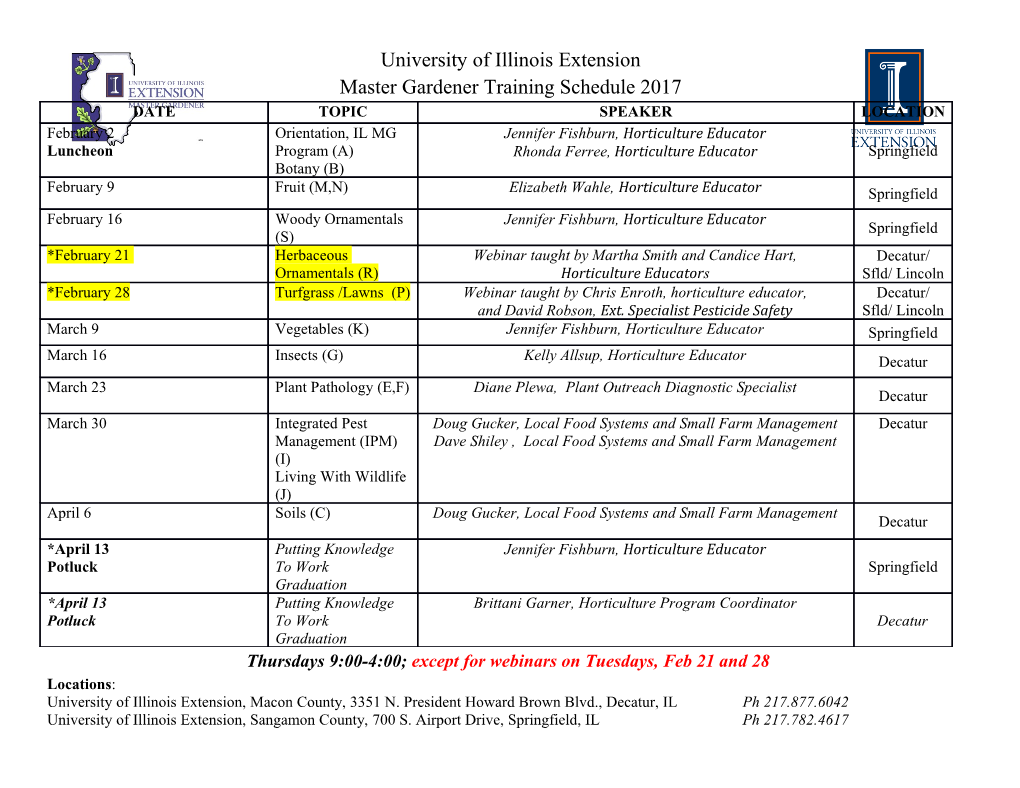
ARTICLE IN PRESS Atmospheric Environment 41 (2007) 3082–3094 www.elsevier.com/locate/atmosenv Ensemble-based data assimilation and targeted observation of a chemical tracer in a sea breeze model Amy L. Stuarta,Ã, Altug Aksoyb, Fuqing Zhangc, John W. Nielsen-Gammonc aDepartments of Environmental and Occupational Health and Civil and Environmental Engineering, University of South Florida, 13201 Bruce B. Downs Boulevard, MDC-56, Tampa, FL 33612-3805, USA bNational Center for Atmospheric Research, Boulder, CO, USA cDepartment of Atmospheric Sciences, Texas A&M University, College Station, TX, USA Received 5 June 2006; received in revised form 25 September 2006; accepted 28 November 2006 Abstract We study the use of ensemble-based Kalman filtering of chemical observations for constraining forecast uncertainties and for selecting targeted observations. Using a coupled model of two-dimensional sea breeze dynamics and chemical tracer transport, we perform three numerical experiments. First, we investigate the chemical tracer forecast uncertainties associated with meteorological initial condition and forcing error. We find that the ensemble variance and error builds during the transition between land and sea breeze phases of the circulation. Second, we investigate the effects on the forecast variance and error of assimilating tracer concentration observations extracted from a truth simulation for a network of surface locations. We find that assimilation reduces the variance and error in both the observed variable (chemical tracer concentrations) and unobserved meteorological variables (vorticity and buoyancy). Finally, we investigate the potential value to the forecast of targeted observations. We calculate an observation impact factor that maximizes the total decrease in model uncertainty summed over all state variables. We find that locations of optimal targeted observations remain similar before and after assimilation of regular network observations. r 2006 Elsevier Ltd. All rights reserved. Keywords: Air quality modeling; Data assimilation; Ensemble modeling; Adaptive observations 1. Introduction nificant uncertainties (Seigneur, 2005). The com- plexity comes in large part from three areas. First, Significant strides in understanding and mitigat- air pollution involves numerous nonlinear physical ing air pollution have been achieved over the past and chemical processes, many of which are not well several decades (Seinfeld, 2004). Despite this pro- understood. Second, the characteristic spatial and gress, modeling and prediction of air pollution and time scales of these processes span many orders of its effects remains difficult and is prone to sig- magnitude. Due to poor understanding of some processes and the computational cost of resolving all processes at the appropriate scale, many ÃCorresponding author. Tel.: +1 813 974 6632; fax: +1 813 974 4986. processes are parameterized in models. These para- E-mail address: [email protected] (A.L. Stuart). meterizations are, by nature, only approximations, 1352-2310/$ - see front matter r 2006 Elsevier Ltd. All rights reserved. doi:10.1016/j.atmosenv.2006.11.046 ARTICLE IN PRESS A.L. Stuart et al. / Atmospheric Environment 41 (2007) 3082–3094 3083 and hence have errors and uncertainties associated short-term monitoring, for specific management and with them. Finally, uncertainties in the initial and research purposes. Recently, interest has escalated boundary conditions lead to uncertain predictions in observation targeting for response to air pollu- of air quality. The chaotic nature of the meteor- tion hazards that result from emergency releases. ological system governing underlying atmospheric In the weather forecasting field, there has been transport (Lorenz, 1963) can often cause even small significant research on adaptive location of targeted uncertainties to grow substantially in time. observations using techniques originating with data Due to the uncertain nature of the prediction of assimilation (Emanuel et al., 1995; Emanuel and air pollution hazards (whether due to routine Langland, 1998; Lorenz and Emanuel, 1998; Berli- emissions or emergency releases), simulation tech- ner et al., 1999) that could be applied to air quality niques combining deterministic modeling with network design. statistical methods (i.e. Monte Carlo methods) have Although data assimilation is used operationally sometimes been used to determine air pollution for meteorological modeling and prediction, its use hazard probabilities and to explore the sensitivity of for air quality modeling is less developed. None- predictions to underlying input and model uncer- theless, it has been found to be useful as an inverse tainties (e.g. Boybeyi et al., 1995; Stuart et al., 1996; modeling technique for diagnosing pollutant emis- Bergin et al., 1999; Dabberdt