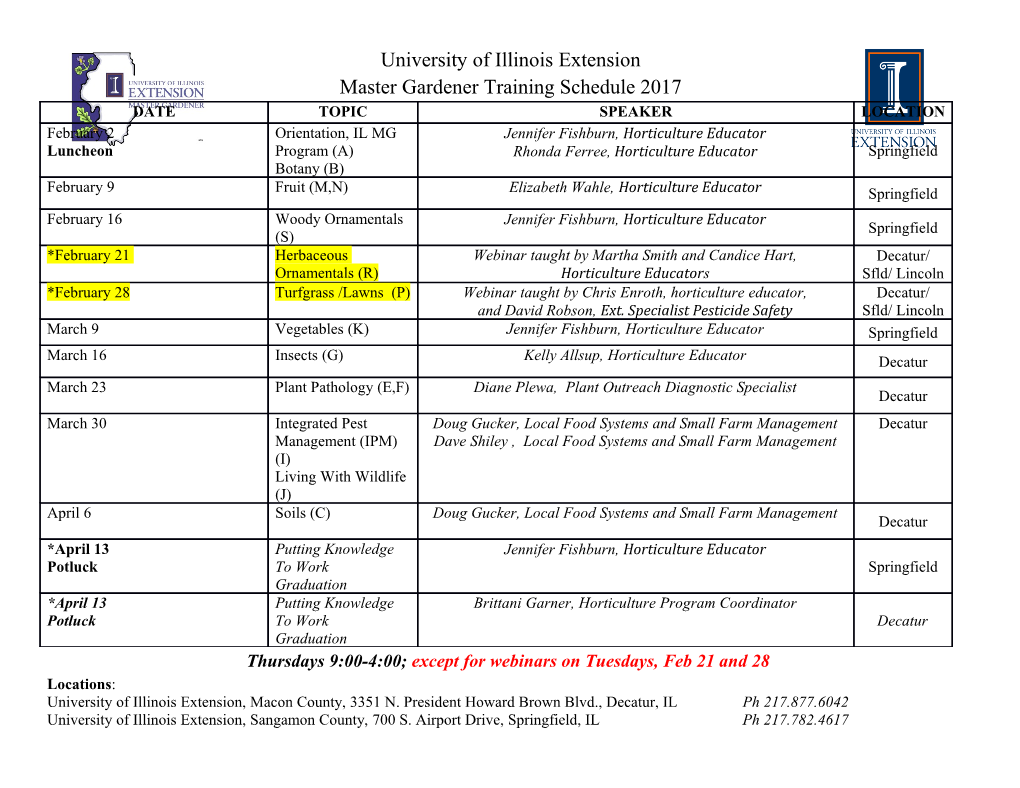
Generative Models for Dimensionality Reduction: Probabilistic PCA and Factor Analysis Piyush Rai Machine Learning (CS771A) Oct 5, 2016 Machine Learning (CS771A) Generative Models for Dimensionality Reduction: Probabilistic PCA and Factor Analysis 1 K Generate latent variables z n 2 R (K D) as z n ∼ N (0; IK ) Generate data x n conditioned on z n as 2 x n ∼ N (Wz n; σ ID ) where W is the D × K \factor loading matrix" or \dictionary" z n is K-dim latent features or latent factors or \coding" of x n w.r.t. W Note: Can also write x n as a linear transformation of z n, plus Gaussian noise 2 x n = Wz n + n (where n ∼ N (0; σ ID )) This is \Probabilistic PCA" (PPCA) with Gaussian observation model 2 N Want to learn model parameters W; σ and latent factors fz ngn=1 When n ∼ N (0; Ψ), Ψ is diagonal, it is called \Factor Analysis" (FA) Generative Model for Dimensionality Reduction D Assume the following generative story for each x n 2 R Machine Learning (CS771A) Generative Models for Dimensionality Reduction: Probabilistic PCA and Factor Analysis 2 Generate data x n conditioned on z n as 2 x n ∼ N (Wz n; σ ID ) where W is the D × K \factor loading matrix" or \dictionary" z n is K-dim latent features or latent factors or \coding" of x n w.r.t. W Note: Can also write x n as a linear transformation of z n, plus Gaussian noise 2 x n = Wz n + n (where n ∼ N (0; σ ID )) This is \Probabilistic PCA" (PPCA) with Gaussian observation model 2 N Want to learn model parameters W; σ and latent factors fz ngn=1 When n ∼ N (0; Ψ), Ψ is diagonal, it is called \Factor Analysis" (FA) Generative Model for Dimensionality Reduction D Assume the following generative story for each x n 2 R K Generate latent variables z n 2 R (K D) as z n ∼ N (0; IK ) Machine Learning (CS771A) Generative Models for Dimensionality Reduction: Probabilistic PCA and Factor Analysis 2 where W is the D × K \factor loading matrix" or \dictionary" z n is K-dim latent features or latent factors or \coding" of x n w.r.t. W Note: Can also write x n as a linear transformation of z n, plus Gaussian noise 2 x n = Wz n + n (where n ∼ N (0; σ ID )) This is \Probabilistic PCA" (PPCA) with Gaussian observation model 2 N Want to learn model parameters W; σ and latent factors fz ngn=1 When n ∼ N (0; Ψ), Ψ is diagonal, it is called \Factor Analysis" (FA) Generative Model for Dimensionality Reduction D Assume the following generative story for each x n 2 R K Generate latent variables z n 2 R (K D) as z n ∼ N (0; IK ) Generate data x n conditioned on z n as 2 x n ∼ N (Wz n; σ ID ) Machine Learning (CS771A) Generative Models for Dimensionality Reduction: Probabilistic PCA and Factor Analysis 2 z n is K-dim latent features or latent factors or \coding" of x n w.r.t. W Note: Can also write x n as a linear transformation of z n, plus Gaussian noise 2 x n = Wz n + n (where n ∼ N (0; σ ID )) This is \Probabilistic PCA" (PPCA) with Gaussian observation model 2 N Want to learn model parameters W; σ and latent factors fz ngn=1 When n ∼ N (0; Ψ), Ψ is diagonal, it is called \Factor Analysis" (FA) Generative Model for Dimensionality Reduction