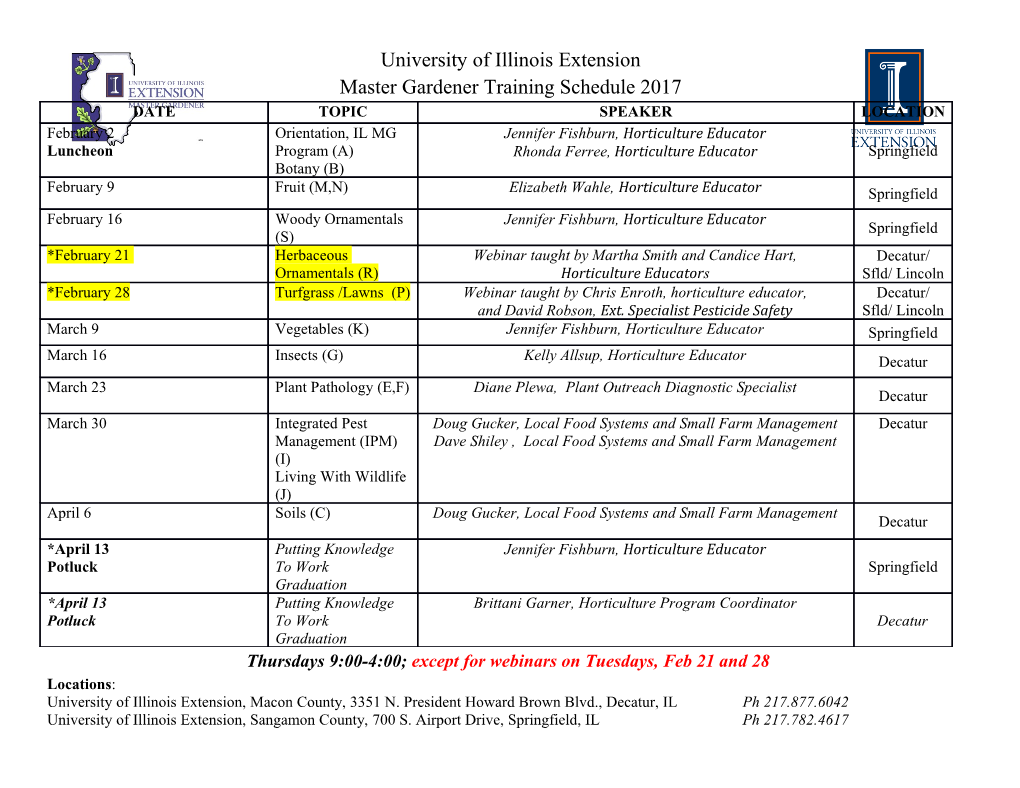
《Random Signal Processing》 Chapter4 Random Processes Chapter 4 Random Processes Contents 4 Random Processes........................................................................................................................................2 4.1 Definition of a Random Process........................................................................................................2 4.2 Characterization of a Random Process..............................................................................................4 4.2.1 Total Characterization of a Random Process..........................................................................4 4.2.2 First-Order Densities of a Random Process ...........................................................................5 4.2.3 Mean of a Random Process....................................................................................................5 4.2.4 Variance of a Random Process ...............................................................................................5 4.2.5 Second-Order Densities of a Random Process.......................................................................6 4.2.6 Autocorrelation and Autocovariance Functions of Random Process .....................................6 4.2.7 Power Spectral Density of a Random Process........................................................................7 4.2.8 Higher-order Moments ...........................................................................................................7 4.2.9 Higher-order Spectra ..............................................................................................................8 4.2.10 Nth-Order Densities..............................................................................................................8 4.3 Stationarity of Random Process ........................................................................................................8 4.3.1 Wide sense Stationary Random Process.................................................................................8 4.3.2 Properties for Wide Sense Stationary Random Processes ....................................................10 4.4 Examples of Random Processes......................................................................................................10 4.4.1 Straight Line Process............................................................................................................10 4.4.2 Semirandom Binary Transmission process...........................................................................13 4.4.3 Random Binary Transmission Process .................................................................................16 4.4.4 Semirandom Telegragh Waves Process ................................................................................18 4.4.5 Random Telegraph Process ..................................................................................................22 4.4.6 Random Sinusoidal Signals..................................................................................................23 4.4.7 Random Walk Process..........................................................................................................25 4.5 Definite In tegrate of Random Processes ........................................................................................29 4.6 Joint Characterizations of Random Process ....................................................................................31 4.6.1 First-Order Joint Densities ...................................................................................................32 4.6.2 Cross-correlation Function ...................................................................................................32 4.6.3 Cross-covariance Function ...................................................................................................32 4.6.4 Joint Stationarity...................................................................................................................32 4.6.5 Cross-spectral Density..........................................................................................................33 4.7 Gaussian Random Processes ...........................................................................................................33 4.7.1 First-Order Densities for Gaussian Random Processes........................................................33 4.7.2 Second-Order Densities for Gaussian Random Processes....................................................34 4.8 White Random Processes ................................................................................................................34 4.9 ARMA Random Processes ..............................................................................................................35 4.9.1 Moving