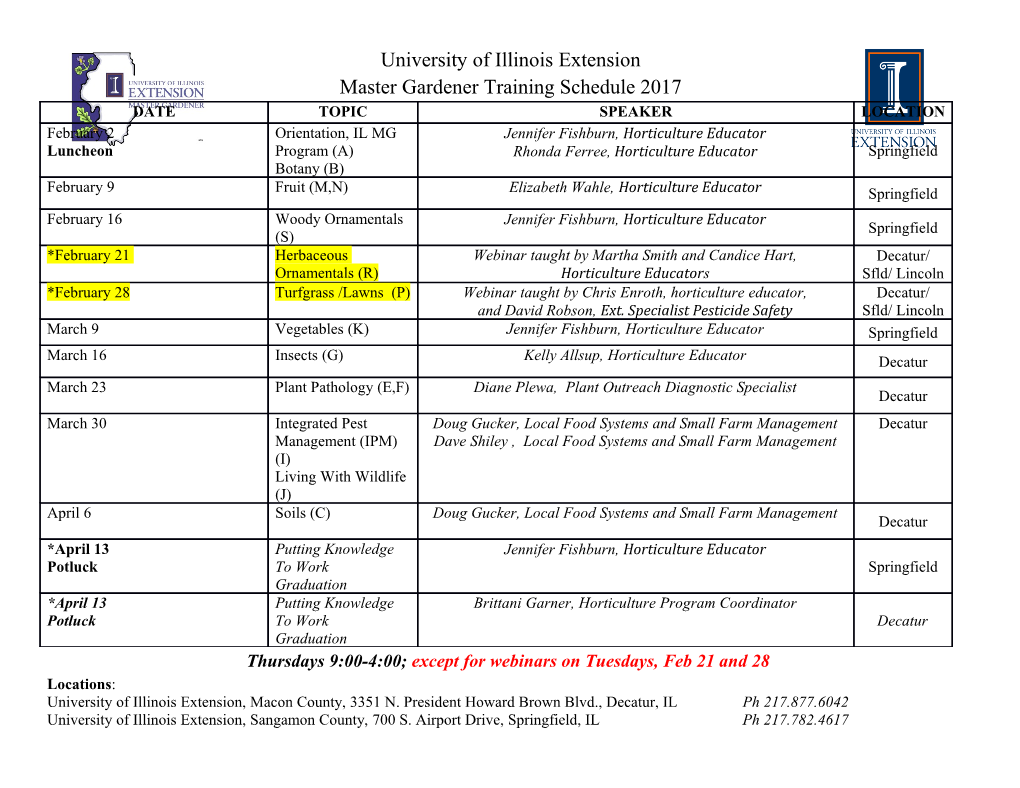
Universita` degli Studi di Pisa Dipartimento di Informatica Dottorato di Ricerca in Informatica Settore Scientifico Disciplinare: INF/01 Ph.D. Thesis Social Network Dynamics Giulio Rossetti Supervisor Supervisor Dino Pedreschi Fosca Giannotti University of Pisa ISTI-CNR May 5, 2015 Abstract This thesis focuses on the analysis of structural and topological network problems. In particular, in this work the privileged subjects of investigation will be both static and dynamic social networks. Nowadays, the constantly growing availability of Big Data describing human behaviors (i.e., the ones provided by online social networks, telco companies, insurances, airline companies. ) of- fers the chance to evaluate and validate, on large scale realities, the performances of algorithmic approaches and the soundness of sociological theories. In this scenario, exploiting data-driven methodologies enables for a more careful modeling and thorough understanding of observed phe- nomena. In the last decade, graph theory has lived a second youth: the scientific community has extensively adopted, and sharpened, its tools to shape the so called Network Science. Within this highly active field of research, it is recently emerged the need to extend classic network analytical methodologies in order to cope with a very important, previously underestimated, semantic infor- mation: time. Such awareness has been the linchpin for recent works that have started to redefine form scratch well known network problems in order to better understand the evolving nature of human interactions. Indeed, social networks are highly dynamic realities: nodes and edges appear and disappear as time goes by describing the natural lives of social ties: for this reason. it is mandatory to assess the impact that time-aware approaches have on the solution of network prob- lems. Moving from the analysis of the strength of social ties, passing through node ranking and link prediction till reaching community discovery, this thesis aims to discuss data-driven methodologies specifically tailored to approach social network issues in semantic enriched scenarios. To this end, both static and dynamic analytical processes will be introduced and tested on real world data. 4 5 The only reason for time is so that everything doesn't happen at once. | Albert Einstein 6 Contents 1 Introduction 15 I Setting the Stage 21 2 Network Analysis 23 2.1 The Graph Representation . 24 2.2 Network Properties . 26 2.3 Network Models . 29 2.3.1 Random Graphs . 29 2.3.2 Small World . 29 2.3.3 Scale Free . 31 2.3.4 Forest Fire . 31 3 Complex Networks and Time 33 3.1 Local Structures . 36 3.2 Topologies . 40 3.3 Diffusion of Information . 42 4 Related Works 43 4.1 Local Structures: Individual Entities analysis . 44 4.1.1 Tie Strength . 44 4.1.2 Link Prediction . 44 4.1.3 Link-Based Object Ranking . 46 4.1.4 Multiplex Networks . 46 4.2 Topologies: Collective analysis . 48 4.2.1 Community Discovery . 48 4.2.2 Network Quantification . 50 4.2.3 Social Engagement . 51 4.3 Diffusion of Information . 52 4.4 Temporal Networks . 53 5 Social Network Data 55 5.1 Social Network Analysis . 56 5.1.1 Social Networks . 57 5.1.2 Collaboration Networks . 57 5.2 Static vs. Dynamic Social Networks . 58 II Frozen in Time: Portrait of a Social Network 59 6 Understanding Local Structures 61 6.1 Multidimensional Networks . 62 8 CHAPTER 0. CONTENTS 6.1.1 Multidimensional network measures . 62 6.2 Ties Strength . 66 6.2.1 Multidimensional Formulation . 66 6.3 Link-Based Object Ranking . 71 6.3.1 Network-Based Human Resources . 