D Assume the following generative story for each x n 2 R K Generate latent variables z n 2 R (K D) as z n ∼ N (0; IK ) Generate data x n conditioned on z n as 2 x n ∼ N (Wz n; σ ID ) where W is the D × K \factor loading matrix" or \dictionary" Machine Learning (CS771A) Generative Models for Dimensionality Reduction: Probabilistic PCA and Factor Analysis 2 Note: Can also write x n as a linear transformation of z n, plus Gaussian noise 2 x n = Wz n + n (where n ∼ N (0; σ ID )) This is \Probabilistic PCA" (PPCA) with Gaussian observation model 2 N Want to learn model parameters W; σ and latent factors fz ngn=1 When n ∼ N (0; Ψ), Ψ is diagonal, it is called \Factor Analysis" (FA) Generative Model for Dimensionality Reduction D Assume the following generative story for each x n 2 R K Generate latent variables z n 2 R (K D) as z n ∼ N (0; IK ) Generate data x n conditioned on z n as 2 x n ∼ N (Wz n; σ ID ) where W is the D × K \factor loading matrix" or \dictionary" z n is K-dim latent features or latent factors or \coding" of x n w.r.t. W Machine Learning (CS771A) Generative Models for Dimensionality Reduction: Probabilistic PCA and Factor Analysis 2 This is \Probabilistic PCA" (PPCA) with Gaussian observation model 2 N Want to learn model parameters W; σ and latent factors fz ngn=1 When n ∼ N (0; Ψ), Ψ is diagonal, it is called \Factor Analysis" (FA) Generative Model for Dimensionality Reduction D Assume the following generative story for each x n 2 R K Generate latent variables z n 2 R (K D) as z n ∼ N (0; IK ) Generate data x n conditioned on z n as 2 x n ∼ N (Wz n; σ ID ) where W is the D × K \factor loading matrix" or \dictionary" z n is K-dim latent features or latent factors or \coding" of x n w.r.t. W Note: Can also write x n as a linear transformation of z n, plus Gaussian noise 2 x n = Wz n + n (where n ∼ N (0; σ ID )) Machine Learning (CS771A) Generative Models for Dimensionality Reduction: Probabilistic PCA and Factor Analysis 2 2 N Want to learn model parameters W; σ and latent factors fz ngn=1 When n ∼ N (0; Ψ), Ψ is diagonal, it is called \Factor Analysis" (FA) Generative Model for Dimensionality Reduction D Assume the following generative story for each x n 2 R K Generate latent variables z n 2 R (K D) as z n ∼ N (0; IK ) Generate data x n conditioned on z n as 2 x n ∼ N (Wz n; σ ID ) where W is the D × K \factor loading matrix" or \dictionary" z n is K-dim latent features or latent factors or \coding" of x n w.r.t. W Note: Can also write x n as a linear transformation of z n, plus Gaussian noise 2 x n = Wz n + n (where n ∼ N (0; σ ID )) This is \Probabilistic PCA" (PPCA) with Gaussian observation model Machine Learning (CS771A) Generative Models for Dimensionality Reduction: Probabilistic PCA and Factor Analysis 2 When n ∼ N (0; Ψ), Ψ is diagonal, it is called \Factor Analysis" (FA) Generative Model for Dimensionality Reduction D Assume the following generative story for each x n 2 R K Generate latent variables z n 2 R (K D) as z n ∼ N (0; IK ) Generate data x n conditioned on z n as 2 x n ∼ N (Wz n; σ ID ) where W is the D × K \factor loading matrix" or \dictionary" z n is K-dim latent