Average Process, MA(q) .........................................................................................36 4.9.2 Autoregressive Processes, AR(p)..........................................................................................39 4.9.3 Autoregressive Moving Average Process, ARMA (p, q) ......................................................43 4.10 Periodic Random Processes...........................................................................................................47 4.11 Sampling of Continuous Random Processes .................................................................................47 4.12 Ergodic Random Processes ...........................................................................................................48 4.13 Summary .......................................................................................................................................49 Xidian University Liu Congfeng E-Mail:[email protected] Page 1 of 49 《Random Signal Processing》 Chapter4 Random Processes 4 Random Processes 4.1 Definition of a Random Process From chapter 2 and 3 we leaned that an experiment is specified by the three tuple S,, BF P ,where S is a countable or noncountable set representing the outcomes of the experiment, BF is a Borel field specifying the set of events for which consistent probabilities exist, and P is a probability measure that allows the calculation of the probability of all the events in the Borel field. A real random variable X e was defined as a real-valued function of S subject to (a) the event specified by e:() X e x is a member of the BF for all e , thus guaranteeing the existence of the cumulative distribution, and (b) P e: X ( e ) 0 or P e: X ( e ) 0 , or both. If, instead of assigning a real value to each e , a time function X t, e is defined for each e , we say a random process is specified. Roughly speaking, a random process is a family of time functions together with probability measure. For a finite sample space S we can visualize the random process as in Figure 4.1, that of a mapping from the sample space to a space of time waveforms. ei X(t,ei) S Waveform Space Figure 4.1 A Random process viewed as a functional mapping A random process can also be viewed as shown in Figure 4.2. For a particular outcome ei , with probability Pi , the time waveform shown X t, ei occurs. The n time signals represent an ensemble of time waveforms. If we evaluate these time waveforms at t0 , the values on the second column from the right are obtained . Coming down that column of values, we have a mapping from the outcomes on the far left, and this mapping describes a particular random variable X t0 . Similarly a random variable X t1 is defined for the mapping shown at time t1 as in the last column. A simple example of random process will now be given. Xidian University Liu Congfeng E-Mail:[email protected] Page 2 of 49 《Random Signal Processing》 Chapter4 Random Processes Random Random Probability Outcome Random Process Variable Variable P(e) e∈S X(t,e) X(t0,e) X(t1,e) 1 P e 1 1 0.5 -0.6 t -1 1 P e 2 2 -0.5 -0.8 t -1 … … … … … . P e 1 3 3 -0.4 0.0 t -1 t0 t1 Figure4.2 Illustration of a random process as an ensemble of time waveforms and a related sample space and probability measure. It should be noted that a random process X t, e is a function of two variables, t and e , where t is the time variable and e is the outcome variable. For a particular time t t0 , X t0 , e specifies a random variable. If e ei , or the outcome is fixed, then X t, ei is a particular time function called a sample function or realization of the random process. With both t and e fixed, say at t0 and ei , X t0 , e becomes a real number for a real random process or a complex value for a complex random process. As the notation for a random variable is usually a capital letter X ,Y or Z rather than the more formal X e ,Y e , Z e , a random process is usually denoted as X t rather than X t, e . Also, it is common to use a small x or x t , to indicate a particular value of the random variable X or a particular time function of a random process X t , respectively. Such an x t, or X t, ei , is called a sample function or a realization of the process. Also as X t, e is a particular random variable for each t , a common alternative definition of a random process is an indexed set of random variables where t the indexing set. If the index set γ can be put into a one to one correspondence with the set of integers, the random process is called a random sequence or a discrete time random process if t is time. While, if the indexing set takes on a continuum of values, we will call the resulting process a continuous random process or continuous time
Details
-
File Typepdf
-
Upload Time-
-
Content LanguagesEnglish
-
Upload UserAnonymous/Not logged-in
-
File Pages49 Page
-
File Size-