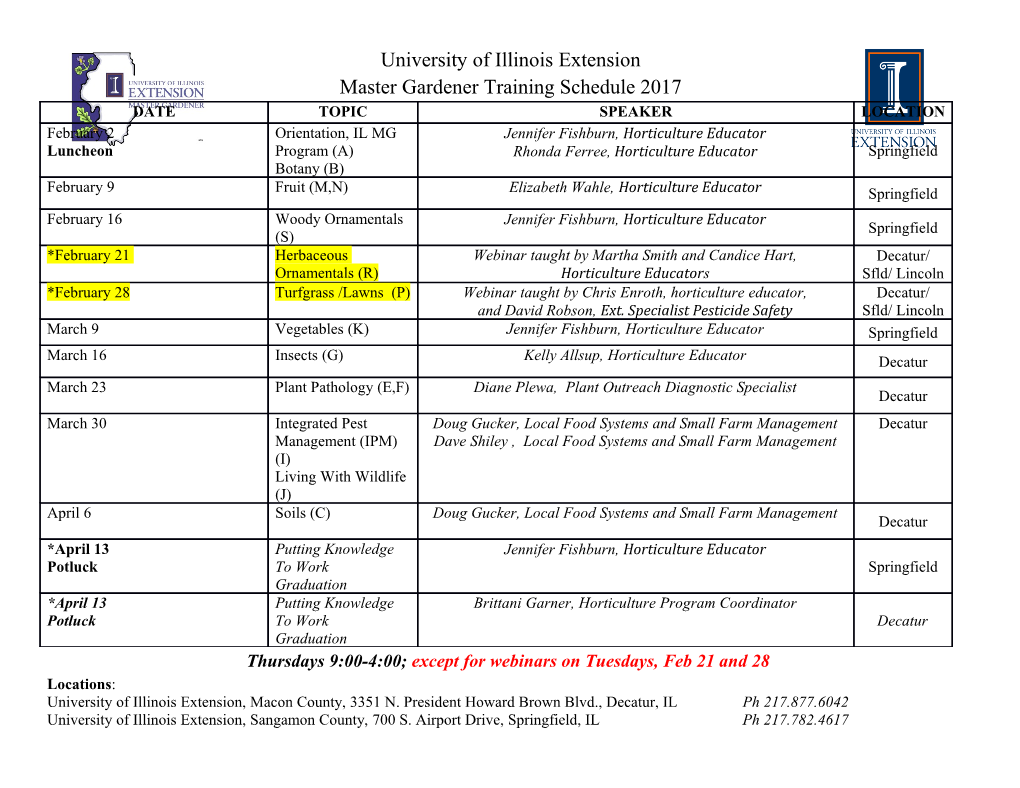
PHYSICAL REVIEW E 81, 031105 ͑2010͒ 1Õf noise from nonlinear stochastic differential equations J. Ruseckas* and B. Kaulakys Institute of Theoretical Physics and Astronomy, Vilnius University, A. Goštauto 12, LT-01108 Vilnius, Lithuania ͑Received 20 October 2009; published 8 March 2010͒ We consider a class of nonlinear stochastic differential equations, giving the power-law behavior of the power spectral density in any desirably wide range of frequency. Such equations were obtained starting from the point process models of 1/ f noise. In this article the power-law behavior of spectrum is derived directly from the stochastic differential equations, without using the point process models. The analysis reveals that the power spectrum may be represented as a sum of the Lorentzian spectra. Such a derivation provides additional justification of equations, expands the class of equations generating 1/ f noise, and provides further insights into the origin of 1/ f noise. DOI: 10.1103/PhysRevE.81.031105 PACS number͑s͒: 05.40.Ϫa, 72.70.ϩm, 89.75.Da I. INTRODUCTION signals with 1/ f noise were obtained in Refs. ͓29,30͔͑see ͓ ͔͒ Power-law distributions of spectra of signals, including also recent papers 5,31 , starting from the point process / ͓ ͔ 1/ f noise ͑also known as 1/ f fluctuations, flicker noise, and model of 1 f noise 27,32–39 . pink noise͒, as well as scaling behavior in general, are ubiq- The purpose of this article is to derive the behavior of the uitous in physics and in many other fields, including natural power spectral density directly from the SDE, without using phenomena, human activities, traffics in computer networks, the point process model. Such a derivation offers additional and financial markets. This subject has been a hot research justification of the proposed SDE and provides further in- / topic for many decades ͑see, e.g., a bibliographic list of pa- sights into the origin of 1 f noise. pers by Li ͓1͔, and a short review in Scholarpedia ͓2͔͒. II. PROPOSED STOCHASTIC DIFFERENTIAL Despite the numerous models and theories proposed since EQUATIONS its discovery more than 80 years ago ͓3,4͔, the intrinsic ori- gin of 1/ f noise still remains an open question. There is no Starting from the point process model, proposed and ana- conventional picture of the phenomenon and the mechanism lyzed in Refs. ͓27,32–38͔, the nonlinear stochastic differen- leading to 1/ f fluctuations are not often clear. Most of the tial equations are derived ͓5,29,30͔. The general expression models and theories have restricted validity because of the for the SDE is assumptions specific to the problem under consideration. A / short categorization of the theories and models of 1 f noise dx = 2ͩ − ͪx2−1dt + xdW. ͑1͒ is presented in the introduction of the paper ͓5͔. 