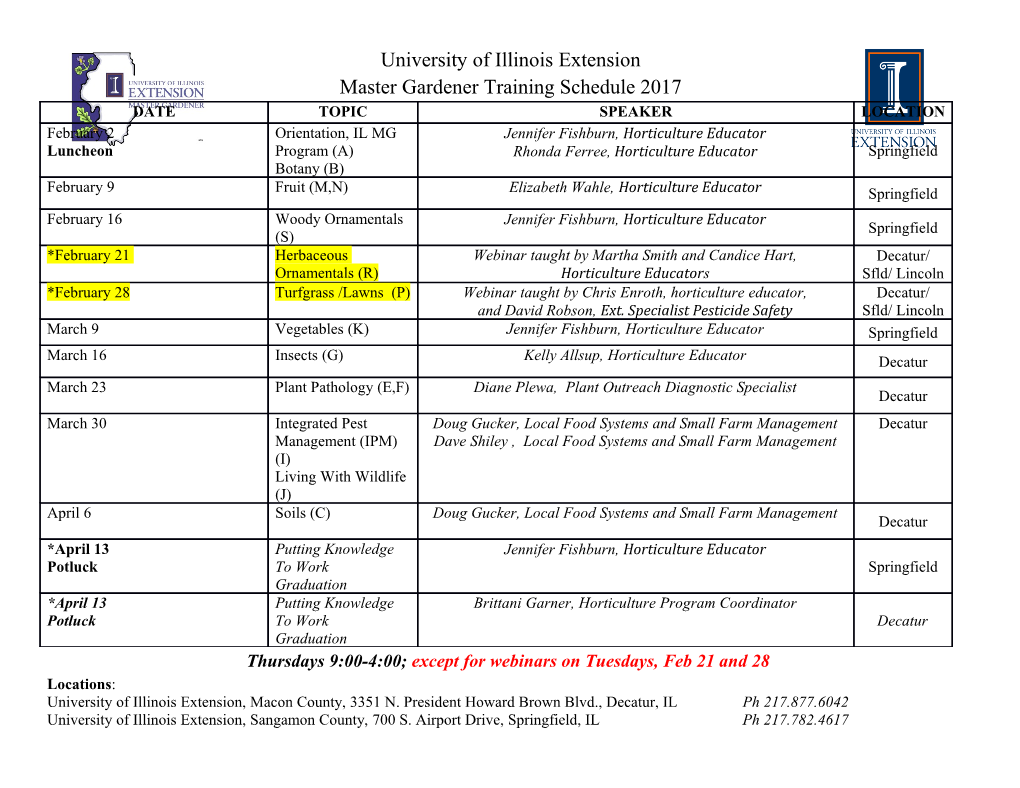
Review of Deep Reinforcement Learning Algorithms Alexandre Berthet Master Ingénierie de l’Informatique Industriel et de l’Image, Sorbonne Université Data Scientist, IBM France 5 place Jussieu, 75005, Paris, France [email protected] Abstract - Since the beginning of the years of Big Data, a particular area of Artificial Intelli- 2010, achievements in reinforcement learning gence is on the rise : Machine Learning. Actually, haven’t stop to emerge. Particularly, a lot of breakthroughs has emerged one after the other since new algorithms derived from the merge be- the 21th century. Moreover, there are plenty of tween Deep Learning and Reinforcement Learn- articles, researches or applications about this field. ing have proved their effectiveness. The biggest Machine Learning is divided in three principal cat- demonstrations that have been effectuated were egories : supervised learning, unsupervised learning on Atari Games and Dota2 thanks to algorithms and reinforcement learning [2] (in fact there is one developed respectively by DeepMind and Open more : semi-supervised learning). The first two are AI. Indeed, in both cases, human being were de- often described like the two main types of tasks in feated. This paper tried to give the explanations machine learning and overall opposed. Effectively, behind these breakthroughs. supervised learning needs labeled data, gives feed- back and is used to predict a result whereas the aim Key words - Reinforcement Learning, of the other is to find some connections between Value Iteration, Policy Iteration, Q-learning, data without any labels or feedback. Reinforcement Deep Reinforcement Learning, Convolutional learning has a very different approach from the Neural Network, Deep Q-Network, Proximal others : the goal is to teach to an agent how to Policy Optimization, Policy Update. act in an environment. I. INTRODUCTION First of all, this paper will detail the re- inforcement learning’s basics such as the relation to Markov Decision Process or the difference be- The notion of Artificial Intelligence is ap- tween model-based and model-free learning. Then, peared with Alain Turing and his well-known "Test it presents algorithms used by DeepMind and Ope- of Turing" to determine if a computer can mimic nAI which are the principal actors of deep rein- a human conversation. This test is exhaustively forcement learning. explained in the article "Computing Machinery and Intelligence" published in Mind (1950) [1]. II. REINFORCEMENT LEARNING Methods and algorithms have evolved constantly since this memorable paper but the concept of Artificial Intelligence have always been the same : A. EXPLORATION AND EXPLOITATION it’s intelligence demonstrated by machines. With the Reinforcement learning is a kind of learning development of data collection and the phenomenon based on a couple action-reward and inspired from animals or human learning. For example, when a into account all the previous actions and satisfies baby begin to walk, his natural instinct push him himself with the reward known. However, in the to go everywhere and test everything. The result two cases the choice isn’t optimal. In fact, the best of these actions can be beneficial or not and kids solution is to do a mix between exploration and will learn like that. In fact, reinforcement learning exploitation to get the ideal goal. An algorithm is built with the same approach and can be define illustrates that : "-greedy [4]. Basically, greedy easily with five terms : algorithms are described like taking the best action 1) agent : the one which learn at the T moment. This one inserts a randomness in 2) environment : where the agent evolve (real or his decision which is represented by the value " (2 virtual) [0, 1]). Effectively, whether take the best choice for 3) state : position of the agent in the environment a probability 1 - " whether make a new action for a 4) action : what the agent does from a state probability ". So, the higher is " the more the agent 5) reward : feedback of an action from a state explores his environment. According to experiments of Scientists, it should be high at the beginning and In fact, whatever the action taken by an agent from decrease progressively. Precisely, the sequence of a state, a reward will be given to him by the these choices represents the evolution of an agent environment and it gives an information about how in his environment and it matches perfectly to a this action was : positive, negative or null [3]. concept called Markov Decision Process. B. MARKOV DECISION PROCESS A Markov Decision Process is defined as a discrete time stochastic control process : it’s a framework that helps an agent to make choice. In fact, a Markov Decision Process is built with a finite sets of states S and