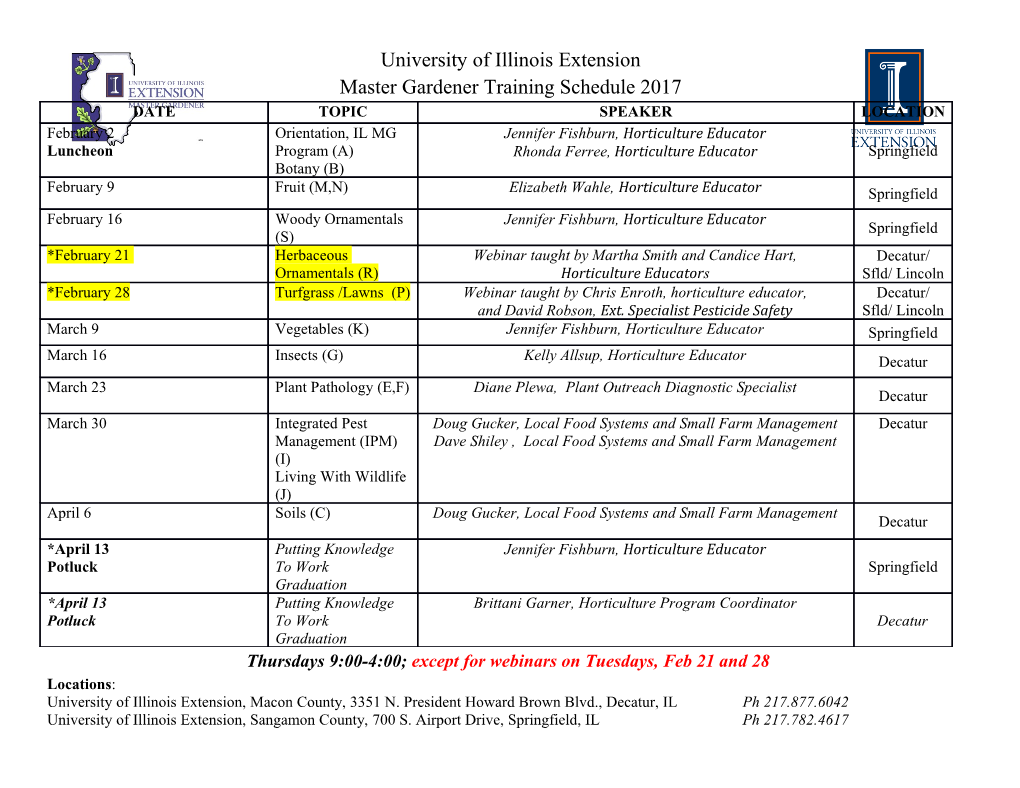
Cooperative Ramp Metering for Urban Motorways Based on Machine Learning Gregurić, Martin Doctoral thesis / Disertacija 2018 Degree Grantor / Ustanova koja je dodijelila akademski / stručni stupanj: University of Zagreb, Faculty of Transport and Traffic Sciences / Sveučilište u Zagrebu, Fakultet prometnih znanosti Permanent link / Trajna poveznica: https://urn.nsk.hr/urn:nbn:hr:119:512923 Rights / Prava: In copyright Download date / Datum preuzimanja: 2021-10-04 Repository / Repozitorij: Faculty of Transport and Traffic Sciences - Institutional Repository Faculty of Transport and Traffic Sciences Martin Gregurić COOPERATIVE RAMP METERING FOR URBAN MOTORWAYS BASED ON MACHINE LEARNING DOCTORAL DISSERTATION Supervisors: Sadko Mandžuka, PhD, Full Professor Edouard Ivanjko, PhD, Associated Professor Zagreb, 2018 Fakultet prometnih znanosti Martin Gregurić KOOPERATIVNO UPRAVLJANJE PRILJEVNIM TOKOVIMA NA URBANIM AUTOCESTAMA ZASNOVNO NA STROJNOM UČENJU DOKTORSKI RAD Mentori: prof. dr. sc. Sadko Mandžuka izv. prof. dr. sc. Edouard Ivanjko Zagreb, 2018 Biographies of Supervisors Sadko Mandžuka PhD is a professor at the Division of Intelligent Transportation System, Faculty of Traffic and Transport Sciences, University of Zagreb. He had the opportunity to work both in academic and industrial environments including Brodarski Institute Ltd, consulting in the Innovation Area for SME’s, etc. Prof. Mandžuka is a president of ITS-Croatia, Collaborating member of Croatian Academy of Engineering, Founding member of Croatian Robotic Association, and a member of Technical Committee on Marine Systems (Coordinating Committee on Transportation and Vehicle Systems – IFAC (International Federation of Automatic Control ), IEEE (Institute of Electrical and Electronics Engineers), IEEE Intelligent Transportation Systems Society etc. Prof. Mandžuka is author of more than 100 internationally reviewed publications. He is the editor of the Engineering Applications Section of the international scientific journal An International Journal of Control and Optimization: Theories & Applications (IJOCTA). Also, he is a member of the editorial board of international scientific journal International Journal of Intelligent Transportation Systems Research (Springer) and the national journal Ceste i mostovi (Roads and Bridges). Prof. Mandžuka was a project leader and collaborating member of several EU and national Research & Development projects. Edouard Ivanjko PhD defended his PhD thesis in 2009 on the Faculty of electrical engineering and computing University of Zagreb. Since 2011 he is with the Department of intelligent transportation systems of the Faculty of transport and traffic sciences, University of Zagreb. His research topics of interests include application of artificial intelligence for road traffic control, autonomous vehicles, and estimation and forecasting of road traffic parameters. As a researcher he was or is active on the following projects: FP7 „Intelligent Cooperative Sensing for improved traffic Efficiency” ICSI, IPA IIIc „Computer Vision Innovations for Safe Traffic“ VISTA and „System for route optimization in a dynamic transport environment“ SORDITO financed from EU structural funds