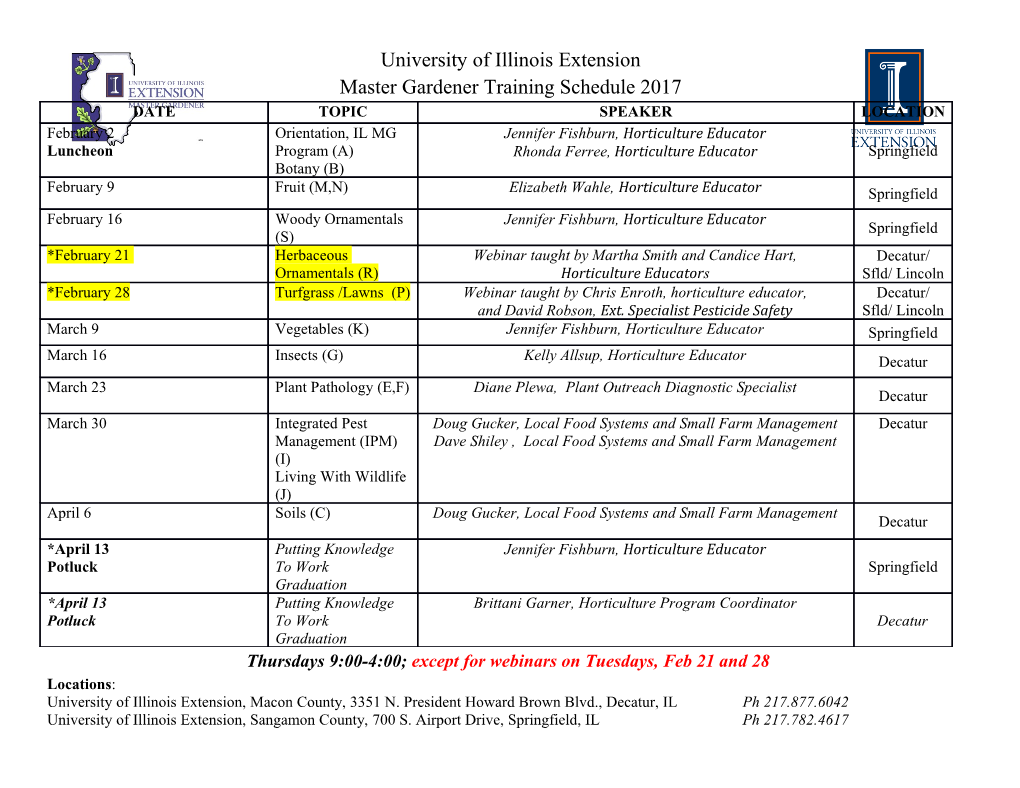
Approaches to Statistical Inference Fundamental Theory of Statistical Inference G. Alastair Young Department of Mathematics Imperial College London LTCC, 2013 http://www2.imperial.ac.uk/∼ayoung/ltcc.html G. Alastair Young Fundamental Theory of Statistical Inference Observational data modelled as observed values of random variables, to provide framework from which inductive conclusions may be drawn about mechanism giving rise to data. Approaches to Statistical Inference What is statistical inference? G. Alastair Young Fundamental Theory of Statistical Inference Approaches to Statistical Inference What is statistical inference? Observational data modelled as observed values of random variables, to provide framework from which inductive conclusions may be drawn about mechanism giving rise to data. G. Alastair Young Fundamental Theory of Statistical Inference I Regard y as observed value of random variable Y = (Y1;:::; Yn) having an (unknown) probability distribution specified by a probability density function, or probability mass function, f (y). I Restrict the unknown density to a suitable family F, of known analytical form, involving a finite number of real unknown 1 d T d parameters θ = (θ ; : : : ; θ ) . The region Ωθ ⊂ R of possible values of θ is called the parameter space. To indicate dependency of the density on θ write f (y; θ), the `model function'. I Assume that the objective of the analysis is to assessing some aspect of θ, for example the value of a single component θi . Approaches to Statistical Inference To analyse observations y = (y1;:::; yn). G. Alastair Young Fundamental Theory of Statistical Inference I Restrict the unknown density to a suitable family F, of known analytical form, involving a finite number of real unknown 1 d T d parameters θ = (θ ; : : : ; θ ) . The region Ωθ ⊂ R of possible values of θ is called the parameter space. To indicate dependency of the density on θ write f (y; θ), the `model function'. I Assume that the objective of the analysis is to assessing some aspect of θ, for example the value of a single component θi . Approaches to Statistical Inference To analyse observations y = (y1;:::; yn). I Regard y as observed value of random variable Y = (Y1;:::; Yn) having an (unknown) probability distribution specified by a probability density function, or probability mass function, f (y). G. Alastair Young Fundamental Theory of Statistical Inference I Assume that the objective of the analysis is to assessing some aspect of θ, for example the value of a single component θi . Approaches to Statistical Inference To analyse observations y = (y1;:::; yn). I Regard y as observed value of random variable Y = (Y1;:::; Yn) having an (unknown) probability distribution specified by a probability density function, or probability mass function, f (y). I Restrict the unknown density to a suitable family F, of known analytical form, involving a finite number of real unknown 1 d T d parameters θ = (θ ; : : : ; θ ) . The region Ωθ ⊂ R of possible values of θ is called the parameter space. To indicate dependency of the density on θ write f (y; θ), the `model function'. G. Alastair Young Fundamental Theory of Statistical Inference Approaches to Statistical Inference To analyse observations y = (y1;:::; yn). I Regard y as observed value of random variable Y = (Y1;:::; Yn) having an (unknown) probability distribution specified by a probability density function, or probability mass function, f (y). I Restrict the unknown density to a suitable family F, of known analytical form, involving a finite number of real unknown 1 d T d parameters θ = (θ ; : : : ; θ ) . The region Ωθ ⊂ R of possible values of θ is called the parameter space. To indicate dependency of the density on θ write f (y; θ), the `model function'. I Assume that the objective of the analysis is to assessing some aspect of θ, for example the value of a single component θi . G. Alastair Young Fundamental Theory of Statistical Inference I Point estimation I Confidence set estimation I Hypothesis testing Approaches to Statistical Inference Types of inference G. Alastair Young Fundamental Theory of Statistical Inference I Confidence set estimation I Hypothesis testing Approaches to Statistical Inference Types of inference I Point estimation G. Alastair Young Fundamental Theory of Statistical Inference I Hypothesis testing Approaches to Statistical Inference Types of inference I Point estimation I Confidence set estimation G. Alastair Young Fundamental Theory of Statistical Inference Approaches to Statistical Inference Types of inference I Point estimation I Confidence set estimation I Hypothesis testing G. Alastair Young Fundamental Theory of Statistical Inference I Bayesian I Fisherian I frequentist Approaches to Statistical Inference Three paradigms of inference G. Alastair Young Fundamental Theory