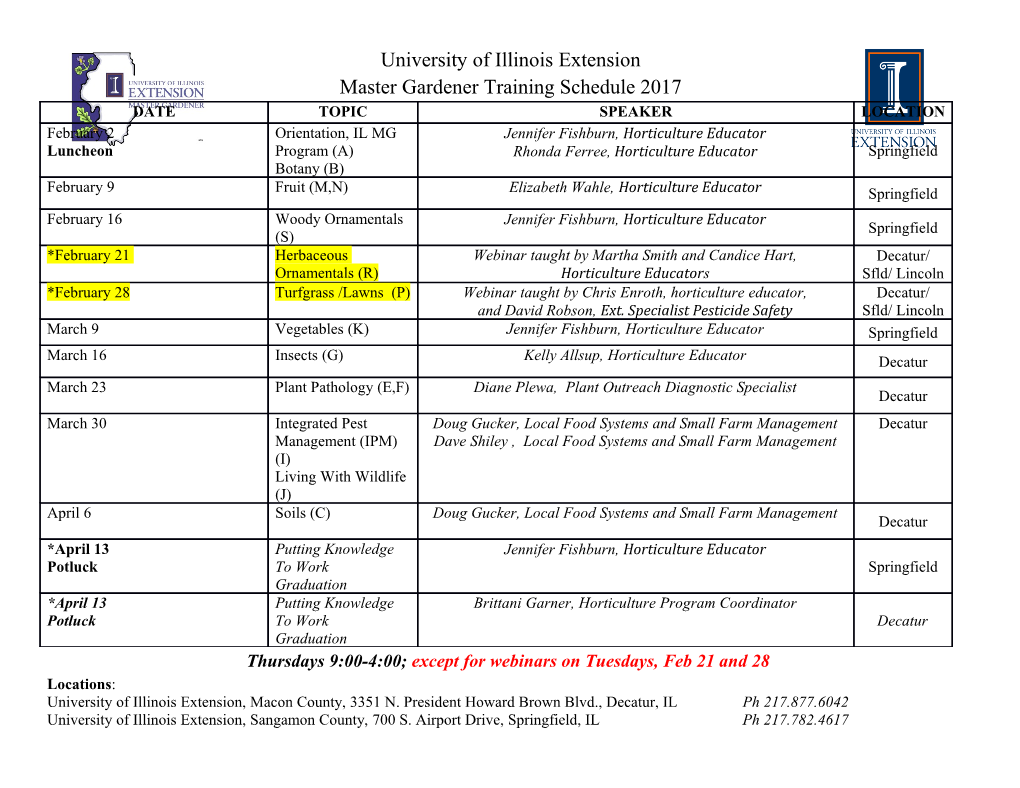
982 JOURNAL OF SOFTWARE, VOL. 7, NO. 5, MAY 2012 Integer Wavelet Image Denoising Method Based on Principle Component Analysis Jing Peng College of Computer and Information Science, Chongqing Normal University, Chongqing, China Email: [email protected] Yan Ma College of Computer and Information Science, Chongqing Normal University, Chongqing, China Email: [email protected] Abstract—Over the years a variety of methods have been of denoising algorithms local-based spatial methods. introduced to remove noise from digital images, such as Whereas, generally speaking, the information encoded in Gaussian filtering, anisotropic filtering, and Total Variation a natural image is redundant to some extent, that is to say, minimization. However, many of these algorithms remove there may exist some repeat patterns in natural images, the fine details and structure of the image in addition to the noise because of assumptions made about the frequency particularly in textured or periodic case. Based on this content of the image. It is analyzed in the way that the noise observation, Buadest [6] developed a non-local image is decomposed into low and high frequency sub-band under denoising algorithm which takes full advantage of image the wavelet transformation, and subconsequently extracts redundancy. Like many noise reduction algorithm, the the principle components feature with the method of method is also based on weighted average. The essence Principle Component Analysis(PCA). This can keep the the of the method is: to estimate a certain pixel, the method picture as detailed as possible, while at the same time getting uses the similarities between it and all the other pixels in rid of the noise. This exeperiment proves that this method image to act as weight, and the similarities are not can get rid of the noise of the picture not only effectively, computed from pixels themselves but from their but also keeps the detail of the picture to the maximum. neighboring window (compare window). The algorithm Index Terms—image denoising, integer wavelet, Principle has demonstrated strong superiority over local-based Component Analysi spatial methods such as Gaussian filter, bilateral filter in terms of both PSNR and visual quality. However, certain critical issues need further investigation. Firstly, the I. INTRODUCTION computational cost is high for its pixel-by-pixel window matching, and it limits the method to be widely used in With the prevalence of digital cameras and scanners, application. Secondly, the quality and computation cost digital images can be easily acquired nowadays. of the algorithm is closely related to the size of compare Unfortunately, digital images are often degraded by noise window which represents geometrical configuration of during acquisition or transmission process. Various pixel’s neighboring region. For small conpare window, image-related applications, such as medical image the algorithm is restricted to suppressing the high- analysis, image segmentation, and object detection, etc., frequency noise and cannot remove the low-frequency generally require effective noise suppression method to noise (1arge-scale). For large compare window, the further produce reliable results. Therefore, image algorithm removes the low-frequency noise effectively, denoising has been one of the most important and widely but becomes less sensitive to small details and the studied problems in image processing and computer additional burden on the computational resources vision. So far, image denoising methods can be basically becomes unacceptable. divided into two categories: spatial filtering methods [1- Since the essential goal of denoising is to preserve the 3] and transform domain filtering methods [4-5] image features while reducing noise effectively, a logical In spatial domain, for every pixel of noisy image, extension of spatial denoising is to transform images into many existing noise reduction methods employ the a representation that distinctive features such as edges information of its nearby local region to estimate its can be extracted from image and perform noise reduction denoised version. Examples include Gaussian filter, algorithms in this domain. As for denoising in frequency median filter, bilateral filter and so on. We call these kind domain, we are more familiar with wavelets which fall into two classes: orthogonal wavelet and non-orthogonal Manuscript received January 1, 2011; revised June 1, 2011; accepted wavelet. Orthogonal wavelet compositions are critically July 1, 2011. sampled (i.e., nonredundant) image descriptions. It has been successfully used in image compression and threshold-based image denoising. However, the non- © 2012 ACADEMY PUBLISHER doi:10.4304/jsw.7.5.982-989 JOURNAL OF SOFTWARE, VOL. 7, NO. 5, MAY 2012 983 redundant property of orthogonal wavelet means they are a ratio of quantities and units. Figure labels should be not suitable for non-local means denoising which builds legible, about 9-point type. upon image