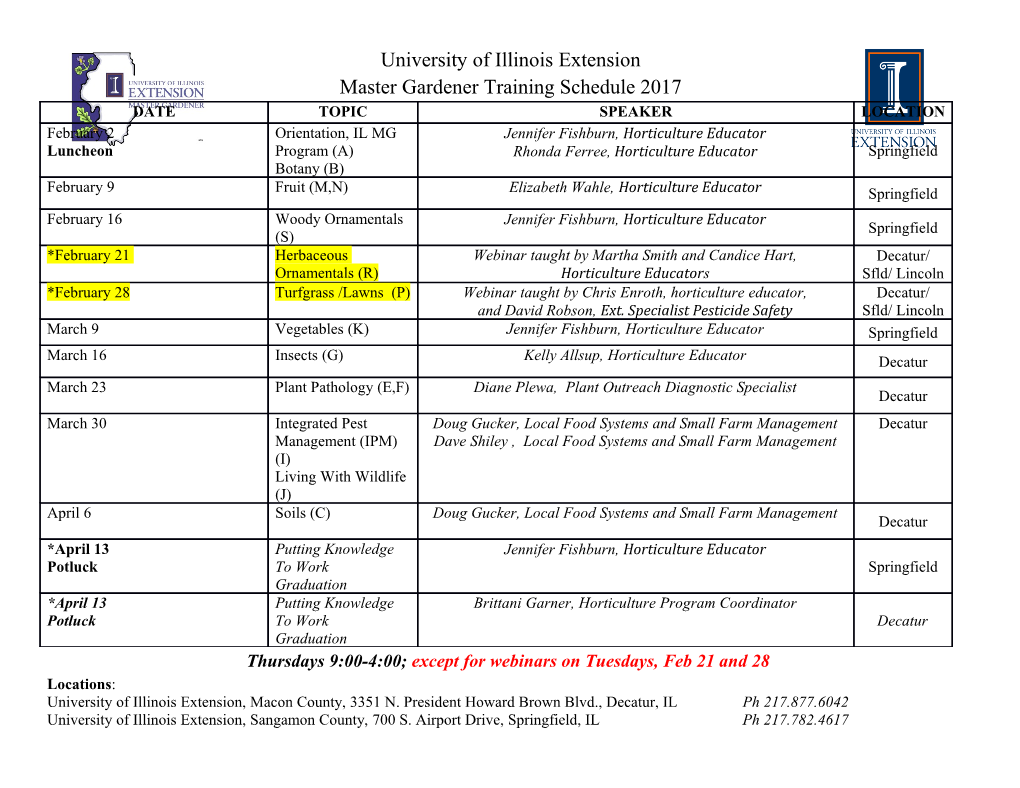
Goal of Analysis of Variance The Formal ANOVA Model Explanation by Example Multiple Comparisons Assumptions Analysis of Variance and Contrasts Ken Kelley's Class Notes 1 / 104 Goal of Analysis of Variance The Formal ANOVA Model Explanation by Example Multiple Comparisons Assumptions Lesson Breakdown by Topic Example: Weight Loss Drink 1 Goal of Analysis of Variance ANOVA Using SPSS A Conceptual Example Appropriate for ANOVA 4 Multiple Comparisons Example F -Test for Independent Why Multiplicity Matters Variances Error Rates Conceptual Underpinnings of Linear Combinations of Means ANOVA Controlling the Type I Error Mean Squares 5 Assumptions 2 The Formal ANOVA Model Assumptions of the ANOVA A Worked Example What You Learned 3 Explanation by Example Notations 2 / 104 Goal of Analysis of Variance The Formal ANOVA Model Explanation by Example Multiple Comparisons Assumptions What You Will Learn from this Lesson You will learn: How to compare more than two independent means to assess if there are any differences via an analysis of variance (ANOVA). How the total sums of squares for the data can be decomposed into a part that is due to the mean differences between groups and to a part that is due to within group differences. Why doing multiple t-tests is not the same thing as ANOVA. Why doing multiple t-tests leads to a multiplicity issue, in that as the number of tests increases, so to does the probability of one or more error. How to correct for the multiplicity issue in order for a set of contrasts/comparisons has a Type I error rate for the collection of tests at the desirable (e.g., .05) level. How to use SPSS and R to implement an ANOVA and follow-up tests. 3 / 104 Goal of Analysis of Variance The Formal ANOVA Model Explanation by Example Multiple Comparisons Assumptions Motivation When looking at different allergy medicines, there are numerous options. So how can it be determined which brand will work best when they all claim to do so? Data could be collected to determine the outcomes from each product among numerous individuals randomly assigned to different brands. An ANOVA could be run to infer if there is a performance difference between these different brands. If there are no significant results, evidence would not exist to suggest there are differences in performance among the brands. If there are significant results, we would infer that the brands do not perform the same, but further tests would have to be conducted so as to infer where the differences are . 4 / 104 Goal of Analysis of Variance A Conceptual Example Appropriate for ANOVA The Formal ANOVA Model Example F -Test for Independent Variances Explanation by Example Conceptual Underpinnings of ANOVA Multiple Comparisons Mean Squares Assumptions Goal of Analysis of Variance The goal of ANOVA is to detect if mean differences exist among m groups. Recall the independent groups t-test is designed to detect differences between two independent groups. 2 The t-test is a special case of ANOVA when m = 2 (tdf equals the F(1;df ) from ANOVA for two groups). 