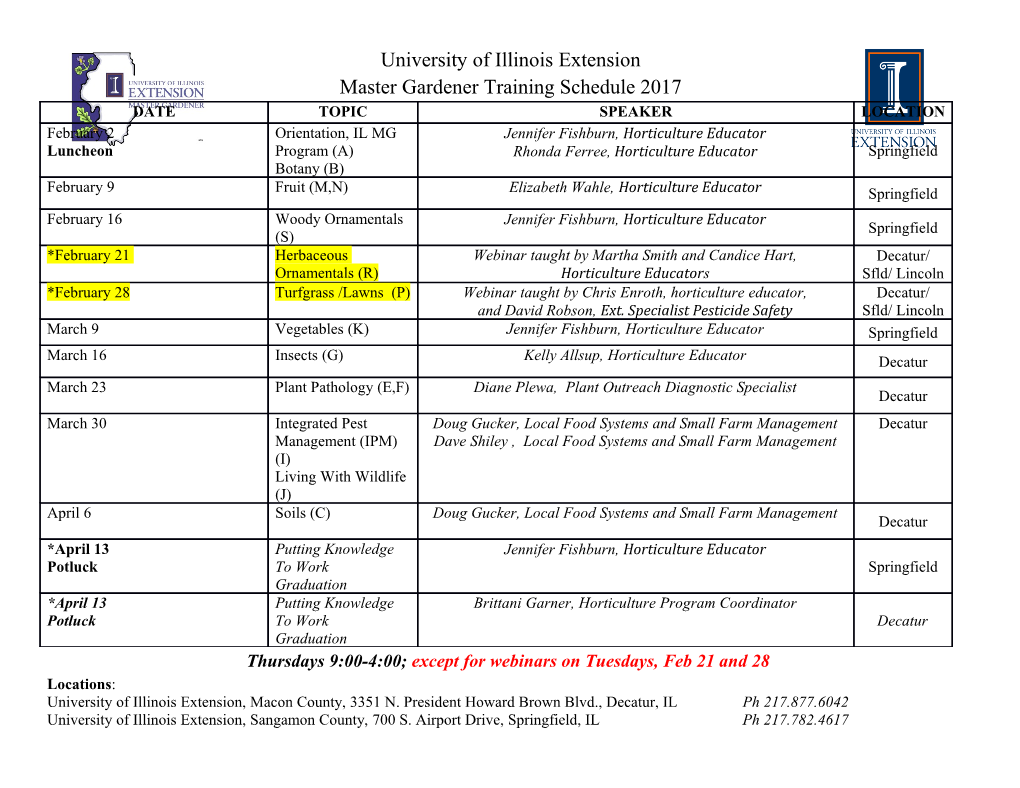
Chemometrics in Spectroscopy Linearity in Calibration: How to Test for Non-linearity Previous methods for linearity testing discussed in this series contain certain shortcom- ings. In this installment, the authors describe a method they believe is superior to others. Howard Mark and Jerome Workman Jr. n the previous installment of “Chemometrics in Spec- instrumental methods have to produce a number, represent- troscopy” (1), we promised we would present a descrip- ing the final answer for that instrument’s quantitative assess- I tion of what we believe is the best way to test for linearity ment of the concentration, and that is the test result from (or non-linearity, depending upon your point of view). In the that instrument. This is a univariate concept to be sure, but first three installments of this column series (1–3) we exam- the same concept that applies to all other analytical methods. ined the Durbin–Watson (DW) statistic along with other Things may change in the future, but this is currently the way methods of testing for non-linearity. We found that while the analytical results are reported and evaluated. So the question Durbin–Watson statistic is a step in the right direction, but we to be answered is, for any given method of analysis: Is the also saw that it had shortcomings, including the fact that it relationship between the instrument readings (test results) could be fooled by data that had the right (or wrong!) charac- and the actual concentration linear? teristics. The method we present here is mathematically This method of determining non-linearity can be viewed sound, more subject to statistical validity testing, based upon from a number of different perspectives, and can be consid- well-known mathematical principles, consists of much higher ered as coming from several sources. One way to view it is as statistical power than DW, and can distinguish different types having a pedigree as a method of numerical analysis (5). of non-linearity from one another. This new method also has Our new method of determining non-linearity (or show- been described recently in the literature (4). ing linearity) also is related to our discussion of derivatives, But let us begin by discussing what we want to test. The particularly when using the Savitzky–Golay method of con- FDA/ICH guidelines, starting from a univariate perspective, volution functions, as we discussed recently (6). This last is considers the relationship between the actual analyte concen- not very surprising, once you consider that the tration and what they generically call the “test result,” a term Savitzky–Golay convolution functions also are (ultimately) that is independent of the technology used to ascertain the derived from considerations of numerical analysis. analyte concentration. This term therefore holds good for In some ways it also bears a resemblance to the current every analytical methodology from manual wet chemistry to method of assessing linearity that the FDA and ICH guidelines the latest high-tech instrument. In the end, even the latest recommend, that of fitting a straight line to the data, as assess- ing the goodness of the fit. As we have shown (2, 3), based Jerome Workman Jr. serves on the Editorial Advisory Board upon the work of Anscombe (7), the currently recommended of Spectroscopy and is director of research, technology, and applica- method for assessing linearity is faulty because it cannot dis- tions development for the Molecular Spectroscopy & Microanalysis tinguish linear from non-linear data, nor can it distinguish division of Thermo Electron Corp. He can be reached by e-mail at: between non-linearity and other types of defects in the data. [email protected]. Howard Mark serves on the But an extension of that method can. Editorial Advisory Board of Spectroscopy Expanding a Definition and runs a consulting In our recent column we proposed a definition of linearity service, Mark (2). We defined linearity as “The property of data comparing Electronics (Suffern, test results to actual concentrations, such that a straight line NY). He can be provides as good a fit (using the least-squares criterion) as any reached via e-mail at: other mathematical function.”This almost seems to be the [email protected]. same as the FDA/ICH approach, which we have just discred- ited. But there is a difference. The difference is the question of 26 Spectroscopy 20(9) September 2005 www.spectroscopyonline.com Chemometrics in Spectroscopy Table I. The results of applying the new method of detecting non-linearity to Anscombe’s data sets (linear and non-linear). Parameter Coefficient when using t-value when using Coefficient using t-value using only linear term only linear term square term square term Results for non-linear data Constant 3.000 4.268 Linear term 0.500 4.24 0.5000 3135.5 Square term -- -- -0.1267 -2219.2 SEE 1.237 0.0017 R 0.816 