of Statistical Inference I Fisherian I frequentist Approaches to Statistical Inference Three paradigms of inference I Bayesian G. Alastair Young Fundamental Theory of Statistical Inference I frequentist Approaches to Statistical Inference Three paradigms of inference I Bayesian I Fisherian G. Alastair Young Fundamental Theory of Statistical Inference Approaches to Statistical Inference Three paradigms of inference I Bayesian I Fisherian I frequentist G. Alastair Young Fundamental Theory of Statistical Inference Approaches to Statistical Inference Differences relate to interpretation of probability and objectives of statistical inference. G. Alastair Young Fundamental Theory of Statistical Inference Unknown parameter θ treated as random variable. Key: specification of prior distribution on θ, before data analysis. Objective or subjective specification. Inference is formalization of how prior changes, to posterior distribution, in light of data y, via Bayes' formula. Approaches to Statistical Inference Bayesian inference (Bayes, Laplace) G. Alastair Young Fundamental Theory of Statistical Inference Key: specification of prior distribution on θ, before data analysis. Objective or subjective specification. Inference is formalization of how prior changes, to posterior distribution, in light of data y, via Bayes' formula. Approaches to Statistical Inference Bayesian inference (Bayes, Laplace) Unknown parameter θ treated as random variable. G. Alastair Young Fundamental Theory of Statistical Inference Approaches to Statistical Inference Bayesian inference (Bayes, Laplace) Unknown parameter θ treated as random variable. Key: specification of prior distribution on θ, before data analysis. Objective or subjective specification. Inference is formalization of how prior changes, to posterior distribution, in light of data y, via Bayes' formula. G. Alastair Young Fundamental Theory of Statistical Inference Approaches to Statistical Inference Use of probability distributions as expressing opinion. G. Alastair Young Fundamental Theory of Statistical Inference Development of logic of inductive inference, releasing from a priori assumptions of Bayesian school. Repeated sampling principle: inference from y founded on comparison with datasets from hypothetical repetitions of experiment generating y, under exactly same conditions. Approaches to Statistical Inference Fisherian inference (Fisher) G. Alastair Young Fundamental Theory of Statistical Inference Repeated sampling principle: inference from y founded on comparison with datasets from hypothetical repetitions of experiment generating y, under exactly same conditions. Approaches to Statistical Inference Fisherian inference (Fisher) Development of logic of inductive inference, releasing from a priori assumptions of Bayesian school. G. Alastair Young Fundamental Theory of Statistical Inference Approaches to Statistical Inference Fisherian inference (Fisher) Development of logic of inductive inference, releasing from a priori assumptions of Bayesian school. Repeated sampling principle: inference from y founded on comparison with datasets from hypothetical repetitions of experiment generating y, under exactly same conditions. G. Alastair Young Fundamental Theory of Statistical Inference I Central role played by likelihood, maximum likelihood. I To be relevant to y, inference carried out conditional on everything known, uninformative about θ. Approaches to Statistical Inference Key elements of Fisherian statistics G. Alastair Young Fundamental Theory of Statistical Inference I To be relevant to y, inference carried out conditional on everything known, uninformative about θ. Approaches to Statistical Inference Key elements of Fisherian statistics I Central role played by likelihood, maximum likelihood. G. Alastair Young Fundamental Theory of Statistical Inference Approaches to Statistical Inference Key elements of Fisherian statistics I Central role played by likelihood, maximum likelihood. I To be relevant to y, inference carried out conditional on everything known, uninformative about θ. G. Alastair Young Fundamental Theory of Statistical Inference Origins in detailed analysis of concepts developed by Fisher, likelihood and sufficiency. Formal incorporation of optimality criteria. Approaches to Statistical Inference Frequentist inference (Pearson, Wald, Lehmann...) G. Alastair Young Fundamental Theory of Statistical Inference Approaches to Statistical Inference Frequentist inference (Pearson, Wald, Lehmann...) Origins in detailed analysis of concepts developed by Fisher, likelihood and sufficiency. Formal incorporation of optimality criteria. G. Alastair Young Fundamental Theory of Statistical Inference I Inference procedures as decisions problems,
Details
-
File Typepdf
-
Upload Time-
-
Content LanguagesEnglish
-
Upload UserAnonymous/Not logged-in
-
File Pages33 Page
-
File Size-