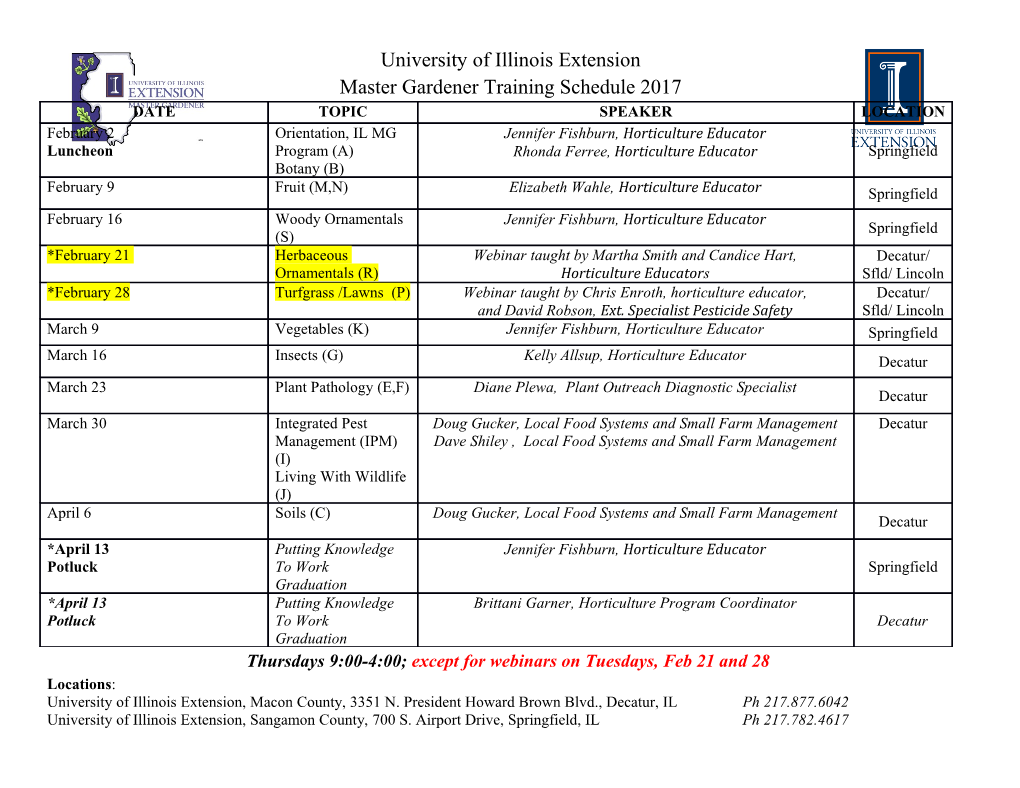
Cesare Palestini Engine crankshaft torsional vibration analysis for anomalies detection Thesis submitted for examination for the degree of Master of Science in Technology. Espoo 31.5.2018 Supervisor: Professor Petri Kuosmanen Advisors: Dr Irene Gallici and Dr Juho Könnö Copyright ⃝c 2018 Cesare Palestini Aalto University, P.O. BOX 11000, 00076 AALTO www.aalto.fi Abstract of the master’s thesis Author Cesare Palestini Title Engine crankshaft torsional vibration analysis for anomalies detection Degree programme Mechanical Engineering Major Master’s Programme in Mechanical Engineering Code of major ENG25 Supervisor Professor Petri Kuosmanen Advisors Dr Irene Gallici, Dr Juho Könnö Date 31.5.2018 Number of pages 61+4 Language English Abstract Reliability is a key factor in medium speed engines. Since they are employed in power plants and vessels, there is the constant need to increase the accuracy of their anomaly detection loops. Here I investigated a novel idea aimed at decreasing the number of sensors deployed. The goal was to understand whether using only one speed sensor, located at the flywheel, is enough to detect and possibly classify different abnormal operating conditions. A torsional vibration model of a V20 spark-ignited gas engine was developed and validated with field data. The model was then used to simulate normal, heavy knock, misfire and overpressure conditions. Subsequently, the speed signals ofthe crankshaft, measured at the flywheel, were analysed in the time- and frequency- domains, in order to identify possible patterns that could be linked to the four scenarios. The spectra of the signals were then processed with two machine-learning approaches: pattern recognition and a neural network. Both approaches showed accuracies up to 97% in the classification of the scenarios. Amongst the pattern recognition algorithms, the Ensemble with Subspace kNN proved to be the most accurate one, with a remarkably low rate of false negatives (<1%). The neural network had a slightly lower accuracy in the binary identification between normal and abnormal situations, but it performed better in classifying the different anomalies. The results are promising. They indicate that, withsome further improvement, the method tested and developed in this thesis could be a reliable alternative to the safety systems currently in use in engines. Keywords torsional vibration, speed measurement, multi-body dynamics, anomalies detection, machine learning, pattern recognition, artificial neural network, data analytics, heavy knock, overpressure, misfire Acknowledgements This master’s thesis is part of a research project funded by Wärtsilä Finland Oy and it aims to investigate new horizons in the field of reliability engineering. It was a fantastic opportunity for me to discover a fascinating world populated by huge engines, learn new things in the field of the systems simulation and the data analysis, and to grow professionally and as a person. I want to thank my supervisor Professor Petri Kuosmanen and my advisors Irene Gallici and Juho Könnö for the guidance received. I also thank the owner and the manager of the research project, Andreas Hjort and Fabio Mauceri, for their support. In the time spent working on my thesis I had the possibility to meet extremely skilled and interesting colleagues that shared their knowledge with me. Among them, I want to cite Harry Särs for the insights on engines technology and the logistics assistance, Jan Kaas and Markus Björkvall for their help with the system simulation, Andrea Greco for his coaching on the data analysis. Last but not least, Jari Hyvönen for sharing his expertise. Without their precious hints this work would not have been possible. Finally, I thank also Håkan Nynäs for the pleasant moments spent together with me and Harry talking about technology and funny stories of the past times. I also want to take this opportunity to thank all the people that in these years in Finland supported me in my master studies: my family and my life partner Maria, the old and the new friends, the Wasa fellas. Otaniemi, 31.5.2018 Cesare Palestini iv CONTENTS Contents Abstract iii Acknowledgements iv Contentsv Symbols and abbreviations vii 1 Introduction1 1.1 Background................................1 1.2 Research problem.............................1 1.3 Scope...................................1 1.4 Aim of the work..............................2 1.5 Methods..................................2 2 Theory4 2.1 Engine knock...............................4 2.2 Cylinder overpressure...........................7 2.3 Engine misfire...............................8 2.4 Anomaly detection algorithms......................9 2.4.1 Pattern recognition algorithms.................. 10 2.4.2 Artificial neural network..................... 