly increase production volume from one-off products to low production volume. This requires highly flexible manufacturing systems that can produce new products at variable production volumes. Current manufacturing systems, classified as dedicated, flexible and reconfigurable systems, are limited to produce one type of product(s), within a production volume range and have fixed layouts of machines. Thus, there is a need for highly flexible systems that can rapidly adjust their production volume according to the production demand (i.e. main HVM challenge). Therefore, a novel manufacturing framework, called INTelligent REconfiguration for a raPID production change (INTREPID), is presented in this thesis. INTREPID consists of a user interface and communications platform, a job allocation system, a globally distributed network of Reconfigurable Manufacturing Centres (RMCs), consisting of interconnected factories, and Self- Reconfigurable Manufacturing Systems (S-RMSs). The highly flexible S-RMS consists of movable machines and Mobile Manufacturing Robots (MMRs). The novelty of the S-RMS is its capability of forming layouts bespoke to the current production needs. The vision of INTREPID is to offer global HVM services through the network of RMCs. The job allocation system determines the best possible RMCs or factories to perform a job by considering the complexity of the production requirements and the status of the available S-RMSs at each factory. The planning of the production with S-RMS is challenging due to its high flexibility. The main example of this flexibility is the possibility to create layouts bespoke to current production needs. Yet, this flexibility involves the challenges of determining allocations and schedules of tasks to robots and machines, positions to manufacture, and routes to reach those positions. In manufacturing systems with fixed layouts, production plans are determined by solving a sequence of problems. However, for the S-RMS, it is proposed to determine production plans with a single problem that covers the scheduling, machine layout and vehicle routing problems simultaneously. This novel problem is called the Scheduling, positions Assigning and Routing problem (SAR) problem. In order to determine the best possible production plan(s) for the S-RMS, it is necessary to use optimisation methods. Dozens of elements, characteristics and assumptions from the constituent problems might be included in the formulation of the SAR problem. Elements, characteristics and assumptions can be considered as decision variables on whether to include or not the elements and characteristics and under which assumptions in the formulation. There are two types of decision variables. Fundamental variables are natural to the SAR problem (e.g. manufacturing resources, factory design and operation), whilst auxiliary variables arise from the aim to simplify the formulation of the optimisation problem (i.e. time formulated as discrete or continuous). Due to the large number of decision variables, there might be millions of possible ways to formulate the SAR problem (i.e. the SAR problem space). Some of these variants are intractable to be solved with optimisation methods. Hence, before formulating the SAR problem, it is necessary to select a problem(s) that is realistic to industrial scenarios but solvable with optimisation methods. Existing selection methods work with pairwise comparisons of alternatives. However, for a space of millions of SAR problems, pairwise comparisons are intractable. Hence, in this thesis, a novel Decision Making Methodology (DMM) based on the controlled convergence method is presented. The DMM helps down-selecting one or a few SAR problems from millions of possible SAR problems. The DMM is demonstrated with a case study of the SAR problem and the results show a significant reduction of the reviewed SAR problems and the time to select them. ii Acknowledgements I would like to express my deepest gratitude to my supervisors, Prof. Tony J. Dodd and Dr. Paul Trodden, for all the guidance and patience through meetings and discussions. Tony motivated me to propose my own research topic and to pursue dreams for a fully automated factory, whilst Paul firmly encouraged me to provide a formal definition of this dreamed factory. Moreover, Tony gave me freedom of action, trusted my judgement to propose and follow my own ideas, guided and challenged me whenever necessary. I would like to also express my sincere gratitude to my examiners, Dr. Reza Abdi and Prof. Peter Fleming, for their constructive and insightful comments, as well as their