and Miller, 2000; Sax sion source locations and strengths (i.e. parameter and Isakov, 2003; Zhang et al., 2006). One such estimation) (Chang et al., 1997; Elbern et al., 2000; statistical technique that has significant promise Mendoza-Dominguez and Russell, 2001) and for for improving air quality modeling and prediction identifying locations (in time and space) for field is four-dimensional data assimilation (FDDA). observation networks and adaptive observations FDDA combines observational data, knowledge (Daescu and Carmichael, 2003). Much of this of the physical and chemical system behavior (as previous work has focused on variational data represented in predictive models), and information assimilation techniques (three-dimensional and on the uncertainty in both the observed data and the four-dimensional variational assimilation, 3DVAR model representation over space and time (Kalnay, and 4DVAR). 2003). By integrating this information, data assim- Ensemble-based Kalman filtering is an alternative ilation can provide a more accurate description of data assimilation approach that is undergoing the system state (i.e., multidimensional information significant investigation for many environmental on wind speed, wind direction, temperature, and modeling applications (Evensen, 2003). Advantages chemical concentration) and its expected evolution include lower computational costs than extended in time. Data assimilation can also be used to Kalman filtering and explicit calculation of the constrain errors associated with the uncertain model nonlinear evolution of background covariances parameterizations and to determine parameter through the ensemble forecast. Explicit calculation values, through on-line optimization of the para- of covariance eliminates the assumption of statio- meters used (‘‘parameter estimation’’) (e.g., Navon, narity of covariances used in 3DVAR, and alleviates 1998). the need for the development of tangent linear and In addition to improving model predictions, data adjoint models of the dynamics used in extended assimilation research has led to the development of Kalman filtering and 4DVAR (Tippett et al., 2003; tools such as adjoint models for the selection and Kalnay, 2003). Heemink and collaborators have design of observational networks and targeted investigated ensemble-based Kalman filtering and observations, as it provides a mechanism for on- other Kalman filtering techniques for prediction of line optimization of this selection (Morss et al., ozone concentrations over Europe with two- and 2001). Existing long-term air quality observational three-dimensional chemical transport models that networks are generally fixed in space and have very are decoupled (calculated off-line) from the under- sparse spatial and temporal resolution. The sparse lying meteorological dynamics simulation (Hanea resolution is due to the number of variables that et al., 2004; Heemink and Segers, 2002). Their work need to be observed (several meteorological vari- indicates improvement in model predictions with ables and up to hundreds of toxic chemical filtering, and demonstrates the feasibility of Kalman pollutants) and large equipment and operational filtering techniques for modeling air quality. expenses. In addition to long-term fixed locations, Here, we study the utility of ensemble-based targeted observational locations are often used for Kalman filtering in the context of a two-dimensional ARTICLE IN PRESS 3084 A.L. Stuart et al. / Atmospheric Environment 41 (2007) 3082–3094 sea breeze model in which chemical tracer transport The model spatial domain represents the cross- is directly coupled to the underlying nonlinear shore horizontal distance and the vertical above- meteorological dynamics. The sea breeze circulation ground altitude. The coast is located at horizontal is an important weather pattern affecting coastal domain center. The force that drives the sea breeze areas. Since many cities are located near coasts, these circulation is modeled as an explicit volume buoy- circulations have important impacts on the forma- ancy source that represents the differential heating tion and transport of urban air pollution. In the over land and sea. The source function varies United States, two of the most polluted cities, horizontally as an arc tangent with the inflection Houston and Los Angeles, are located on coastlines point at the coast-line, decays exponentially with for which sea breeze circulations affect air quality. vertical distance from the ground, and varies For example, observational and modeling studies sinusoidally in time but with added stochastic noise. (Banta et al., 2005; Bao et al., 2005; Zhang et al., Free slip and thermal insulation are assumed at 2006) have
Details
-
File Typepdf
-
Upload Time-
-
Content LanguagesEnglish
-
Upload UserAnonymous/Not logged-in
-
File Pages13 Page
-
File Size-