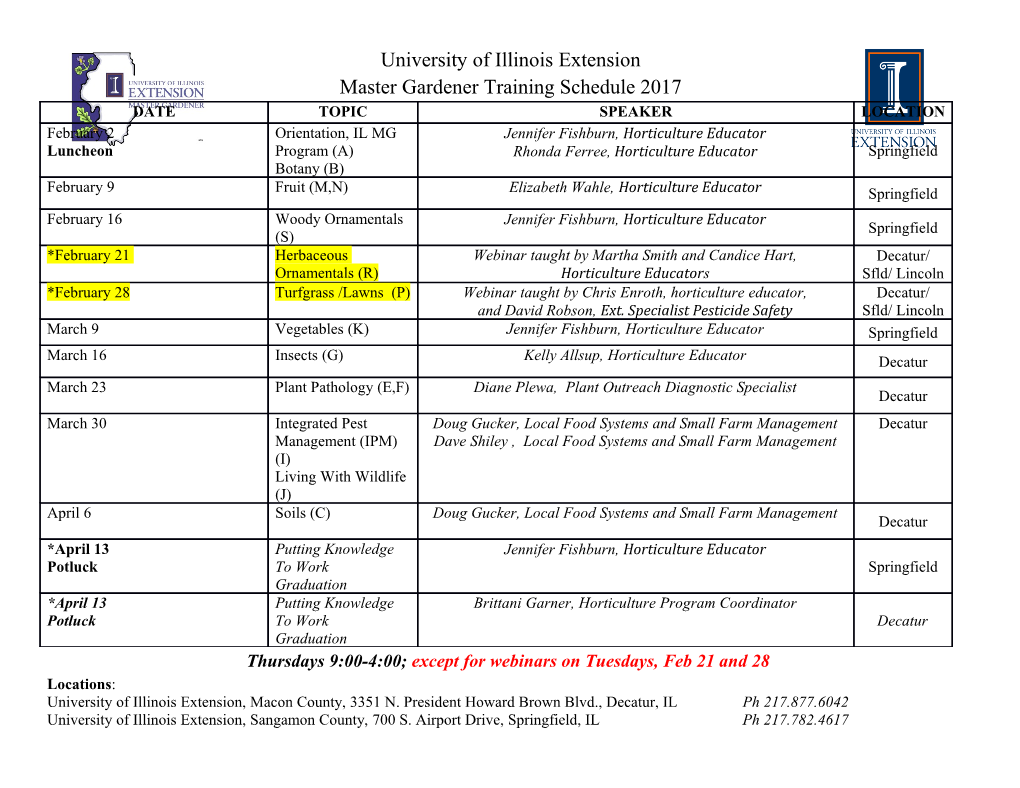
00˙AMS June 18, 2007 Optimization Algorithms on Matrix Manifolds 00˙AMS June 18, 2007 00˙AMS June 18, 2007 Optimization Algorithms on Matrix Manifolds P.-A. Absil Robert Mahony Rodolphe Sepulchre PRINCETON UNIVERSITY PRESS PRINCETON AND OXFORD 00˙AMS June 18, 2007 Copyright c 2008 by Princeton University Press Published by Princeton University Press 41 William Street, Princeton, New Jersey 08540 In the United Kingdom: Princeton University Press 3 Market Place, Woodstock, Oxfordshire OX20 1SY All Rights Reserved ISBN-13: 978-0-691-13298-3 ISBN-10: 0-691-13298-4 British Library Cataloging-in-Publication Data is available This book has been composed in Computer Modern in LATEX The publisher would like to acknowledge the authors of this volume for providing the camera-ready copy from which this book was printed. Printed on acid-free paper. ∞ press.princeton.edu Printed in the United States of America 10 9 8 7 6 5 4 3 2 1 00˙AMS June 18, 2007 To our parents 00˙AMS June 18, 2007 00˙AMS June 18, 2007 Contents Foreword, by Paul Van Dooren xi Notation conventions xiii 1. Introduction 1 2. Motivation and Applications 5 2.1 A case study: the eigenvalue problem 5 2.1.1 The eigenvalue problem as an optimization problem 7 2.1.2 Some benefits of an optimization framework 9 2.2 Research problems 10 2.2.1 Singular value problem 10 2.2.2 Matrix approximations 12 2.2.3 Independent component analysis 13 2.2.4 Pose estimation and motion recovery 14 2.3 Notes and references 16 3. Matrix Manifolds: First-Order Geometry 17 3.1 Manifolds 18 3.1.1 Definitions: charts, atlases, manifolds 18 3.1.2 The topology of a manifold* 20 3.1.3 How to recognize a manifold 21 3.1.4 Vector spaces as manifolds 22 n×p n×p 3.1.5 The manifolds R and R∗ 22 3.1.6 Product manifolds 23 3.2 Differentiable functions 24 3.2.1 Immersions and submersions 24 3.3 Embedded submanifolds 25 3.3.1 General theory 25 3.3.2 The Stiefel manifold 26 3.4 Quotient manifolds 27 3.4.1 Theory of quotient manifolds 27 3.4.2 Functions on quotient manifolds 29 3.4.3 The real projective space RPn−1 30 3.4.4 The Grassmann manifold Grass(p, n) 30 3.5 Tangent vectors and differential maps 32 3.5.1 Tangent vectors 33 3.5.2 Tangent vectors to a vector space 35 00˙AMS June 18, 2007 viii CONTENTS 3.5.3 Tangent bundle 36 3.5.4 Vector fields 36 3.5.5 Tangent vectors as derivations∗ 37 3.5.6 Differential of a mapping 38 3.5.7 Tangent vectors to embedded submanifolds 39 3.5.8 Tangent vectors to quotient manifolds 42 3.6 Riemannian metric, distance, and gradients 45 3.6.1 Riemannian submanifolds 47 3.6.2 Riemannian quotient manifolds 48 3.7 Notes and References 51 4. Line-Search Algorithms on Manifolds 54 4.1 Retractions 54 4.1.1 Retractions on embedded submanifolds 56 4.1.2 Retractions on quotient manifolds 59 4.1.3 Retractions and local coordinates* 61 4.2 Line-search methods 62 4.3 Convergence analysis 63 4.3.1 Convergence on manifolds 63 4.3.2 A topological curiosity* 64 4.3.3 Convergence of line-search methods 65 4.4 Stability of fixed points 