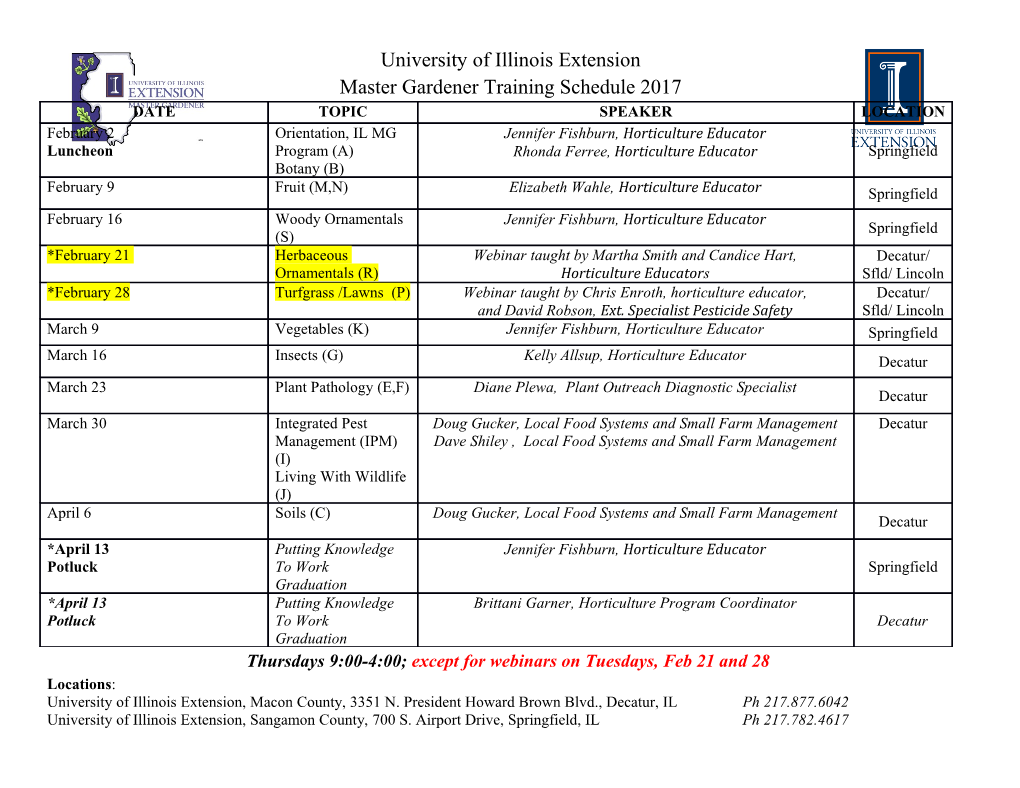
Tuan V. Nguyen Gene$cs Epidemiology of Osteoporosis Lab Garvan Ins$tute of Medical Research Garvan Ins$tute Biostas$cal Workshop 17 April 2014 © Tuan V. Nguyen Introduction to R • A brief history • Installaon • Packages • Essen$al grammar • A session with R Previously … • Many stas$cal packages were/are available • Popular packages include Systat, Minitab, Stas$ca, BMDP, S+, Gauss, Spida JMP, SPSS, Stata, SAS and now R R is gaining popularity Number of scholarly ar$cles that reference each soUware by year (Source: Muenchen R. The popularity of data analysis soUware, r4stat.com/ar$cles/popularity) R is gaining popularity Number of scholarly ar$cles that reference each soUware by year, aer removing the top two, SPSS and SAS (Source: Muenchen R. The popularity of data analysis soUware, r4stat.com/ar$cles/popularity) A brief history • R is a “stas$cal and graphical programming language” • Originated from S – 1988 - S2: RA Becker, JM Chambers, A Wilks – 1992 - S3: JM Chambers, TJ Has$e – 1998 - S4: JM Chambers • R was ini$ally wriben by Ross Ihaka and Robert Gentleman (Univ of Auckland, New Zealand) in 1990s • From 1997: internaonal “R-core”, 15 people What can R do? • It is a sta$s$cal language • All models of stas$cal analysis • Great for simulaon work • Programming (do you want to take a challenge?) Why R ? • Open source – totally free! • Developed by professional and academic stas$cians • Run on Windows, Unix, MacOS • Keep up-to-date with methodological developments • Speak the language of experts (bioinformacs and stas$cs) • Large user community Installaon cran.r-project.org Installation of R on Windows • Select Windows • Select “base” • Run à OK à Next • Then Finish – R icon on your desktop A screenshot of R RStudio An “add-on” of R RStudio hbp://rstudio.org Introduction to RStudio • An IDE (Interface Development Environment) of R. • Provide some convenient func$ons for running R • R also has a number of other IDEs: • TinnR • R commander R and RStudio Can run R within Rstudio (you don’t need to start R) RStudio Workspace: Variables R console Files Packages R is a real demonstration of the power of collaboration Ihaka Packages • R = Base + Packages • Base R includes basic R func$ons for simple func$ons and analyses • Packages are modules for specific analyses • More than 6000 packages in R ! Common packages Hmisc: Miscellaneous for data rms: Regression modeling strategies manipulaon car: Companion to regression tables: For tabulaon of data analysis foreign: For reading data from survival: Survival analyses other soUwares EpiR: Epidemiological analyses tables: For tabulaon of data epicalc: Epidemiological analyses gmodels: Programming tools boot: Bootstrap analyses ggplot2: Advanced graphics cluster: Cluster analysis sciplot: Scien$fic graphs psych: Psychometrics and Zelig: “Every one’s stas$cal descrip$ve stas$cs soUware” Basic management of packages • Installing new packages (try now!) install.packages(c("Hmisc", "rms", "tables", "foreign", "gmodels", "ggplot2", "sciplot", "Zelig", "car", "survival", "EpiR", "epicalc", "boot", "cluster", "psych", "binom", "BMA", "ExactCIdiff", "lattice", "mgcv", "gam", "nlme", "quantreg") • To find out which packages you have installed library() R Grammar: a quick introduc9on Interacting with R • Start up R • Can use up/down arrow keys to retrieve command history • Can use leU/right keys to edit a command line • Can use TAB to append a full command – very useful! • Mul$ple commands can be wriben in 1 line by using “;” separator Variable names • Use lebers, numbers, and signs (., -, _) • Assignment symbol: <- or = • Dis$nc$on between upper and lower case lebers Genotype = 5; genotype <- 7; Geno.type = Genotype + genotype Object-oriented language R is an object-oriented language • Funcon • Vector • Matrix • Dataframe Function • R “commands” = func$on • Func$on has arguments • Arguments include variables (name), parameters, opons, etc • Example: fing a linear regression model y = a + bx m1 = lm(y ~ x, data=test) Function • R “commands” = func$on • Func$on has arguments • Example: fing a linear regression model y = a + bx m1 = lm(y ~ x, data=test) Object name Func9on Arguments: m1 lm = linear model variables: y, x dataset name Vector • Vectors are basic building block in R • Vector = a series of values • Values can be numeric or character score = c(4,2,1,5) gender = c('F','M','F','M') c (concatenaon) for direct data entry Matrix • Rectagular data à rows, columns • Matrix can be a collec$on of vectors 1 3 6 7 3 4 7 9 5 7 8 0 Matrix 1 3 6 7 3 4 7 9 5 7 8 0 v1 = c(1,3,5) v2 = c(3,4,7) v3 = c(6,7,8) v4 = c(7,9,0) m = cbind(v1,v2,v3,v4) m Reference to matrix > m • Row first, column later v1 v2 v3 v4 [1,] 1 3 6 7 • Flexible in R [2,] 3 4 7 9 [3,] 5 7 8 0 > m[2,3] v3 7 > m[,2:3] v2 v3 > m[1,] [1,] 3 6 v1 v2 v3 v4 [2,] 4 7 1 3 6 7 [3,] 7 8 > m[1:2,] > m[,3:4]*m[1,2] v1 v2 v3 v4 v3 v4 [1,] 1 3 6 7 [1,] 18 21 [2,] 3 4 7 9 [2,] 21 27 [3,] 24 0 Dataframe Dataset in R = “Dataframe” = matrix fields, columns, variables ID Gender Math Reading 1 F 5 8 2 M 5 2 rows records 3 F 7 3 observaons 4 F 8 6 numeric character numeric numeric Reference to field/column in a dataframe • Dataframe should be attached prior to analysis • Reference to field: (dataframe name)$(field name) • Example: v1 = c(1,3,5) v2 = c(3,4,7) v3 = c(6,7,8) v4 = c(7,9,0) dat = data.frame(v1, v2, v3, v4) attach(dat) dat$sum = dat$v1 + dat$v3 sum1 = v1 + v3 dat The effect of $ v1 = c(1,3,5) > dat v2 = c(3,4,7) v1 v2 v3 v4 sum v3 = c(6,7,8) 1 1 3 6 7 7 v4 = c(7,9,0) 2 3 4 7 9 10 dat=data.frame(v1,v2,v3,v4) 3 5 7 8 0 13 attach(dat) dat$sum = dat$v1 + dat$v3 There is NO sum1 ! sum1 = v1 + v3 dat Data coding in R id = c(1, 2, 3, 4, 5) gender = c("male", "female", "male", "female", "female") dat = data.frame(id, gender) We want to create a new variable called sex with numeric values (1, 2) dat$sex[gender=="male"] <- 