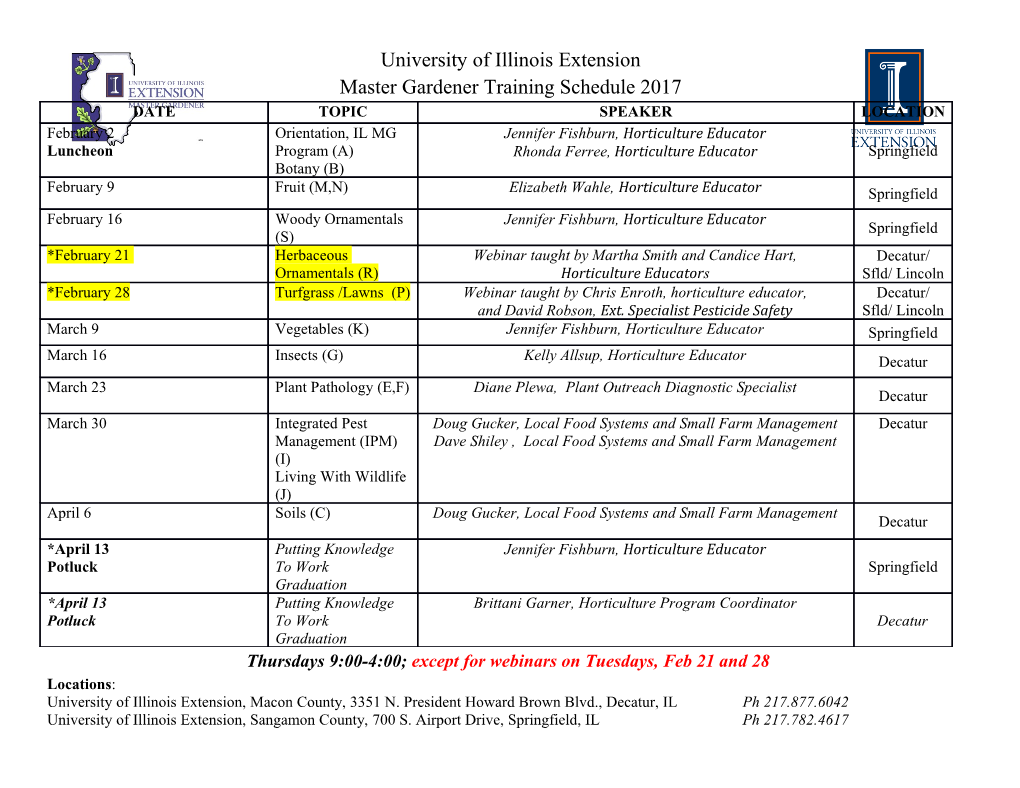
Multi-view Metric Learning in Vector-valued Kernel Spaces Riikka Huusari Hachem Kadri Cécile Capponi Aix Marseille Univ, Université de Toulon, CNRS, LIS, Marseille, France Abstract unlabeled examples [26]; the latter tries to efficiently combine multiple kernels defined on each view to exploit We consider the problem of metric learning for information coming from different representations [11]. multi-view data and present a novel method More recently, vector-valued reproducing kernel Hilbert for learning within-view as well as between- spaces (RKHSs) have been introduced to the field of view metrics in vector-valued kernel spaces, multi-view learning for going further than MKL by in- as a way to capture multi-modal structure corporating in the learning model both within-view and of the data. We formulate two convex opti- between-view dependencies [20, 14]. It turns out that mization problems to jointly learn the metric these kernels and their associated vector-valued repro- and the classifier or regressor in kernel feature ducing Hilbert spaces provide a unifying framework for spaces. An iterative three-step multi-view a number of previous multi-view kernel methods, such metric learning algorithm is derived from the as co-regularized multi-view learning and manifold reg- optimization problems. In order to scale the ularization, and naturally allow to encode within-view computation to large training sets, a block- as well as between-view similarities [21]. wise Nyström approximation of the multi-view Kernels of vector-valued RKHSs are positive semidefi- kernel matrix is introduced. We justify our ap- nite matrix-valued functions. They have been applied proach theoretically and experimentally, and with success in various machine learning problems, such show its performance on real-world datasets as multi-task learning [10], functional regression [15] against relevant state-of-the-art methods. and structured output prediction [5]. The main advan- tage of matrix-valued kernels is that they offer a higher degree of flexibility in encoding similarities between 1 Introduction data points. However finding the optimal matrix-valued kernel of choice for a given application is difficult, as is In this paper we tackle the problem of supervised multi- the question of how to build them. In order to overcome view learning, where each labeled example is observed the need for choosing a kernel before the learning pro- under several views. These views might be not only cess, we propose a supervised metric learning approach correlated, but also complementary, redundant or con- that learns a matrix-valued multi-view kernel jointly tradictory. Thus, learning over all the views is expected with the decision function. We refer the reader to [3] for arXiv:1803.07821v1 [cs.LG] 21 Mar 2018 to produce a final classifier (or regressor) that is better a review of metric learning. It is worth mentioning that than each individual one. Multi-view learning is well- algorithms for learning matrix-valued kernels have been known in the semi-supervised setting, where the agree- proposed in the literature, see for example [9, 8, 17]. ment among views is usually optimized [4, 28]. Yet, the However, these methods mainly consider separable ker- supervised setting has proven to be interesting as well, nels which are not suited for multi-view setting, as will independently from any agreement condition on views. be illustrated later in this paper. Co-regularization and multiple kernel learning (MKL) The main contributions of this paper are: 1) we intro- are two well known kernel-based frameworks for learn- duce and learn a new class of matrix-valued kernels de- ing in the presence of multiple views of data [31]. The signed to handle multi-view data 2) we give an iterative former attempts to optimize measures of agreement algorithm that learns simultaneously a vector-valued and smoothness between the views over labeled and multi-view function and a block-structured metric be- tween views, 3) we provide generalization analysis of Proceedings of the 21st International Conference on Artifi- our algorithm with a Rademacher bound; and 4) we cial Intelligence and Statistics (AISTATS) 2018, Lanzarote, show how matrix-valued kernels can be efficiently com- Spain. PMLR: Volume 84. Copyright 2018 by the author(s). puted via a block-wise Nyström approximation in order Multi-view Metric Learning in Vector-valued Kernel Spaces to reduce significantly their high computational cost. kernels are defined by 2 Preliminaries K(x; z) = k(x; z)T; where T is a matrix in v×v. This class of kernels is We start here by briefly reviewing