and several MZOS projects. He is or was also a local management committee member for the Republic of Croatia for EU COST actions “Towards autonomic road transport support systems” TU1102 and “High-Performance Modelling and Simulation for Big Data Applications (cHiPSet)” IC1406. He is the local research group leader on the Faculty of transport and traffic sciences for the „Scientific centre of excellence for data science and cooperative systems“. Until now he published three chapters in books, 7 scientific papers in international journals and 37 scientific papers on international conferences. i Acknowledgment I would like to thank my supervisors prof. Sadko Mandžuka and prof. Edouard Ivanjko for guidance and support. This thesis would not have been completed without their support and guidance. Many thanks go to my colleagues at the Department of Intelligent transportation systems and prof. Vanja Ličina. I am deeply grateful to my family, for their remarkable and unconditional support. I am extremely lucky to have them in my life. ii Abstract To cope with today’s urban motorway congestions and the inability to increase motorway capacity in urban environments requires the implementation of advanced control methods. These methods are an integral part of Intelligent Transportation Systems (ITS). An ITS essentially integrates information and communication technology to solve the congestion problems. Ramp metering (RM) and Variable Speed Limit Control (VSLC) are some of the most widely used urban motorway traffic control methods. RM provide direct influence over the on-ramp flows by using specialized traffic lights, while the VSLC control speed of mainstream flow by using variable messaging signs. A dedicated algorithm for RM or VSLC uses sensory data form an urban motorway to compute actions that will have a positive impact on both types of traffic flow. This study will focus on the cooperation of an RM and a VSLC systems, and the integration of several different RM algorithms into a single algorithm called INTEGRA. The algorithm is created by using the Adaptive Neuro-fuzzy Inference System (ANFIS) as an instance of machine learning techniques. Furthermore, INTGERA is expanded in order to integrate its original functionality with a recurrent neural network for traffic demand prediction. As the final step, this doctoral thesis will provide evaluation of different criteria for learning dataset functional setup, based on which ANFIS neural network of INTEGRA will be learned. Results of all mentioned approaches will be compared and discussed in relation with other commonly used urban motorway control methods. Key words Cooperative control, ramp metering, variable speed limit control, urban motorways, adaptive neuro fuzzy inference systems, recurrent neural network, learning dataset iii Prošireni sažetak Kako bi se ostvario veći stupanj uslužnosti na urbanim autocestama primjenjuju se nove upravljačke metode. Najkorištenije upravljačke metode na urbanim autocestama su upravljanje priljevnim tokovima (engl. ramp metering - RM) i promjenjivo ograničenje brzina vozila. Algoritam za upravljanje priljevnim tokovima ima zadaću računati stupanj propuštanja vozila s ulazne rampe (priljevni tok) u glavni tok u odnosu na