2 Until recently, probably the most general and common / Here, x is the signal, is the exponent of the multiplicative models, theories and explanations of 1 f noise have been based on some formal mathematical description such as frac- noise, defines the behavior of stationary probability distri- tional Brownian motion, the half-integral of the white noise, bution, and W is a standard Wiener process. SDE ͑1͒ has the simplest form of the multiplicative noise or some algorithms for generation of signals with scaled properties ͓6–14͔ and the popular modeling of 1/ f noise as term, x dW. Multiplicative equations with the drift coeffi- the superposition of independent elementary processes with cient proportional to the Stratonovich drift correction for the Lorentzian spectra and a proper distribution of relaxation transformation from the Stratonovich to the Itô stochastic / ͓ ͔ equation ͓40͔ generate signals with the power-law distribu- times, e.g., a 1 relax distribution 15–21 . The weakness of ͓ ͔ ͑ ͒ the latter approach is that the simulation of 1/ f noise with tions 5 . Equation 1 is of such type and has probability ͑ ͒ϳ − the desirable slope  requires finding the special distribu- distribution of the power-law form P x x . Because of the divergence of the power-law distribution and the requirement tions of parameters of the system under consideration; at ͑ ͒ least a wide range of relaxation time constants should be of the stationarity of the process, the SDE 1 should be assumed in order to allow correlation with experiments analyzed together with the appropriate restrictions of the dif- ͓22–28͔. fusion in some finite interval. For simplicity, in this article, Nonlinear stochastic differential equation with linear we will adopt reflective boundary conditions at x=xmin and noise and nonlinear drift, was considered in Ref. ͓9͔.Itwas x=xmax. However, other forms of restrictions are possible. For example, exponential restriction of the diffusion can be found that if the damping is decreasing with increase in the ͑ ͒ absolute value of the stochastic variable, then the solution of obtained by introducing additional terms in Eq. 1 , such a nonlinear stochastic differential equation ͑SDE͒ has m m m xmin m x long correlation time. Recently nonlinear SDEs generating dx = 2ͩ − + ͩ ͪ − ͩ ͪ ͪx2 −1dt + x dW. 2 2 x 2 xmax ͑2͒ *[email protected] Here, m is some parameter. 1539-3755/2010/81͑3͒/031105͑7͒ 031105-1 ©2010 The American Physical Society J. RUSECKAS AND B. KAULAKYS PHYSICAL REVIEW E 81, 031105 ͑2010͒ ͑ ͒ ץ Equation 1 with the reflective boundary condition at xmin 1 S͑x,t͒ = ͩ − ͪx2−1P − x2P. ͑10͒ ץ and xmax can be rewritten in a form that does not contain 2 2 x parameters and xmin. Introducing the scaled stochastic vari- → / →2 2−2 able x x xmin and scaled time t xmin t one transforms At the reflective boundaries xmin=1 and xmax= the probabil- Eq. ͑1͒ to ity current S͑x,t͒ should vanish, and, therefore, the boundary conditions for Eq. ͑8͒ are 2−1 dx = ͩ − ͪx dt + x dW. ͑3͒ ͑ ͒ ͑ ͒ ͑ ͒ 2 S 1,t =0, S ,t =0. 