valuable time during the examination process. I dedicate this thesis to my family, specially to my beloved mother and the memory of my beloved grandmother, uncle and aunts. All my gratitude to my friends from Mexico and from around the world for all their support throughout the PhD. People from around the world, with different opinions, with different religious beliefs, and different societies enrich ourselves, our culture and our societies. We should not be afraid of \the different", neither lock ourselves within walls nor se- clude \the others" outside walls. Also, I would like to thank my sponsor, CONACyT, for giving me the great opportunity to study abroad and contribute to the development of science and technology. iii iv Contents Abstract i Acknowledgements iii Contents v List of Figures ix List of Figures in Appendices xii List of Tables xiii List of Tables in Appendices xiv List of Acronyms xv 1 Introduction 1 1.1 Motivation . .2 1.2 Aim and objectives . .6 1.3 Contributions . .7 1.4 Publications . 10 1.5 Outline . 10 2 Literature review 15 2.1 Manufacturing paradigms evolution . 16 2.1.1 Pre-industry and Industry 1.0 . 17 2.1.2 Industry 2.0 . 20 2.1.3 Industry 3.0 . 23 2.1.4 Industry 4.0 . 25 2.2 Manufacturing models, systems and layouts . 28 2.2.1 Manufacturing models . 29 2.2.2 Manufacturing systems . 32 v Contents 2.2.3 Manufacturing layouts . 36 2.2.4 Relationships between models, systems and layouts . 38 2.3 Concluding remarks . 40 3 INTREPID: A comprehensive framework for high value manufac- turing 41 3.1 Key paradigms for INTREPID . 42 3.2 INTREPID framework . 46 3.2.1 INTREPID general description . 46 3.2.2 INTREPID operation . 49 3.2.3 INTREPID challenges . 52 3.3 INTREPID parts . 53 3.3.1 User interface and communication platform . 53 3.3.2 Infrastructure: Flexible production networks and transformable factories . 56 3.3.3 Job allocation system: Network, cluster and factory levels . 60 3.3.4 Highly flexible manufacturing resources . 64 3.4 Concluding remarks . 69 4 The SAR problem: analysis of constituent problems 72 4.1 Defining the SAR problem . 73 4.2 Scheduling . 74 4.2.1 Types of scheduling problems . 76 4.2.2 Scheduling elements and characteristics . 77 4.2.3 Multi robot task allocation, MRTA . 83 4.2.4 MRTA taxonomies . 84 4.2.5 Optimisation objectives . 87 4.3 Positions assigning problem . 89 4.3.1 Machine layout problem . 90 4.3.2 Dynamic layout problems . 91 4.3.3 Machine layout elements and characteristics . 92 4.3.4 Optimisation objectives . 94 4.4 Routing and motion planning problems . 95 4.4.1 Motion planning . 96 4.4.2 Motion planning elements and characteristics . 97 4.4.3 Routing . 98 4.4.4 Types of vehicle routing problems . 99 4.4.5 Routing elements and characteristics . 100 4.4.6 Optimisation objectives . 104 4.5 Concluding remarks . 104 vi Contents 5 The SAR problem: definitions, assumptions and notation 106 5.1 Assumptions that characterise the SAR problem for a single factory 107 5.2 Factory design and operation . 111 5.2.1 Definitions . 111 5.2.2 Notation . 112 5.2.3 Assumptions on factory design and operation . 116 5.3 Production requirements . 117 5.3.1 Definitions . 118 5.3.2 Notation . 119 5.3.3 Assumptions on production requirements . 121 5.4 Manufacturing resources . 121 5.4.1 Definitions . 122 5.4.2 Notation . 124 5.4.3 Assumptions on manufacturing resources . 128 5.5 Complete notation . 128 5.6 Problem formulation as a decision making problem . 133 5.6.1 SAR Problem space . 135 5.7 Concluding remarks . 137 6 A methodology for problem space exploration and selection 139 6.1 Literature review on concept generation and selection . 141 6.1.1 Decision matrix methods . 144 6.1.2 Outranking methods for selection . 148 6.1.3 Challenges with large number of alternatives . 150 6.2 Methodology . 151 6.3 Working principles . 152 6.3.1 Variables collection and weighting . 154 6.3.2 Combination process . 154 6.3.3 Aggregation process . 158 6.3.4 Problem space . 159 6.3.5 Interactive analysis and selection . 162 6.4 Methodology algorithm . 164 6.4.1 Metrics of success . 167 6.5 Methodology implementation . 167 6.6 Concluding remarks . 176 7 A SAR problem case study 178 7.1 DMM step 1: Data collection . 178 7.2 DMM step 2: Variables grouping, hierarchisation and weighting . 180 7.3 DMM step 3: Variables selection . 184 vii Contents 7.4 DMM steps 4, 5 and 6: Variables' options combination and aggre- gation, and SAR problems analysis and selection . 189 7.4.1 Stage 1 . 189 7.4.2 Stage 2 .
Details
-
File Typepdf
-
Upload Time-
-
Content LanguagesEnglish
-
Upload UserAnonymous/Not logged-in
-
File Pages312 Page
-
File Size-