features or latent factors or \coding" of x n w.r.t. W Note: Can also write x n as a linear transformation of z n, plus Gaussian noise 2 x n = Wz n + n (where n ∼ N (0; σ ID )) This is \Probabilistic PCA" (PPCA) with Gaussian observation model 2 N Want to learn model parameters W; σ and latent factors fz ngn=1 Machine Learning (CS771A) Generative Models for Dimensionality Reduction: Probabilistic PCA and Factor Analysis 2 Generative Model for Dimensionality Reduction D Assume the following generative story for each x n 2 R K Generate latent variables z n 2 R (K D) as z n ∼ N (0; IK ) Generate data x n conditioned on z n as 2 x n ∼ N (Wz n; σ ID ) where W is the D × K \factor loading matrix" or \dictionary" z n is K-dim latent features or latent factors or \coding" of x n w.r.t. W Note: Can also write x n as a linear transformation of z n, plus Gaussian noise 2 x n = Wz n + n (where n ∼ N (0; σ ID )) This is \Probabilistic PCA" (PPCA) with Gaussian observation model 2 N Want to learn model parameters W; σ and latent factors fz ngn=1 When n ∼ N (0; Ψ), Ψ is diagonal, it is called \Factor Analysis" (FA) Machine Learning (CS771A) Generative Models for Dimensionality Reduction: Probabilistic PCA and Factor Analysis 2 Wdk denotes the weight of relationship between feature d and latent factor k This view also helps in thinking about \deep" generative models that have many layers of latent variables or \hidden units" Generative Model for Dimensionality Reduction D K Zooming in at the relationship between each x n 2 R and each z n 2 R Machine Learning (CS771A) Generative Models for Dimensionality Reduction: Probabilistic PCA and Factor Analysis 3 This view also helps in thinking about \deep" generative models that have many layers of latent variables or \hidden units" Generative Model for Dimensionality Reduction D K Zooming in at the relationship between each x n 2 R and each z n 2 R Wdk denotes the weight of relationship between feature d and latent factor k Machine Learning (CS771A) Generative Models for Dimensionality Reduction: Probabilistic PCA and Factor Analysis 3 Generative Model for Dimensionality Reduction D K Zooming in at the relationship between each x n 2 R and each z n 2 R Wdk denotes the weight of relationship between feature d and latent factor k This view also helps in thinking about \deep" generative models that have many layers of latent variables or \hidden units" Machine Learning (CS771A) Generative Models for Dimensionality Reduction: Probabilistic PCA and Factor Analysis 3 (x = Wz + b + , where ∼ N (0; Σx )) A few nice properties of such systems (follow from properties of Gaussians): The marginal distribution of x, i.e., p(x), is Gaussian Z Z > p(x) = p(x; z)dz = p(xjz)p(z)dz = N (Wµz + b; Σx + WΣz W ) The posterior distribution of z, i.e., p(zjx) / p(z)p(xjz) is Gaussian p(zjx) = N (µ; Σ) −1 −1 > −1 Σ = Σz + W Σx W > −1 −1 µ = Σ[W Σx (x − b) + Σz µz ] (Chapter 4 of Murphy and Chapter 2 of Bishop have various useful results on properties of multivar.
Details
-
File Typepdf
-
Upload Time-
-
Content LanguagesEnglish
-
Upload UserAnonymous/Not logged-in
-
File Pages100 Page
-
File Size-