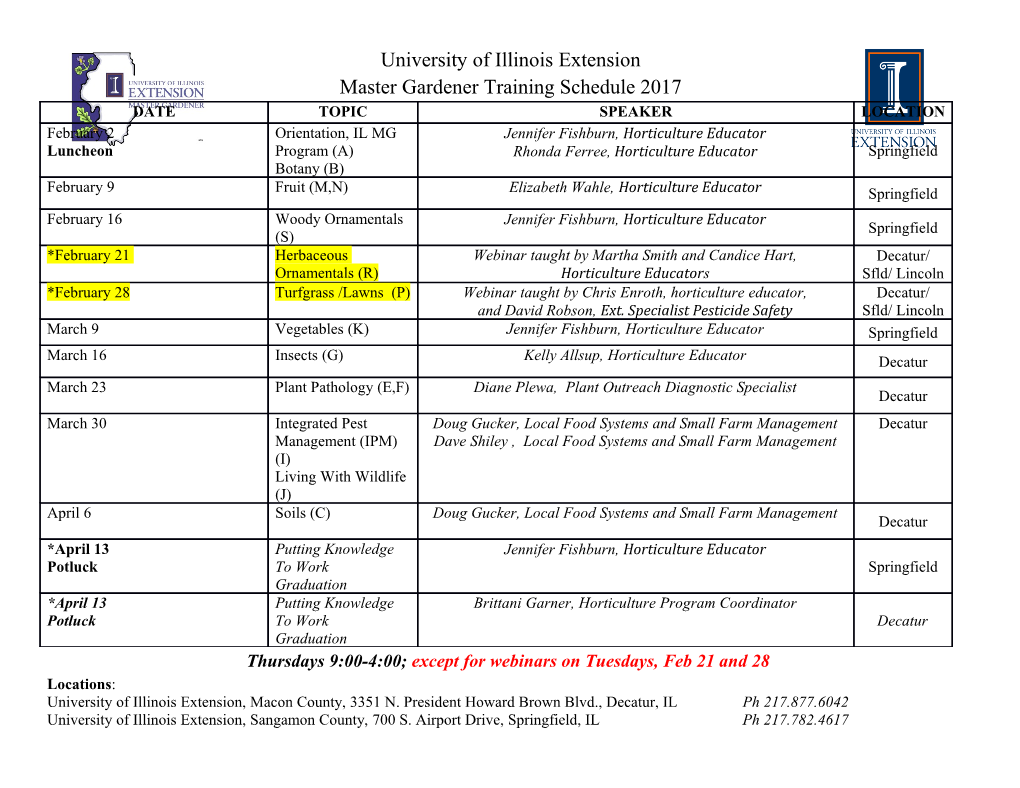
Atmospheric and Climate Sciences, 2021, 11, 284-301 https://www.scirp.org/journal/acs ISSN Online: 2160-0422 ISSN Print: 2160-0414 Classified Early Warning and Forecast of Severe Convective Weather Based on LightGBM Algorithm Xinwei Liu1, Haixia Duan2, Wubin Huang1*, Runxia Guo1, Bolong Duan1 1Lanzhou Central Meteorological Observatory, Lanzhou, China 2Lanzhou Institute of Drought Meteorology, China Meteorological Administration, Lanzhou, China How to cite this paper: Liu, X.W., Duan, Abstract H.X., Huang, W.B., Guo, R.X. and Duan, B.L. (2021) Classified Early Warning and Severe convective weather can lead to a variety of disasters, but they are still Forecast of Severe Convective Weather Based difficult to be pre-warned and forecasted in the meteorological operation. on LightGBM Algorithm. Atmospheric and This study generates a model based on the light gradient boosting machine Climate Sciences, 11, 284-301. https://doi.org/10.4236/acs.2021.112017 (LightGBM) algorithm using C-band radar echo products and ground obser- vations, to identify and classify three major types of severe convective weather Received: February 5, 2021 (i.e., hail, short-term heavy rain (STHR), convective gust (CG)). The model Accepted: April 11, 2021 evaluations show the LightGBM model performs well in the training set Published: April 14, 2021 (2011-2017) and the testing set (2018) with the overall false identification ra- Copyright © 2021 by author(s) and tio (FIR) of only 4.9% and 7.0%, respectively. Furthermore, the average Scientific Research Publishing Inc. probability of detection (POD), critical success index (CSI) and false alarm This work is licensed under the Creative ratio (FAR) for the three types of severe convective weather in two sample Commons Attribution International License (CC BY 4.0). sets are over 85%, 65% and lower than 30%, respectively. The LightGBM http://creativecommons.org/licenses/by/4.0/ model and the storm cell identification and tracking (SCIT) product are then Open Access used to forecast the severe convective weather 15 - 60 minutes in advance. The average POD, CSI and FAR for the forecasts of the three types of severe convective weather are 57.4%, 54.7% and 38.4%, respectively, which are sig- nificantly higher than those of the manual work. Among the three types of severe convective weather, the STHR has the highest POD and CSI and the lowest FAR, while the skill scores for the hail and CG are similar. Therefore, the LightGBM model constructed in this paper is able to identify, classify and forecast the three major types of severe convective weather automatically with relatively high accuracy, and has a broad application prospect in the future automatic meteorological operation. Keywords Severe Convective Weather, Machine Learning, LightGBM, Early Warning DOI: 10.4236/acs.2021.112017 Apr. 14, 2021 284 Atmospheric and Climate Sciences X. W. Liu et al. and Forecast 1. Introduction Severe convective weather usually refers to kinds of disastrous weather generated by deep moist convections, such as hail, gale, tornado and heavy precipitation [1]. Although there is no unified criterion for the definition of severe convective weather, the severe convective weather defined by the Central Meteorological Observatory of China Meteorological Administration refers to the event with any or several following weather conditions: hail with a diameter of 5 mm or above on the ground, tornado at any level on land, convective wind gust (CG) of more than 17 m∙s−1 and short-term heavy rainfall (STHR) of 20 mm∙h−1 or above [2]. Since the severe convective weather has strong destructiveness and often brings great harm to industry, agriculture and people’s safety, its nowcasting and early warning play a great important role in the meteorological disaster preven- tion and mitigation. Moreover, severe convective weather occurs abruptly and locally with short duration, so it is still difficult to be early warned and fore- casted in the meteorological operation. For example, the probability of detection of human forecasts of STHR, hail and CG are all less than 35%, while the false alarm ratio is even higher than 90% for the CG and hail in 2015-2017 [3]. Therefore, it is urgent to improve the forecast and early warning skills of severe convective weather in China and enhance the services of disaster prevention and mitigation. With the continuous densifying of the Doppler weather radar network, the radar-echo products have been playing a key role in the monitoring, analysis and short-term early warning of severe convective weather [4] [5]. At present, most of the new generation Doppler weather radars in China are S-band and C-band radars. The S-band weather radar stations mainly are located in eastern China, and their data are the majority of radar products in researches with advantages such as the weak echo attenuation (He, 2012). The C-band radar stations mainly are located in western China, but their data are not fully studied and applied in researches and operations. Although the C-band radar products have problem of echo attenuation, studies have shown