redundancy. Color figures will be appearing only in online Actually, the various methods about image, which publication. All figures will be black and white graphs in eliminate noise, are going to trade-off between print publication. eliminating and keeping useful high-frequency 1) Split information. Major denoising methods include Gaussian The primitive signal split into two sub-sets that not filtering, Wiener filtering, and wavelet thresholding. each other crossing in this course: Even sample and odd Many more methods have been developed; however, sample S S most methods make assumptions about the image that can j ,2l j,2l +1 lead to blurring. S j,2l =even( S j ) (1) PCA(Principle Component Analysis)[6-7] technology on the basis of the neural network method, because the S j,2l+1 =odd( S j ) (2) altitudinal parallelism and adaptability, it can extract the main characteristic component of the image, thus 2) Predict eliminate the noise signal of the main characteristic During the processing of predicting, in order to keep component. However, if we extract the main character of the even sample S j,2l fixedness, we can aim at the image space domain with PCA directly, it will lose the intrinsic existing detail of the image while suppressing dependence among initial data, and adopt a prediction the noise probably. So, this papper propose to change the operator which having nothing to do with datum structure image from space domain to the frequency domain P , making use of even sample S j,2l to predict the odd through frequency transformation, low frequency and sample S j,2l+1 , and substitute the odd number sample high-frequency signal are gained after resolving firstly. S The noise signal concentrate on the high-frequency signal with the odd sample j,2l+1 minus predicted value more at this moment, and extract the main characteristic (called detail coefficient ), predict that the expression to low frequency and high-frequency signal separately. formula of the course is as follows: Then inversed transformation goes back to the space domain, it can realize that getting rid of the noise, and d j−1 = S j,2l+1 - P( S j,2l ) (3) will keep the detail part of more images. While going on to the image - the method to vary frequently can select 3) Update the wavelet of integer transformation, and it is harmless Because the 1st step of even signals produced is not that it can enable resolving. the same as initial data on some whole qualities, so need to adopt newer course. Newer starting point to find one II. INTEGER WAVELET TRANSFORM good sub datum set S j−1 , and make it keep some It is all the varying of real number fields that the characteristics which initial data S j collected, for traditional wavelete is varied, even the digital signal is an integer array, the corresponding wavelete varies instance energy, mean value, disappearance square, etc., coefficient is a real number too. People hope that the namely Q ( S j−1 )=Q ( S j ). So introduce upgrade wavelete transformation of the matrix of the image is the operator U, act on detail coefficient and superpose on integer matrix, namely the wavelete transformation of an the even sample , receive the signal similarly, integer of integer, which array integer is mapping into integer wavelet coefficient, and reversible, known as " S S d j−1 = j,2l + j−1 integer wavelete vary " (IWT) usually[7-9]. U ( ) (4) A. Lifting Scheme S Here predict wave filter P( j,2l )= ∑ p j,l s j,2l , Sweldens [7-9] put forward the new construct of l d wavelete, which not depend on Fourier transformation - - update filter U( j−1 ) = ∑u j−1,l d j−1,2l+1 , int [x] is lifting scheme, this method can not only keeps intrinsic l existing of wavelete, but also overcome the limitation fetched whole to the thing that x rounds up. Lifting from inflexibility of translation and flexion. The second algorithms that can be apt to realize from integer to generation of wavelete transformation which based on integer mapping of wavelete transformation. And the advanced method, has the fast algorithm with wavelete, it course is all reversible. can realize transformation from integer to the integer, and This processing is called a lifting scheme of wavelete carrying on the entropy code to the data after varying can transformation, and the lowpass component S j−1 and realize the harmless compression of the picture. On the d basis of lifting integer wavelet method is including 3 highpass j−1 are exported, the sub-band result after steps, viz. split, predict and update. often some wavelete transformation still need to carry on To figure axis labels, use words rather than symbols. deeper resolving further. Can be according to the request Do not label axes only with units. Do not label axes with of state of the network and user (resolution ratio of users' display device, computing capability, etc.) come to adjust © 2012 ACADEMY PUBLISHER 984 JOURNAL OF SOFTWARE, VOL.
Details
-
File Typepdf
-
Upload Time-
-
Content LanguagesEnglish
-
Upload UserAnonymous/Not logged-in
-
File Pages8 Page
-
File Size-