ukupan broj vozila koja žele uči u glavni tok, pri tome koristeći ulaznu rampu kao privremeni „spremnik“ vozila. Čitava RM metoda upravljanja prometa na ubranoj autocesti zasniva se na prometnim podatcima koji se prikupljaju u stvarnom vremenu posredstvom prometnih senzora (induktivnih petlji, kamera, itd.), te proslijeđeni RM algoritmu. Stupanj propuštanja vozila u glavni tok proračunat od strane RM algoritma prosljeđuje se specijalnoj upravljivoj prometnoj signalizaciji [4]. Glavnina istraživanja u ovom doktorskom radu vezana je upravo za upravljanje priljevnim tokovima s posebnim naglaskom na kooperaciju s drugim sustavima upravljanja prometom, te primjeni strojnog učenja. Također, u kooperaciji s upravljanjem priljevnih tokova razmatrat će se druge upravljačke metode kao što su sustav zabrane prometovanja određenim prometnim trakama, te potpuno ili djelomično upravljanje vozilima opremljenim posebnim računalnim jedinicama. Od strane autora predložen je neuro-neizraziti okvir za učenje koji omogućuje integraciju različitih strategija upravljanja priljevnim tokovima. CTMSIM makro-simulacijski alat koji je izrađen u Matlab programskom okruženju korišten je u simulaciji odabranih metoda upravljanja prometom na urbanim autocestama. Simulator je proširen od strana autora kako bi podržao kooperativno upravljanje priljevnim tokovima, kao i sustav za promjenjivo ograničenje brzina vozila. Cilj istraživanja: Razviti strategije za upravljanje urbanom autocestom koje će biti zasnovane na konceptu kooperacije te ih evaluirati u relaciji s postojećim samostalnim upravljačkim strategijama. Dodatni cilj je izrada novog okvira za učenje različitih strategija upravljanja priljevnim tokovima. Hipoteze: Upravljanje priljevnim tokovima zasnovano na strojnom učenju, u slučajevima značajnih promjena prometne potražnje, može ostvariti kraće vrijeme putovanja uz prihvatljivi red reda čekanja na ulaznim rampama te prihvatljivog ukupnog kašnjenja u odnosu na dosadašnje strategije upravljanja priljevnim tokovima. Algoritam koji je zasnovan na kooperaciji između više ulaznih rampi i kooperaciji upravljanja priljevnim tokovima i iv promjenjivog ograničenja brzina vozila, može ostvariti značajno bolje rezultate u odnosu na samostalne (pojedinačne) aplikacije upravljanja priljevnim tokovima i promjenjivog ograničenja brzina. Prometne mreže velikih urbanih središta imaju glavnu zadaću opsluživati prometnu potražnju bližih manjih gradova, većih središnjih gradova, te tranzitni promet. Kako bi se rasteretila urbana mreža grada od tranzitnog prometa izgrađene su posebne autoceste pod nazivom - gradske obilaznice, a smještene su na rubnim dijelovima urbanih područja. Urbane obilaznice su ubrzo postale okružene urbanom infrastrukturom s proširenjem urbanih područja. Spomenuto je uzrokovalo njihovu integraciju s urbanom prometnom mrežom. Urbane obilaznice su postale poznate kao urbane autoceste. Glavne značajke urbanih autocesta su: 1. Upitna mogućnost za fizičkim povećanjem postojećih prometnih kapaciteta; 2. Veći broj ulaznih i izlaznih rampi koje su blizu jedna drugoj; 3. Opslužuju tranzitni promet
Details
-
File Typepdf
-
Upload Time-
-
Content LanguagesEnglish
-
Upload UserAnonymous/Not logged-in
-
File Pages163 Page
-
File Size-