5 / 104 Goal of Analysis of Variance A Conceptual Example Appropriate for ANOVA The Formal ANOVA Model Example F -Test for Independent Variances Explanation by Example Conceptual Underpinnings of ANOVA Multiple Comparisons Mean Squares Assumptions Obtaining a statistically significant result for ANOVA conveys that not all groups have the same population mean. However, a statistically significant ANOVA with more than two groups does not convey where those differences exist. Follow-up tests (contrasts/comparisons) can be conducted to help discern specifically where group means differ. 6 / 104 Goal of Analysis of Variance A Conceptual Example Appropriate for ANOVA The Formal ANOVA Model Example F -Test for Independent Variances Explanation by Example Conceptual Underpinnings of ANOVA Multiple Comparisons Mean Squares Assumptions Consumer Preference Consider the overall perception of how consumers regard different companies. An experiment was done in which 30 individuals were randomly assigned into one of three groups. All participants saw (almost) the same commercial advertising a new Android smart phone. The difference between the groups was that the commercial attributed the phone to either (a) Nokia, (b) Samsung, or (c) Motorola. Of interest is in whether consumers tend to rate the brands differently, even for the \same" cell phone. 7 / 104 Goal of Analysis of Variance A Conceptual Example Appropriate for ANOVA The Formal ANOVA Model Example F -Test for Independent Variances Explanation by Example Conceptual Underpinnings of ANOVA Multiple Comparisons Mean Squares Assumptions What are other examples in which ANOVA would be useful? 8 / 104 Notice that F cannot be negative and is unbound on the high side. F -is a positively skewed distribution. The F -statistic is used to evaluate the above null hypothesis, and is defined as the ratio of two independent variances: 2 s1 F(df1;df2) = 2 ; s2 2 2 where df1 and df2 are the degrees of freedom for s1 and s2 , respectively. Goal of Analysis of Variance A Conceptual Example Appropriate for ANOVA The Formal ANOVA Model Example F -Test for Independent Variances Explanation by Example Conceptual Underpinnings of ANOVA Multiple Comparisons Mean Squares Assumptions Consider the null hypotheses of equal variances: 2 2 H0: σ1 = σ2: 9 / 104 Notice that F cannot be negative and is unbound on the high side. F -is a positively skewed distribution. Goal of Analysis of Variance A Conceptual Example Appropriate for ANOVA The Formal ANOVA Model Example F -Test for Independent Variances Explanation by Example Conceptual Underpinnings of ANOVA Multiple Comparisons Mean Squares Assumptions Consider the null hypotheses of equal variances: 2 2 H0: σ1 = σ2: The F -statistic is used to evaluate the above null hypothesis, and is defined as the ratio of two independent variances: 2 s1 F(df1;df2) = 2 ; s2 2 2 where df1 and df2 are the degrees of freedom for s1 and s2 , respectively. 10 / 104 Goal of Analysis of Variance A Conceptual Example Appropriate for ANOVA The Formal ANOVA Model Example F -Test for Independent Variances Explanation by Example Conceptual Underpinnings of ANOVA Multiple Comparisons Mean Squares Assumptions Consider the null hypotheses of equal variances: 2 2 H0: σ1 = σ2: The F -statistic is used to evaluate the above null hypothesis, and is defined as the ratio of two independent variances: 2 s1 F(df1;df2) = 2 ; s2 2 2 where df1 and df2 are the degrees of freedom for s1 and s2 , respectively. Notice that F cannot be negative and is unbound on the high side. F -is a positively skewed distribution. 