66 4.5 Speed of convergence 68 4.5.1 Order of convergence 68 4.5.2 Rate of convergence of line-search methods* 70 4.6 Rayleigh quotient minimization on the sphere 73 4.6.1 Cost function and gradient calculation 74 4.6.2 Critical points of the Rayleigh quotient 74 4.6.3 Armijo line search 76 4.6.4 Exact line search 78 4.6.5 Accelerated line search: Locally optimal conjugate gradient 78 4.6.6 Links with the power method and inverse iteration 78 4.7 Refining eigenvector estimates 80 4.8 Brockett cost function on the Stiefel manifold 80 4.8.1 Cost function and search direction 80 4.8.2 Critical points 81 4.9 Rayleigh quotient minimization on the Grassmann manifold 83 4.9.1 Cost function and gradient calculation 83 4.9.2 Line-search algorithm 85 4.10 Notes and references 86 5. Matrix Manifolds: Second-Order Geometry 91 5.1 Newton’s method in Rn 91 5.2 Affine connections 93 5.3 Riemannian Connection 96 5.3.1 Symmetric connections 96 5.3.2 Definition of the Riemannian connection 97 5.3.3 Riemannian connection on Riemannian submanifolds 98 5.3.4 Riemannian connection on quotient manifolds 100 00˙AMS June 18, 2007 CONTENTS ix 5.4 Geodesics, exponential mapping, and parallel translation 101 5.5 Riemannian Hessian operator 104 5.6 Second covariant derivative* 108 5.7 Notes and references 110 6. Newton’s Method 111 6.1 Newton’s method on manifolds 111 6.2 Riemannian Newton method for real-valued functions 113 6.3 Local convergence 114 6.3.1 Calculus approach to local convergence analysis 117 6.4 Rayleigh quotient algorithms 118 6.4.1 Rayleigh quotient on the sphere 118 6.4.2 Rayleigh quotient on the Grassmann manifold 120 6.4.3 Generalized eigenvalue problem 121 6.4.4 The nonsymmetric eigenvalue problem 125 6.4.5 Newton with subspace acceleration: the Jacobi-Davidson approach 126 6.5 Analysis of Rayleigh quotient algorithms 128 6.5.1 Convergence analysis 128 6.5.2 Numerical implementation 129 6.6 Notes and references 131 7. Trust-Region Methods 136 7.1 Models 137 7.1.1 Models in Rn 137 7.1.2 Models in general Euclidean spaces 137 7.1.3 Models on Riemannian manifolds 138 7.2 Trust-region methods 140 7.2.1 Trust-region methods in Rn 140 7.2.2 Trust-region methods on Riemannian manifolds 140 7.3 Computing a trust-region step 141 7.3.1 Computing a nearly exact solution 142 7.3.2 Improving on the Cauchy point 143 7.4 Convergence analysis 145 7.4.1 Global convergence 145 7.4.2 Local convergence 152 7.4.3 Discussion 158 7.5 Applications 159 7.5.1 Checklist 159 7.5.2 Symmetric eigenvalue decomposition 160 7.5.3 Computing an extreme eigenspace 161 7.6 Notes and references 166 8. A Constellation of Superlinear Algorithms 168 8.1 Vector transport 168 8.1.1 Vector transport and affine connections 170 8.1.2 Vector transport by differentiated retraction 172 8.1.3 Vector transport on Riemannian submanifolds 174 8.1.4 Vector transport on quotient manifolds 174 8.2 Approximate Newton methods 175 00˙AMS June 18, 2007 x CONTENTS 8.2.1 Finite difference approximations 176 8.2.2 Secant methods 178 8.3 Conjugate gradients 180 8.3.1 Application: Rayleigh quotient minimization 183 8.4 Least-square methods 184 8.4.1 Gauss-Newton methods 186 8.4.2 Levenberg-Marquardt methods 187 8.5 Notes and references 188 A. Elements of Linear Algebra, Topology, and Calculus 189 A.1 Linear algebra 189 A.2 Topology 191 A.3 Functions 193 A.4 Asymptotic notation 194 A.5 Derivatives 195 A.6 Taylor’s formula 198 Bibliography 200 Index 221 00˙AMS June 18, 2007 Foreword Constrained optimization is quite well established as an area of research, and there exist several powerful techniques that address general problems in that area. In this book a special class of constraints is considered, called geomet- ric constraints, which express that the solution of the optimization problem lies on a manifold. This is a recent area of research that provides powerful alternatives to the more general constrained optimization methods. Clas- sical constrained optimization techniques work in an embedded space that can be of a much larger dimension than that of the manifold. Optimization algorithms that work on the manifold have therefore a lower complexity and quite often also have better numerical properties (see, e.g., the numerical integration schemes that preserve invariants such as energy). The authors refer to this as unconstrained optimization in a constrained search space. The idea that one can describe difference or differential equations whose solution lies on a manifold originated in the work of Brockett, Flaschka, and Rutishauser. They described, for example, isospectral flows that yield time-varying matrices which are all similar to each other and eventually converge to diagonal matrices of ordered eigenvalues. These ideas did not get as much attention in the numerical linear algebra community as in the area of dynamical systems because the resulting difference and differential equations did not lead immediately to efficient algorithmic implementations. An important book synthesizing several of these ideas is Optimization and Dynamical Systems (Springer, 1994), by Helmke and Moore, which focuses on dynamical systems related to gradient flows that converge exponentially to a stationary point that is the solution of some optimization problem. The corresponding discrete-time version of this algorithm would then have linear convergence, which seldom compares favorably with state-of-the-art eigenvalue solvers. The formulation of higher-order optimization methods on manifolds grew out of these ideas. Some of the people that applied these techniques to basic linear algebra problems include Absil, Arias, Chu, Dehaene, Edelman, El- den, Gallivan, Helmke, H¨uper, Lippert, Mahony, Manton, Moore, Sepulchre, Smith, and Van Dooren. It is interesting to see, on the other hand, that several basic ideas in this area were also proposed by Luenberger and Gabay in the optimization literature in the early 1980s, and this without any use of dynamical systems.
Details
-
File Typepdf
-
Upload Time-
-
Content LanguagesEnglish
-
Upload UserAnonymous/Not logged-in
-
File Pages241 Page
-
File Size-