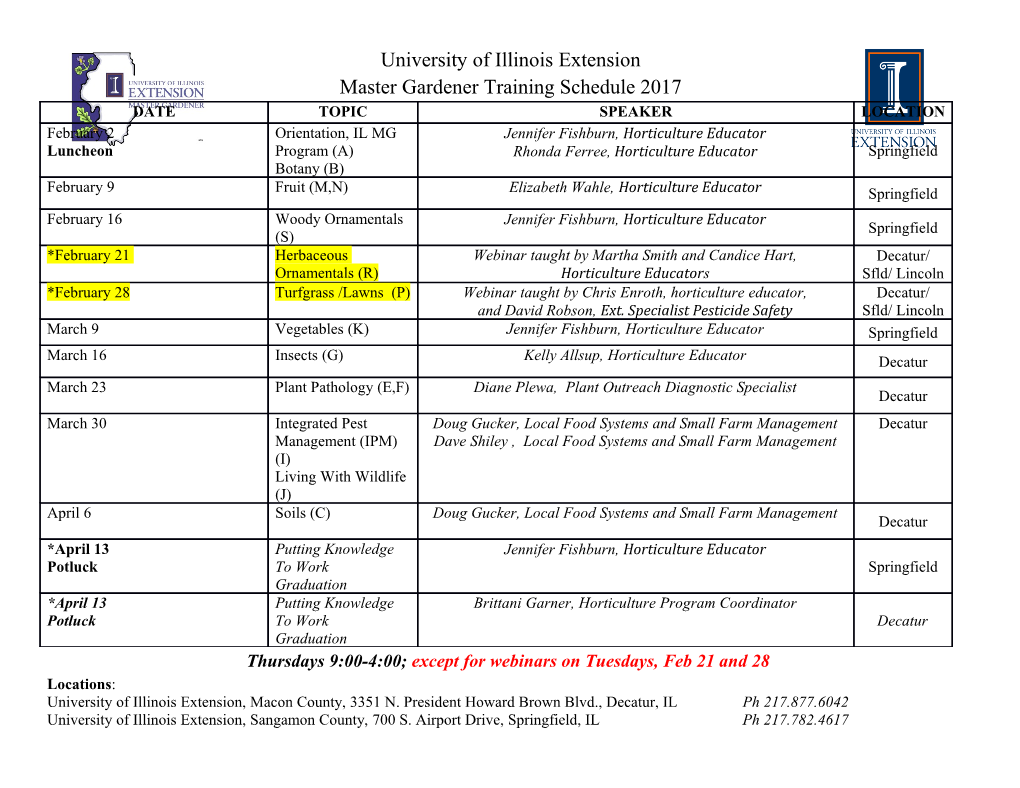
Chapter 1 Introduction to Linear Algebra 1.1 Vector Operations • scalar x: a simple numeric value/ variable, e.g. x = 2:5, x = π, x = 105 • N-dimensional column vector ~v with elements vi: 0 1 v1 B v2 C ~v = B C (1.1) B . C @ . A vN The transpose of ~v, ~v>, is a row vector: > ~v = (v1; v2; : : : ; vN ) (1.2) ~ ~ • Addition of vectors: The sum ~a+b is a vector with elements (~a+b)i = ai+bi • The dot-product (inner product) of two vectors gives a scalar value: ~ >~ ~> X ~a · b = ~a b = b ~a = aibi (1.3) i p pP 2 The norm (length) of vector ~v is given by j~vj = ~v · ~v = i vi ~v Unit/normalized vector v^ of a non-zero vector ~v:v ^ = jvj , jv^j = 1 The dot-product of two vectors has an interesting geometric interpretation: ~a ·~b = j~aj · j~bj · cos(θ) (1.4) Thereby θ is the angle between the two vectors. π ~ Two vectors are orthogonal if θ = 2 , i.e. if ~a · b = 0. The projection of ~a onto ~b (fig. 1.1) is given by: ~a · ^b = j~aj · cos(θ) (1.5) 1 2 CHAPTER 1. INTRODUCTION TO LINEAR ALGEBRA Figure 1.1: Projection of ~a onto ~b. > • Multiplication by a scalar k: k · ~v = (k · v1; k · v2; : : : ; k · vN ) The length of the vector ~v scales with the factor k: s s X 2 2 X 2 jk · ~vj = (k · vi) = k · vi = k · j~vj (1.6) i i Exercise 1.1.1. Calculate all the vector products and the lengths for the vectors: ~v1 = (1; 2; 3);~v2 = (2; 3; 1); ~v3 = (−8; −5; 31). Exercise 1.1.2. Try to explain in your own words why ~v2 · ~v3 = 0 for any 2 vectors ~v1 and ~v2, if ~v3 = ~v1 · j~v2j − ~v2 · ( ~v1 · ~v2). Exercise 1.1.3. Proof that ~a ·~b = j~aj · j~bj · cos(θ), eg. using the law of cosines. 1.1.1 The Linear Neuron Imagine a neuron A receiving input from N sensory neurons. Each synapse has a weight or efficacy wi and the activity of each pre-synaptic neuron is described by the firing rate xi. Synaptic weights with wi > 0 correspond to excitatory synapses, whereas weights with wi < 0 represent inhibitory synapses. In the case of a linear neuron, the firing rate xA of depends linearly on its input, i.e. its firing rate is a weighted sum of its inputs: X xA = w1x1 + w2x2 + ::: + wN xN = wixi (1.7) i If we describe the neuronal inputs and synaptic weights by vectors ~x and ~w, respectively, then we can write eq. 1.7 for the firing rate xA more compactly as dot product: xA = ~w · ~x (1.8) The output of the linear neuron A is zero precisely if the input vector ~x is orthogonal to the weight vector ~w. The set of input vectors that are orthogonal to the weight vector form a so-called hyperplane in the input space. In other words, our linear neuron is a detector which is maximally sensitive to inputs parallel to a particular direction in the input space and minimally sensitive to inputs lying on a (N −1)-dimensional hyperplane orthogonal to this direction. 