1.0 Results for normal data Constant 3.000 3.316 Linear term 0.500 4.24 0.500 4.1 Square term -- -- -0.0316 -0.729 SEE 1.237 1.27 R 0.816 0.8291 fitting other possible functions to the do it. Many texts exist dealing with this highest power to which the variable is data; the FDA/ICH guidelines only subject, but we will follow the presenta- raised in that polynomial). If we have specify trying to fit a straight line to the tion of Arden (5). Arden points out and chosen the wrong function, then there data. This also is more in line with our discusses in detail many applications of might be some error in the estimate of own proposed definition of linearity. numerical analysis: fitting data, deter- data between the known data points, We can try to fit functions other than a mining derivatives and integrals, inter- but at the data points the error must be straight line to the data, and if we can- polation (and extrapolation), solving zero. A good deal of mathematical not obtain an improved fit, we can con- systems of equations, and solving dif- analysis goes into estimating the error clude that the data is linear. ferential equations. These methods are that can occur between the data points. But it also is possible to fit other func- all based on using a Taylor series to tions to a set of data, using least-squared form an approximation to a function Approximation mathematics. In fact, this is what the describing a set of data. The nature of The concepts of interest to us are con- Savitzky–Golay method does. The the data, and the nature of the approxi- tained in Arden’s book in a chapter ti- Savitzky–Golay algorithm, however, does mation considered differs from what we tled “Approximation.”This chapter takes a whole bunch of things, and lumps all are used to thinking about, however. a slightly different tack than the rest of those things together in a single set of The data is assumed to be univariate the discussion, but one that goes exactly convolution coefficients: it includes (which is why this is of interest to us in the direction that we want to go. In smoothing, differentiation, curve-fitting here) and to follow the form of some this chapter, the scenario described of polynomials of various degrees, least- mathematical function, although we above is changed very slightly. There is squares calculations, does not include might not know what the function is. still the assumption that there is a single interpolation (although it could), and All the applications mentioned there- (univariate) mathematical system (cor- finally combines all those operations fore are based upon the concept that responding to “analyte concentration” into a single set of numbers that you can since a function exists, our task is to and “test reading”), and that there is a multiply your measured data by to get estimate the nature of that function, functional relationship between the two the desired final answer directly. using a Taylor series, and then evaluate variables of interest, although again, the For our purposes, though, we don’t the parameters of the function by nature of the relationship might be un- want to lump all those operations imposing the condition that our known. The difference, however, is the together. Rather, we want to separate approximating function must pass recognition that data might have error, them and retain only those operations through all the data points available, and therefore we no longer impose the that are useful for our own purposes. because those data points all are condition that the function we arrive at For starters, we discard the smoothing, described exactly by that function. must pass through every data point. We derivatives, and performing a successive Using a Taylor series implies that the replace that criterion with a different (running) fit over different portions of approximating function that we wind criterion — the criterion we use is one the data set, and keep only the curve- up with will be a polynomial, and per- that will allow us to say that the func- fitting. Texts dealing with numerical haps one of very high degree (the tion we use to describe the data “fol- analysis tell us what to do and how to “degree” of a polynomial being the lows” the data in some sense. While 28 Spectroscopy 20(9) September 2005 www.spectroscopyonline.com Chemometrics in Spectroscopy other criteria can be used, a common criterion used for this ai and setting each of those derivatives equal to zero. Note that purpose is the “least squares” principle: to find parameters for because there are n + 1 different ai we wind up with n + 1 any given function that minimize the sum of the squares of equations, although we only show the first three of the set: the differences between the data and a corresponding point of the function.
Details
-
File Typepdf
-
Upload Time-
-
Content LanguagesEnglish
-
Upload UserAnonymous/Not logged-in
-
File Pages7 Page
-
File Size-