1 dat$sex[gender=="female"] <- 2 Character and numeric coding Character to numeric X = c("1", "2", "3", "4", "5") We want to create a new variable called Y with numeric values (for calculaon) Y = as.numeric(X) mean(Y) Numeric to character Y = 1:10 We want to create a new variable called X with character values X = as.character(Y) Sorting dat: sort() X = rnorm(10); X [1] 1.5651300 -0.5382971 -0.1995302 1.0111098 0.3590144 -1.5245237 [7] -0.3192534 0.1323256 -0.7916954 -0.0664167 sort(X) [1] -1.5245237 -0.7916954 -0.5382971 -0.3192534 -0.1995302 -0.0664167 [7] 0.1323256 0.3590144 1.0111098 1.5651300 Merging datasets id = c(1,2,3,4) id = c(1,2,3,4,5) sex=c("M","F","M","F") age=c(21,34,45,32,18) dat1=data.frame(id,sex) dat2=data.frame(id,age) dat = merge(dat1, dat2, by="id") dat = merge(dat1, dat2, by="id", all.x=T, all.y=T) An R Session (demo) To work with R … • R, like most stas$cal programs, works on observaons (rows) and variables • You should keep in mind – Name of dataframe – Name of variables Allison and Cichhetti’s study Trueb Allison; Domenic V. Cicche. Sleep in Mammals: Ecological and Cons$tu$onal Correlates. Science 1976; 194:732-734. R Session • Reading a file into R for analysis Filename: allison.csv • Some graphical analyses • Some descrip$ve (and not so descrip$ve) analyses Allison T, Cicchetti DV (1976). Sleep in mammals: ecological and constitutional correlates. Science 194, 732–734. NonDrea Species BodyWt BrainWt ming Dreaming TotalSleep LifeSpan Gestaon Predaon Exposure Danger Africanelephant 6654 5712 NA NA 3.3 38.6 645 3 5 3 Africangiantpouchedrat 1 6.6 6.3 2 8.3 4.5 42 3 1 3 ArccFox 3.385 44.5 NA NA 12.5 14 60 1 1 1 Arccgroundsquirrel 0.92 5.7 NA NA 16.5 NA 25 5 2 3 Asianelephant 2547 4603 2.1 1.8 3.9 69 624 3 5 4 Baboon 10.55 179.5 9.1 0.7 9.8 27 180 4 4 4 Bigbrownbat 0.023 0.3 15.8 3.9 19.7 19 35 1 1 1 Braziliantapir 160 169 5.2 1 6.2 30.4 392 4 5 4 Cat 3.3 25.6 10.9 3.6 14.5 28 63 1 2 1 Chimpanzee 52.16 440 8.3 1.4 9.7 50 230 1 1 1 Chinchilla 0.425 6.4 11 1.5 12.5 7 112 5 4 4 Cow 465 423 3.2 0.7 3.9 30 281 5 5 5 Deserthedgehog 0.55 2.4 7.6 2.7 10.3 NA NA 2 1 2 Donkey 187.1 419 NA NA 3.1 40 365 5 5 5 EasternAmericanmole 0.075 1.2 6.3 2.1 8.4 3.5 42 1 1 1 Reading file csv • Locate your folder and filename • Use the func$on read.csv • In Mac, you simply drag the filename to the R command line dat = read.csv("~/Dropbox/Garvan Lectures 2014/Datasets and Teaching Materials/ allison.csv", header=T, na.strings="NA") Reading file through file.choose() f = file.choose() # find the file dat = read.csv(f, header=T, na.strings="NA") attach(dat) # aach the data before analysis names(dat) # want to know variable names dim(dat) # how many rows and columns? summary(dat) # summarize data Summary: an overall “picture” > summary(dat) Species BodyWt BrainWt Africanelephant : 1 Min.
Details
-
File Typepdf
-
Upload Time-
-
Content LanguagesEnglish
-
Upload UserAnonymous/Not logged-in
-
File Pages69 Page
-
File Size-