actions, a probability Pa(s, s’) to take an action a in state s (at time t) which lead to state s’ (at time t+1) and a reward Ra(s, s’) to go from state s to state s’ with an action a [5]. These parameters sounds familiar because there are closed Figure 1 : Typical framing of reinforcement to a model of reinforcement learning. However, the learning scenario policy, i.e. a sequence of actions by state which lead to a final state, and the value function are other important notion of Markov Decision Process. The scenario in Figure 1 shows exactly how an The aim is to find the optimal policy (N.B : similar agent evolves in its environment at each stage. Re- to the ideal goal of reinforcement learning). There inforcement learning aims to maximize this reward. are two methods to solve it, value iteration and The higher it is, the better the agent learns. Two oth- policy iteration, of which approaches are based on ers notions appears with this goal : exploration and different value function. The state-value function exploitation. They can be associated to human being V(s) which indicates the interest to be in a state again. Indeed, when someone wants to eat outside, s and the action-value function which scores the he has two options : go to a restaurant where he fact to take an action a from a state s. The policy knows the food or try another one which may be iteration is the optimization of the policy via state- tasty or not. In one hand the model makes new value function until have the optimal one whereas actions to discover the environment and perhaps the value iteration is obtain the best action-value obtain a huge reward. In the other hand he takes function and then calculate the associated policy. the risk is to learn with something very far from the original environment. In contrast, model-free methods are really closed to reality. Following the previous example, this time, the chess player will make his moves and learn from the result, i.e. his opponent’s move. One of the most known model- free algorithm is Q-learning. C. Q-LEARNING With an algorithm such as Q-learning, the Figure 2 : Algorithm steps of value iteration agent needs to explore his environment and learns from his actions, whether there are positives or not. The basic idea of this method is to calculate the maximum of the expected future rewards for action at each state [6]. Then appears the Q-table which is an important notion of this algorithm. In fact, this table stores the Q-value obtained from each action-state and so it represents more or less the environment. The Bellman equation [7] gives this value from the expected cumulative reward where γ represents the discount rate (γ 2 [0, 1]), i.e. the higher it is the more distant rewards are important. S π t X Q (s; a) = E[γ ∗ Rt+1(s; a)] Figure 3 : Algorithm steps of policy iteration t=0 The first step of the process is to create the Q-table with the following parameters : n columns for the In chess, before making a move, a player number of actions and m rows for the number of imagines the next moves of his opponent (and states and to initialize the whole values to zero. himself), to be sure that he will do the right action. In fact, chess players create a whole succession of actions before taking the first one. The previous algorithms are based on this type of thoughts. From the first results (N.B : initialization values), theses algorithms build a model and use it to simulate the subsequent actions of the agent. Then, the results are returned by applying these actions to the constructed model : it’s called model-based rein- forcement learning. In fact, Reinforcement Learning algorithms based on Markov Decision Process are divided in two parts : model-based and model-free. Whether in chess or with algorithms, model-based methods are built on assumptions and can lead to Figure 4 : Example of a basic environment (left) erroneous results. Indeed, if the model is inaccurate, and its Q-table (right) Then, there is the choice and the achievement of learning, this latter has only one input (the state) and the action. With this part, the concept of exploration give as many outputs (Q-values) as possible actions. and exploitation trade-off comes into play with the The approach of DQN is to give from those outputs "-greedy strategy. As it’s explained previously, at the best action to take basing on the higher value. the beginning, the " parameter will be higher and it will decrease progressively. The logic is that in a first time the agent discover the environment. And in a second time, he will exploit his knowledge to make the best actions. Once the action is chose and performed comes the final step which is the update.
Details
-
File Typepdf
-
Upload Time-
-
Content LanguagesEnglish
-
Upload UserAnonymous/Not logged-in
-
File Pages7 Page
-
File Size-