72 6.3.2 The Ubik Algorithm . 74 7 Understanding Topologies 83 7.1 The Modular Organization of a Network . 84 7.1.1 The Demon Algorithm . 86 7.1.2 The Overlap in Social Networks . 99 7.2 Homophily and Quantification . 104 7.2.1 Community Discovery for Quantification . 106 7.2.2 Ego-networks for Quantification . 107 7.3 Social Engagement: Skype . 114 7.3.1 Understanding Group Engagement . 115 III Social Dynamics: Networks through Time 127 8 Modeling Individual Dynamics 129 8.1 Unsupervised Link Prediction . 130 8.1.1 Multidimensional Link Prediction . 130 8.2 Superivsed Interaction Prediction . 140 8.2.1 Time-Aware Interaction Prediction . 141 9 Modeling Collective Dynamics 155 9.1 Evolutionary Community Discovery . 156 9.1.1 The Tiles algorithm . 158 10 Information Diffusion 171 10.1 Social Prominence . 172 10.1.1 Leader Characterization . 172 10.1.2 Local Diffusion Measures . 175 11 Conclusion 185 Bibliography 187 Index 199 List of Figures 1.1 From static to dynamic: Individual and Collective analysis . 17 2.1 Graph Topologies . 24 2.2 Graph Properties: Toy example . 26 2.3 Small World connectivity . 30 2.4 Scale Free network growth . 31 3.1 Dynamic network problems and their classification . 34 3.2 Graphical representation of tie strength in a social context. 36 3.3 Link Prediction: an example. 37 3.4 Multidimensional Network: An example . 38 5.1 From Static to Dynamic: Network representations . 58 6.1 Multidimensional Measures: Toy example . 62 6.2 Ties Strength: Local and global visualization . 67 6.3 Ties Strength: 4-dimensional network details . 68 6.4 Ties Strength: Network resilience . 69 6.5 Ties Strength: Measure correlations . 69 6.6 Ubik: Toy example . 73 6.7 Ubik: Running times . 76 6.8 Ubik: q-q plots against degree centrality ranking . 77 7.1 Demon: Intuition example . 84 7.2 Demon: Label Propagation example . 88 7.3 Demon: F-measure in benchmark networks . 93 7.4 Demon: impact . 96 7.5 Demon: Distribution of community sizes . 96 7.6 Demon: Amazon example . 97 7.7 Demon: Overlap in multidimensional network . 100 7.8 Demon: Multidimensional overlap in Facebook . 101 7.9 Quantification: Quantification vs Classification . 105 7.10 Quantification: Community-based approach . 106 7.11 Quantification: 1-hop and 2-hop ego-networks . 107 7.12 Quantification: Ego-network based approach . 108 7.13 Quantification: Label frequencies . 109 7.14 Quantification: Label ferquency distribution . 110 7.15 Group Engagement: From local to global . 115 7.16 Group Engagement: Approaches characterization . 116 7.17 Distribution of community size for HDemon, Louvain, Ego-Networks and BFS. 119 7.18 Group Engagements: SGD weigths in balanced scenario . 121 7.19 Group Engagements: AUC study in balanced scenario . 122 7.20 Group Engagements: Lift charts unbalanced scenario . 122 10 CHAPTER 0. LIST OF FIGURES 7.21 Group Engagements: SGD weights in unbalanced scenario . 124 7.22 Group Engagements: AUC study in unbalanced scenario . 124 7.23 Group Engagements: Pearson Correlation . 125 8.1 Link Prediction: Toy example . 132 8.2 Link Prediction: ROC curves for Neighbors models . 136 8.3 Link Prediction: ROC curves for NeighborsXOR models . 137 8.4 Link Prediction: Running times . 138 8.5 Supervised Link Prediction: Moving Average AUC . 147 8.6 Supervised Link Prediction: ROC curves balanced scenario . 148 8.7 Supervised Link Prediction: Feature relevance . 149 8.8 Supervised Link Prediction: Squared errors per feature . 152 8.9.
Details
-
File Typepdf
-
Upload Time-
-
Content LanguagesEnglish
-
Upload UserAnonymous/Not logged-in
-
File Pages199 Page
-
File Size-