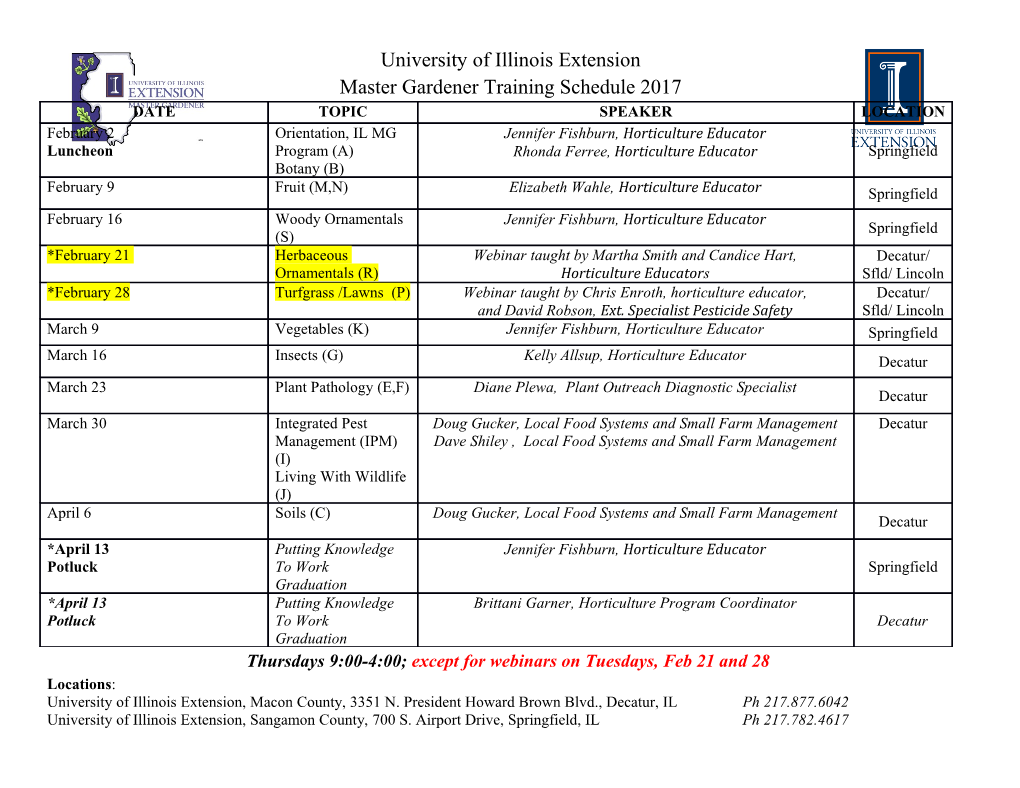
Methods in Statistical Genomics In the Context of Genome-Wide Association Studies Edited by Philip Chester Cooley Methods in Statistical Genomics: In the Context of Genome-Wide Association Studies Edited by Philip Chester Cooley RTI Press ©2016 RTI International. RTI International is a The RTI Press mission is to disseminate registered trademark and a trade name of Research information about RTI research, analytic Triangle Institute. The RTI logo is a registered tools, and technical expertise to a national trademark of Research Triangle Institute. and international audience. RTI Press This work is distributed under the publications are peer-reviewed by at least terms of a Creative Commons two independent substantive experts and Attribution-NonCommercial-NoDerivatives 4.0 one or more Press editors. license (CC BY-NC-ND), a copy of which is available at RTI International is an independent, https://creativecommons.org/licenses/by-nc-nd/4.0 nonprofit research institute dedicated /legalcode. to improving the human condition. We combine scientific rigor and technical Library of Congress Control Number: 2016949391 expertise in social and laboratory sciences, ISBN 978-1-934831-16-8 engineering, and international development (refers to print version) to deliver solutions to the critical needs of RTI Press publication No. BK-0016-1608 clients worldwide. https://doi.org/10.3768/rtipress.2016.bk.0016.1608 www.rti.org/rtipress Cover design: John Theilgard This publication is part of the RTI Press Book series. RTI International 3040 East Cornwallis Road, PO Box 12194 Research Triangle Park, NC 27709-2194, USA [email protected] www.rti.org Contents Chapter 1. Overview of Chapters 1 Philip Chester Cooley Chapter 2. Genome-Wide Association Data: Where Are the Standards? 17 Philip Chester Cooley Chapter 3. Creating the Synthetic Gene Data 31 Philip Chester Cooley Chapter 4. Genetic Inheritance and Genome-Wide Association Statistical Test Performance Using Simulated Data 37 Philip Chester Cooley, Robert F. Clark, Ralph E. Folsom, and Grier Page Chapter 5. The Influence of Errors Inherent in Genome-Wide Association Studies (GWAS) in Relation to Single-Gene Models 49 Philip Chester Cooley, Robert F. Clark, and Grier Page Chapter 6. Conducting Genome-Wide Association Studies (GWAS): Epistasis Scenarios 65 Philip Chester Cooley, Nathan Gaddis, Ralph E. Folsom, and Diane Wagener Chapter 7. Assessing Gene-Environment Interactions in Genome-Wide Association Studies (GWAS): Statistical Approaches 85 Philip Chester Cooley, Robert F. Clark, and Ralph E. Folsom Chapter 8. Polygene Methods in Genome-Wide Association Studies (GWAS) 117 Philip Chester Cooley and Ralph E. Folsom Chapter 9. Conclusions and Recommendations 143 Philip Chester Cooley Acknowledgment 149 Contributors 151 Index 153 CHAPTER 1 Overview of Chapters Philip Chester Cooley Introduction The objective of this book is to describe procedures for analyzing genome-wide association studies (GWAS). Some of the material is unpublished and contains commentary and unpublished research; other material (Chapters 4 through 7) has been published previously. Each previously published chapter investigates a different genomics model, but all focus on identifying the strengths and limitations of various statistical procedures that have been applied to different GWAS scenarios. The distinction between genotype and phenotype was initially presented by the Danish botanist, plant physiologist, and geneticist Wilhelm Johannsen in a book he published in 1905, The Elements of Heredity. He distinguished between the genotype of the organism (it is hereditary) and the ways in which its heredity is demonstrated in phenotypes, or physical characteristics. This distinction was an outgrowth of Johannsen’s experiments concerning heritable variation in plants.1 Today, it is understood that the process leading from genes to proteins that ultimately establish phenotypes is complex. Most proteins are the products of multiple genes. Whether a protein is an enzyme, receptor, or hormone, it functions in a specific environment that includes external factors like temperature, rainfall, the amount of sunlight available, and nutrition, as well as internal factors that can include other hormones, enzymes, and other proteins. Further, biochemical pathways are not always linear; they can have multiple positive and negative feedback loops and may involve multiple steps and the products of hundreds of genes. In summary, the evolutionary forces producing a phenotype may often involve many genes and can be influenced by a variety of specific environmental factors. 