11 / 104 Goal of Analysis of Variance A Conceptual Example Appropriate for ANOVA The Formal ANOVA Model Example F -Test for Independent Variances Explanation by Example Conceptual Underpinnings of ANOVA Multiple Comparisons Mean Squares Assumptions Examples We have previously asked questions about the mean difference, but the F -distribution allows us to ask questions about variability. Is the variability of user satisfaction of Gmail users different than the variability of user satisfaction of Outlook.com? Does Mars and their M&M's production have \better control" (i.e., smaller variance) than Wrigley's Skittles? For a given item, are Wal-Mart prices across the country more stable than Kroger's (for like items)? Does a particular machine (or location/worker/shift) produce more variable products than a counterpart? 12 / 104 Goal of Analysis of Variance A Conceptual Example Appropriate for ANOVA The Formal ANOVA Model Example F -Test for Independent Variances Explanation by Example Conceptual Underpinnings of ANOVA Multiple Comparisons Mean Squares Assumptions The standard deviation of Gmail user satisfaction was 6.35 based on a sample size of 55. The standard deviation of Outlook.com user satisfaction was 8.90 based on a sample size of 42. For an F -test of this sort addressing any differences in the variance (e.g., is there more variability in user satisfaction in one group), there are two critical values, one at the α=2 value and one at the 1 − α=2 value. The critical values are and for the .025 and .975 quantiles (i.e., when α = :05). The F -statistic for the test of the null hypothesis is 6:352 40:3225 F = = = :509: 8:902 79:21 The conclusion is: . 13 / 104 Goal of Analysis of Variance A Conceptual Example Appropriate for ANOVA The Formal ANOVA Model Example F -Test for Independent Variances Explanation by Example Conceptual Underpinnings of ANOVA Multiple Comparisons Mean Squares Assumptions Thus far, we have talked only about the idea of comparing two variances. But, what does this have to do with comparing means, which is the question we are interested in addressing? 14 / 104 We thus consider the variability of the group means to assess if the population group means differ. one variance calculates the variance of the group means; another variance is the (weighted) mean of within group 2 variances (recall sp from the two group t-test). Goal of Analysis of Variance A Conceptual Example Appropriate for ANOVA The Formal ANOVA Model Example F -Test for Independent Variances Explanation by Example Conceptual Underpinnings of ANOVA Multiple Comparisons Mean Squares Assumptions Analysis of variance (ANOVA) considers two variances: 15 / 104 We thus consider the variability of the group means to assess if the population group means differ. Goal of Analysis of Variance A Conceptual Example Appropriate for ANOVA The Formal ANOVA Model Example F -Test for Independent Variances Explanation by Example Conceptual Underpinnings of ANOVA Multiple Comparisons Mean Squares Assumptions Analysis of variance (ANOVA) considers two variances: one variance calculates the variance of the group means; another variance is the (weighted) mean of within group 2 variances (recall sp from the two group t-test).
Details
-
File Typepdf
-
Upload Time-
-
Content LanguagesEnglish
-
Upload UserAnonymous/Not logged-in
-
File Pages104 Page
-
File Size-