1.2. LINEAR MAPPINGS OF VECTORS 3 1.2 Linear Mappings of Vectors Consider a function M(~v that maps a N-dimensional vector ~v to a P -dimensional > vector M(~v) = (M1(~v);M2(~v);:::;MP (~v)) . This mapping is linear if and only if: 1. For all scalars k: M(k · ~v) = k · M(~v) 2. For all pairs of vectors ~a and ~b: M(~a +~b) = M(~a) + M(~b) This means that each element of M(~v) is determined by a linear combination of the elements ~v. Hence, for each element Mi(~v) we can find some scalars Mij such that: X Mi(~v) = Mi1v1 + Mi2v2 + ::: + MiN vN = Mijvj (1.9) j We arrange the scalars Mij to a P × N-matrix M and define the product M · ~v of matrix M with column vector ~v by: X (M · ~v)i = Mijvj (1.10) j and the product ~v> · M of matrix M with row vector ~v is given by: > X (~v · M)j = viMij (1.11) i This motivates the definition of matrices and matrix multiplication. Thus, each possible linear function on any vector can be described by multiplying the vector with a corresponding matrix. We say the matrix multiplication of a vector corresponds to a linear transformation of the vector. 1.3 Matrix Operations • A P × N-matrix M has P rows and N columns and elements Mij, where i indicates the row index and j represents the column index: 0 1 M11 M12 ··· M1N BM21 M22 ··· M2N C M = B C (1.12) B . .. C @ . A MP 1 MP 2 ··· MPN > > The transpose of M, M , is the matrix with elements Mij = Mji. I.e. the columns and rows of M are flipped: 0 1 M11 M21 ··· MP 1 B M12 M22 ··· MP 2 C M> = B C (1.13) B . .. C @ . A M1N M2N ··· MPN 4 CHAPTER 1. INTRODUCTION TO LINEAR ALGEBRA • Multiplication by a scalar k: The matrix k · M = M · k has the elements (k · M)ij = k · Mij • Addition of matrices: A + B is a matrix with elements (A + B)ij = Aij + Bij • The matrix-product of M ×N-matrix A with N ×P -matrix B is defined as follows: 0 1 0 1 A11 A12 ··· A1N B11 B12 ··· B1P B A21 A22 ··· A2N C B B21 B22 ··· B2P C A · B = B C · B C B . .. C B . .. C @ . A @ . A AM1 AM2 ··· AMN BN1 BN2 ··· BNP . 