2 Chapter 1 The Human Genome Project The Human Genome Project (HGP) was an international scientific project with the goals of determining the sequence of chemical base pairs that make up human DNA and of identifying all of the physical and functional genes of the human genome. The HGP produced the first complete sequences of individual human genomes. As of 2012, thousands of human genomes had been completely sequenced, and many more had been mapped at lower levels of resolution. The resulting data have been used worldwide in biomedical science, anthropology, forensics, and other branches of science. With the mapping of the human genome near completion, researchers expected that subsequent genomic studies would lead to advances in our understanding of human evolution, and advances in many subfields of biology, particularly the diagnosis and treatment of many diseases. To that end, researchers have worked to identify genes that constitute biomarkers using a combination of high-throughput experimental and bioinformatics approaches; nevertheless, the identification of biological functions of the protein and RNA products of DNA has only just begun. Recent results suggest that most of the vast quantities of noncoding DNA within the genome have biochemical activities that include regulating gene expression, organizing chromosome architecture, and producing signals that control epigenetic inheritance.2 A major aim of the HGP was to determine the functions of genes. Researchers believed that once the complete genome sequence was developed, interpreting the sequence by comparing the intermediate messenger RNA and protein products would be straightforward and ultimately would identify the genetic factors that influence important phenotypes such as predisposition to certain diseases. The simple rationale behind GWAS is that if certain genetic variations are more frequent in persons with a given disease, the variations are said to be “associated” with the disease. The associated genetic variations serve as pointers to regions of the human genome that may be involved in causing the disease. Genome-Wide Association Studies GWAS compare the DNA of two groups of participants: subjects with the phenotype of interest (cases, or persons with a particular disease) and similar subjects without the phenotype (controls). Each subject provides a sample of DNA, from which millions of genetic variants are read using single Overview of Chapters 3 polymorphism (SNP) arrays. If one type of the variant (one allele, i.e., the “wild- type” allele) is more frequent in people with the disease, the SNP is said to be associated with the disease. The associated SNPs are then considered to mark a region of the human genome that influences the risk of the phenotype. Also, in contrast to methods which specifically test one or a few genetic regions, GWAS investigate the entire genome. The approach is therefore said to be non–candidate driven, in contrast to gene-specific candidate-driven studies. GWAS identify tag SNPs, which are defined as representative SNPs in a region of the genome with high linkage disequilibrium and other variants in DNA associated with a disease. Tag SNPs in isolation cannot specify which genes cause the phenotype. The first successful GWAS investigated age-related macular degeneration and was published in 2005.3 This study found two SNPs that had significantly altered allele frequency when compared with healthy controls. As of 2015, The Catalog of Published Genome-Wide Association Studies contained more than 2,141 catalog entries, 1,856 publications and 12,874 implicated SNPs.4 Prior to the introduction of GWAS, the major method of investigation was via genetic linkage studies in families. This approach was useful for identifying single-gene disorders, many of which appear in the comprehensive compendium of human genes and genetic phenotypes, the Online Mendelian Inheritance in Man (OMIM) database.5 However, for both common and complex diseases, the results of genetic linkage studies have been hard to reproduce.6,7 In contrast, GWAS seek to identify whether the allele of a genetic variant is found more often than expected in individuals with the phenotype of interest. The statistical methods used in GWAS are based on traditional approaches, and early calculations of statistical power indicated that GWAS could be better than linkage studies at detecting weak genetic effects.8 In addition to a simple conceptual framework, the proliferation of GWAS has also been driven by improvements in sequencing methods, reduced computational costs, and the advent of biobanks, which are repositories of human genetic material that greatly reduce the cost and difficulty of collecting sufficient numbers of biological specimens for study.9,10 The development of rapid genome-level sequencing techniques also permits researchers to assess methods to mine this information to identify genetic associations with disease
Details
-
File Typepdf
-
Upload Time-
-
Content LanguagesEnglish
-
Upload UserAnonymous/Not logged-in
-
File Pages161 Page
-
File Size-