the basics of vector- R very attractive in terms of computational time, as it valued RKHSs and their associated matrix-valued ker- is easily decomposable. However the matrix T acts nels. We then describe how they can be used for learn- only on the outputs independently of the input data, ing from multi-view data. which makes it difficult for these kernels to encode nec- essary similarities in multi-view setting. Transformable 2.1 Vector-valued RKHSs kernels are defined by Vector-valued RKHSs were introduced to the machine [K(x; z)] = k(S x;S z): learning community by Micchelli and Pontil [19] as a lm m l way to extend kernel machines from scalar to vector Here m and l are indices of the output matrix (views outputs. In this setting, given a random training sample in multi-view setting) and operators fS gv , are used n t t=1 fxi; yigi=1 on X × Y, optimization problem to transform the data. In contrast to separable kernels, n here the St operate on input data; however choosing X 2 arg min V (f; xi; yi) + λkfkH; (1) them is a difficult task. For further reading on matrix- f2H i=1 valued reproducing kernels, see, e.g., [1, 6, 7, 15]. where f is a vector-valued function and V is a loss function, can be solved in a vector-valued RKHS H by 2.2 Vector-valued multi-view learning the means of a vector-valued extension of the represen- This section reviews the setup for supervised multi-view ter theorem. To see this more clearly, we recall some learning in vector-valued RKHSs [14, 21]. The main fundamentals of vector-valued RKHSs. idea is to consider a kernel that measures not only the Definition 1. (vector-valued RKHS) similarities between examples of the same view but also A Hilbert space H of functions from X to Rv is called those coming from different views. Reproducing ker- a reproducing kernel Hilbert space if there is a positive nels of vector-valued Hilbert spaces allow encoding in a definite Rv×v-valued kernel K on X × X such that: natural way these similarities and taking into account i. the function z 7! K(x; z)y belongs to H; 8z; x 2 both within-view and between-view dependencies. In- deed, a kernel function K in this setting outputs a X ; y 2 Rv, matrix in Rv×v, with v the number of views, so, that v ii. 8f 2 H; x 2 X ; y 2 R ; hf; K(x; ·)yiH = K(xi; xj)lm, l; m = 1; : : : ; v, is the similarity measure v hf(x); yiR (reproducing property). between examples xi and xj from the views l and m. Definition 2. (matrix-valued kernel) More formally, consider a set of n labeled data v×v f(x ; y ) 2 X × Y; i = 1; : : : ; ng, where X ⊂ d and An R -valued kernel K on X × X is a function i i R v×v Y = {−1; 1g for classification or Y ⊂ for regression. K(·; ·): X × X ! R ; it is positive semidefinite if: R Also assume that each input instance x = (x1;:::; xv) > > i i i i. K(x; z) = K(z; x) , where denotes the transpose l dl Pv is seen in v views, where xi 2 R and l=1 dl = d. of a matrix, The supervised multi-view learning problem can be thought of as trying to find the vector-valued function ii. and, for every r 2 N and all f(xi; yi)i=1;:::;rg 2 v ^ ^1 ^v ^l P v f(·) = (f (·);::: f (·)), with f (x) 2 Y, solution of X × R , i;jhyi;K(xi; xj)yjiR ≥ 0. n Important results for matrix-valued kernels include the X 2 arg min V (yi; W(f(xi))) + λkfk : (2) positive semidefiniteness of the kernel K and that we ob- f2H;W i=1 tain a solution for regularized optimization problem (1) via a representer theorem. It states that solution f^ 2 H Here f is a vector-valued function that groups v learn- for a learning problem can be written as ing functions, each corresponding to one view, and W : v ! is combination operator for combining n R R ^ X v the results of the learning functions. f(x) = K(x; xi)ci; with ci 2 R : i=1 While the vector-valued extension of the representer theorem provides an algorithmc way for computing the Some well-known classes of matrix-valued kernels in- solution of the multi-view learning problem (2), the clude separable and transformable kernels. Separable question of choosing the multi-view kernel K remains Riikka Huusari, Hachem Kadri, Cécile Capponi n crucial to take full advantage of the vector-valued learn- where we have written kl(xi) = (kl(xt; xi))t=1. We ing framework. In [14], a matrix-valued kernel based on note that this class is not in general separable or trans- cross-covariance operators on RKHS that allow mod- formable. However in the special case when it is possi- eling variables of multiple types and modalities was ble to write Aml = AmAl the kernel is transformable.
Details
-
File Typepdf
-
Upload Time-
-
Content LanguagesEnglish
-
Upload UserAnonymous/Not logged-in
-
File Pages12 Page
-
File Size-