0 1 A~ 1 0 1 B A~ C B 2 C ~ ~ ~ = B . C · @ B1 B2 ··· BP A B . C @ . A A~M 0 1 A~1 · B~1 A~1 · B~ 2 ··· A~1 · B~P ~ ~ ~ ~ ~ ~ B A2 · B1 A2 · B2 ··· A2 · BP C = B C B . .. C @ . A A~M · B~1 A~M · B~ 2 ··· A~M · B~P 0 P P P 1 A1iBi1 A1iBi2 ··· A1iBiP Pi Pi Pi B i A2iBi1 i A2iBi2 ··· i A2iBiP C = B C (1.14) B . .. C @ . A P P P i AMiBi1 i AMiBi2 ··· i AMiBiP For each row of A we calculate the dot-product with each column of B. Note, in general the matrix-product is not commutative: AB 6= BA • An N × N-matrix is a square matrix. A square matrix M is called > symmetric if M = M . This means Mij = Mji for all i and j. • The identity matrix 1 is a matrix that is Mii = 1 on the diagonal and Mij = 0; i 6= j otherwise. • The inverse of a square matrix M is a matrix M−1 satisfying: M−1 · M = M · M−1 = 1 (1.15) Note, not all matrices have an inverse, but if the inverse exists, it is unique. If the inverse M−1 exists, the matrix M is called invertible. Exercise 1.3.1. Calculate the following products: A~v, ~v>B, AB and BA for: 01 5 61 04 1 31 > ~v = (1; 1; 1) ; A = @3 2 5A ; B = @2 1 1A 4 1 7 3 1 2 1.4. LINEAR EQUATIONS 5 Exercise 1.3.2. Show that (AB)> = B>A>. Exercise 1.3.3. Show that (A>)−1 = (A−1)>. Exercise 1.3.4. Suppose A and B are both invertible N × N-matrices. Show that (AB)−1 = B−1A−1. 1.4 Linear Equations A central problem of linear algebra is to solve systems of linear equations (SLE) with several unknowns. Simple SLE can be solved by substitution and elimina- tion. For example suppose the following SLE: 2x + 3y = 6 4x + 9y = 15: 1. We solve the top equation for x in terms of y: 3 x = 3 − y 2 2. Then we substitute the expression for x into the bottom equation: 3 4 3 − y + 9y = 15 2 3. Now we solve this equation for y and get y = 1. This in turn we substitute 3 3 into the reduced equation of the first step and we get: x = 3 − 2 · 1 = 2 However, for more complicated SLE with more equations and more unkowns we need a moore systematic approach. A method that is particularly useful and efficient for numerical solutions to SLE is Gaussian elimination. We will discuss the Gaussian elimination algorithm by solving the following SLE: v1 + v2 + v3 = 0 4v1 + 2v2+ v3 = 1 9v1 + 3v2+ v3 = 3 1. Write the SLE in matrix-form M · ~v = ~b and generate the extended coefficient matrix: 0 1 0 1 1 1 0 1 @ M ~b A = @ 4 2 1 1 A 9 3 1 3 2. The goal is to turn M into the identity matrix by • swapping rows • multiplying rows by a scalar value • adding/ subtracting rows from each other 6 CHAPTER 1. INTRODUCTION TO LINEAR ALGEBRA 0 1 0 1 1 1 1 0 R2−4·R1 1 1 1 0 R3−9·R1 @ 4 2 1 1 A −−−−−−! @ 0 −2 −3 1 A 9 3 1 3 0 −6 −8 3 R3−3·R2 0 1 1 1 0 1 R1−R3 0 1 1 0 0 1 − 1 ·R R − 3 ·R 2 2 3 1 2 2 3 1 −−−−−−! @ 0 1 2 − 2 A −−−−−−! @ 0 1 0 − 2 A 0 0 1 0 0 0 1 0 0 1 1 1 0 0 2 R1−R2 1 −−−−−! @ 0 1 0 − 2 A 0 0 1 0 3.
Details
-
File Typepdf
-
Upload Time-
-
Content LanguagesEnglish
-
Upload UserAnonymous/Not logged-in
-
File Pages11 Page
-
File Size-