10 3 Methods 13 3.1 Torsional vibration model........................ 13 3.1.1 Engine............................... 13 3.1.2 Coupling.............................. 20 3.1.3 Generator............................. 21 3.1.4 Assumptions............................ 22 3.2 Model Validation............................. 22 3.2.1 Flywheel speed analysis..................... 23 3.2.2 Expected behavior analysis in presence of an anomaly..... 25 3.3 Sensitivity of the model......................... 26 3.4 Simulations................................ 26 3.5 Testing method.............................. 29 3.5.1 Pattern recognition algorithms.................. 29 3.5.2 Neural network.......................... 30 3.6 Limitations................................ 30 3.6.1 Simulation model......................... 30 3.6.2 Real engine............................ 32 3.6.3 Algorithms............................ 33 3.7 Accuracy and possible sources of error................. 34 3.7.1 Simulation model......................... 34 3.7.2 Data processing.......................... 35 v CONTENTS 3.7.3 Algorithms............................ 35 4 Results 36 4.1 Post-processed simulation results.................... 36 4.2 Pattern recognition algorithms...................... 40 4.3 Artificial neural network......................... 44 5 Discussion 47 6 Conclusions 51 References 53 Appendix 55 vi CONTENTS Symbols and abbreviations Symbols zi incremental stage variable zˆi approximation of the incremental stage variable εi truncation error h step size y vector containing unknown variables f frequency Operators ˙ derivative with respect to time Abbreviations LNG liquefied natural gas IEC International Electrotechnical Commission SIL safety integrity level MTBF mean time between failures SI spark-ignition SG spark-ignited gas BMEP brake mean effective pressure KI knock intensity GUI graphical user interface FEM finite element method CAD computer aided design CA crankshaft angle LPF low pass filter FFT fast Fourier transform ML machine learning PRA pattern recognition algorithm ANN artificial neural network TDC top dead center kNN k nearest neighbors SVM support vector machines ROC receiver operating characteristic FPR false positive rate TPR true positive rate vii 1 Introduction 1.1 Background Large combustion engines are the main source of energy in ships and certain types of power plants. Wärtsilä is a leading company in the development and production of these engines, which can use different fuels, from heavy fuel to LNG. It operates globally and offers tailored solutions for both marine and energy applications. For energy production, the standard set-up usually consists in an engine connected to a generator by means of a flexible coupling, and the reliability of these systems is one of the key concerns that must be addressed. 1.2 Research problem When operating large engines, it is imperative to minimize the risk of failure, since it can lead to additional costs due to repair, and cause temporary unwanted shut-downs and potentially harmful situations. Many customers require the manufacturers of the engines to equip their installations with safety systems that satisfy specific safety standards. In this case study, the standard involved is defined by the International Electrotechnical Commission as IEC 61508 and concerns the functional safety of electrical/electronic/programmable electronic safety-related systems. This standard defines four levels of risk reduction called Safety Integrity Level (SIL). The levels rank the different control systems, according to criteria of failure rate, redundancy, likelihood of failure, differentiation and criticality of the potentially harmful situation in case of failure. They range from 1 (the lowest) to 4 (the highest). 1.3 Scope There are several events that can be harmful for an engine, and they should be prevented or at least detected as soon as possible, to avoid damages to the engine or explosions. For spark ignition engines, one of these events is engine knock. Engine knock is defined by Wang, Liu and Reitz as “the name given to the noise associated with the auto-ignition of a portion of the fuel-air mixture ahead of the propagating flame front” in the combustion chamber (Wang, Liu, and D. Reitz 2017). It can damage the piston rings, cause seizure, piston and cylinder head overheating and, in the most extreme cases, lead to an explosion and to non-repairable damages. To detect the knock, the most common method consists in using one or several piezoelectric sensors called “knock sensors”, located on the cylinder heads or on the engine block. These sensors are effective but their failure rate is too high to comply with thestrict requirements of the SIL-2 standard. Another potentially harmful
Details
-
File Typepdf
-
Upload Time-
-
Content LanguagesEnglish
-
Upload UserAnonymous/Not logged-in
-
File Pages65 Page
-
File Size-