that the research results based on the products of S-band and C-band radar have good consistency in detecting rain [6], hail [7] and so on. Due to the complex topography in northwestern China with plateaus, mountains and deserts, disasters such as mountain torrent and debris flow are easy to occur in extreme severe convective weather, such as the heavy mountain torrent and debris flow disaster in Zhouqu, Gansu Province on August 8, 2010 and the disaster of heavy hail, mountain torrent and debris flow in Min County, Gansu Province on May 10, 2012. Therefore, it is very important for the meteorological operation in northwestern China to explore the applica- tion of C-band radar in the early warning and forecast of severe convective DOI: 10.4236/acs.2021.112017 285 Atmospheric and Climate Sciences X. W. Liu et al. weather and enhance its warning and forecast skill. At present, the early warning and nowcasting (0 - 2 hours) of severe convec- tive weather are carried out mainly through the manual recognition of ra- dar-echo and satellite images. Traditional methods of the early warning and forecast of severe convective weather mainly include the extrapolation forecast, the experimental forecast, the statistical forecast [8] [9] and the probability fore- cast [10], which have certain limitations, such as hysteresis, and low accuracy. Artificial intelligence and machine learning can classify and identify severe con- vective weather automatically, rapidly and systematically without artificial devia- tion. Therefore, the application of advanced big data, machine learning and arti- ficial intelligence techniques in the short-term forecast of severe convective weather is one of the groundbreaking hotspots in meteorological research. For example, McGovern et al. [11] showed the applications of modern artificial in- telligence techniques in forecasting a wide variety of high-impact weather phe- nomena, including storm duration, severe wind, severe hail, precipitation classi- fication, renewable energy and aviation turbulence. Czerneckia et al. [12] applied machine learning to large hail predictions using the ERA5 data. Zhou et al. (2019) used a deep learning algorithm to forecast severe convective weather in- cluding hail, short-duration heavy rain, convective gust (CG) and thunderstorm, and found that the deep learning algorithm has a higher classification accuracy than support vector machine and random forest. Machine learning methods are also used to forecast the damage of straight-line wind [13], nowcast the 0 - 2 h storms [14] and lighting occurrence [15], diagnose aviation turbulence [16], map storm structures in advance [4] and even map the spatial distribution of soil or- ganic matter [17]. The light gradient boosting machine (LightGBM) algorithm is a research hot- spot in the data mining and classified prediction in recent years, and is widely used in the classification problems in all walks of life. For example, LightGBM is used in human activity recognition such as the safe driving [18], and the medical research such as the protein-protein interactions [19]. LightGBM is also widely used in the prediction of economics. Ma et al. [20] generated a prediction of peer-to-peer (P2P) network loan default based on the LightGBM and the ex- treme gradient boosting algorithms. Jiang et al. [21] predicted the directions of stock-price index using four machine-learning methods including the LightGBM. Sun et al. [22] used LightGBM to forecast the price trend of cryptocurrency market and found that the robustness of the LightGBM model is better than other methods. LightGBM has wide applications in atmospheric science as well, such as the prediction of air quality [23] [24] and wind power [25]. Moreover, Fan et al. [26] evaluated the LightGBM, random forest, the tree-based M5 Model Tree and four empirical models (Hargreaves-Samani, Tabari, Makkink and Trabert) to estimate daily reference evapotranspiration with local and external meteorological data, and pointed out that LightGBM generally performs better than other models. DOI: 10.4236/acs.2021.112017 286 Atmospheric and Climate Sciences X. W. Liu et al. Although some studies have applied machine learning in the classification and identification of a single type of severe convective weather [12] [13] [27], various types of severe convective weather often occur concomitantly. Therefore, this study develops a LightGBM model to identify and classify three major types of severe convective weather using C-band radar-echo data and ground observa- tions of severe convective weather. The LightGBM model is tested and evaluated in the training set and the testing set of independent samples, respectively, and then applied in the forecast of severe convective weather. The LightGBM algo- rithm and its model construction are introduced in Section 2. Section 3 intro- duces the datasets used in this study, including the radar products and ground observations of severe convective weather, and the methods of model evaluation.
Details
-
File Typepdf
-
Upload Time-
-
Content LanguagesEnglish
-
Upload UserAnonymous/Not logged-in
-
File Pages18 Page
-
File Size-