11 The scaled Eq. ͑3͒ has a boundary at x=1 and at A. Eigenfunction expansion xmax = . ͑4͒ ͑ ͒ x We solve Eq. 8 using the method of eigenfunctions. An min ansatz of the form Further, we will consider Eq. ͑3͒ only. In order to obtain 1/ f ͑ ͒ ͑ ͒ −t ͑ ͒ noise we require that the region of diffusion of the stochastic P x,t = P x e 12 ӷ variable x should be large. Therefore, we assume that 1. leads to the equation 2ץ 1 ץ III. POWER SPECTRAL DENSITY FROM ͩ ͪ 2−1 2 ͑ ͒ ͑ ͒ THE FOKKER-PLANCK EQUATION − − x P + x P =− P x , 13 x2ץ x 2ץ 2 According to Wiener-Khintchine relations, the power ͑ ͒ Ն spectral density is where P x are the eigenfunctions and 0 are the corre- sponding eigenvalues. The eigenfunctions P͑x͒ obey the or- ϱ ϱ thonormality relation ͓42͔ S͑f͒ =2͵ C͑t͒eitdt =4͵ C͑t͒cos͑t͒dt, ͑5͒ −ϱ 0 ͵ ⌽͑x͒ ͑ ͒ ͑ ͒ ␦ ͑ ͒ e P x PЈ x dx = ,Ј, 14 where =2f and C͑t͒ is the autocorrelation function. For 1 the stationary process, the autocorrelation function can be ⌽͑ ͒ ͑ ͒ expressed as an average over realizations of the stochastic where x is the potential, associated with Eq. 8 , process, ⌽͑ ͒ ͑ ͒ ͑ ͒ x =−lnP0 x . 15 ͑ ͒ ͗ ͑ Ј͒ ͑ Ј ͒͘ ͑ ͒ C t = x t x t + t . 6 It should be noted that the restriction of diffusion of the This average can be written as variable x by xmin and xmax ensures that the eigenvalue spec- trum is discrete. Expansion of the transition probability den- ͑ ͒ ͵ ͵ Ј Ј ͑ ͒ ͑ Ј ͉ ͒ ͑ ͒ sity in a series of the eigenfunctions has the form ͓42͔ C t = dx dx xx P0 x Px x ,t x,0 , 7 ⌽͑x͒ −t P ͑xЈ,t͉x,0͒ = ͚ P͑xЈ͒e P͑x͒e . ͑16͒ ͑ ͒ x where P0 x is the steady-state probability distribution func- tion and P ͑xЈ,t͉x,0͒ is the transition probability ͑the condi- x ͑ ͒ ͑ ͒ tional probability that at time t the signal has value xЈ with Substituting Eq. 16 into Eq. 7 we get the autocorrelation the condition that at time t=0 the signal had the value x͒. function The transition probability can be obtained from the solution −t 2 C͑t͒ = ͚ e X. ͑17͒ of the Fokker-Planck equation with the initial condition ͑ ͉ ͒ ␦͑ ͒ Px xЈ,0 x,0 = xЈ−x . Therefore, for the calculation of the power spectral den- Here, sity of the signal x we will use the Fokker-Planck equation instead of stochastic differential Eq. ͑3͒. The Fokker-Planck ͵ ͑ ͒ ͑ ͒ X = xP x dx 18 equation corresponding to the Itô solution of Eq. ͑3͒ is 1 ͓41,42͔ is the first moment of the stochastic variable x evaluated with 2 -the -th eigenfunction P͑x͒. Such an expression for the au ץ 1 ץ ץ P =−ͩ − ͪ x2−1P + x2P. ͑8͒ x2 tocorrelation function has been obtained in Ref. ͓43͔. Usingץ x 2ץ t 2ץ Eqs. ͑5͒ and ͑17͒ we obtain the power spectral density The steady-state solution of Eq. ͑8͒ has the form ͑ ͒ 2 ͑ ͒ −1 − S f =4͚ 2 2 X. 19 x , 1, + 1−1− P ͑x͒ = ͑9͒ 0 Ά 1 · This expression for the power spectral density resembles the −1 x , =1. models of 1/ f noise using the sum of the Lorentzian spectra ln ͓15–18,27,28,44,45͔.
Details
-
File Typepdf
-
Upload Time-
-
Content LanguagesEnglish
-
Upload UserAnonymous/Not logged-in
-
File Pages7 Page
-
File Size-