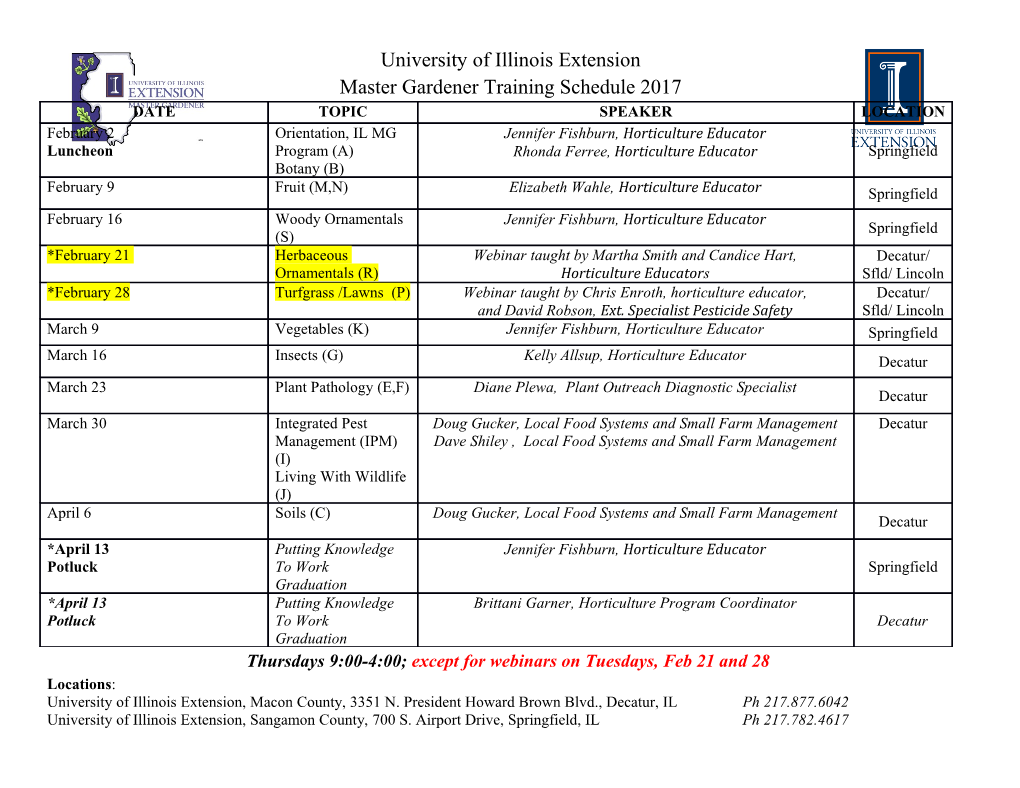
TWITTER OR FACEBOOK? WHICH DATA IS MOST INDICATIVE FOR MOVIE SALES? Word count: 11,677 Lore Aelter Student number: 01201548 Michiel Vanluchene Student number: 01206078 Supervisor: Prof. dr. Dirk Van den Poel Master’s Dissertation submitted to obtain the degree of: Master of Science in Business Engineering Academic year: 2016 - 2017 TWITTER OR FACEBBOK? WHICH DATA IS MOST INDICATIVE FOR MOVIE SALES? Word count: 11,677 Lore Aelter Student number: 01201548 Michiel Vanluchene Student number: 01206078 Supervisor: Prof. dr. Dirk Van den Poel Master’s Dissertation submitted to obtain the degree of: Master of Science in Business Engineering Academic year: 2016 - 2017 Deze pagina is niet beschikbaar omdat ze persoonsgegevens bevat. Universiteitsbibliotheek Gent, 2021. This page is not available because it contains personal information. Ghent Universit , Librar , 2021. ii Preface This thesis is not only the written version of our research but also the culmination of our training as business engineers. It concludes an intense, educational and wonderful five years at Ghent university. As we both mastered in Operations Management, opting for a master’s thesis in the field of Data Analytics was not straightforward. However, we both wanted to broaden our scope by deepening ourselves in an area we were unfamiliar with. At the start of this thesis, we want to express our gratitude to the people without whom this project could not have been successful. First and foremost, we want to thank Matthias Bogaert, who guided us every step of the way, and who was always willing to answer our questions and give thorough feedback. We also appreciate the opportunity Prof. Dr. Dirk Van den Poel gave us to elaborate on a subject within the Department of Marketing. Furthermore, we are grateful to our parents for allowing us to educate ourselves and providing us with a promising future. We also thank them and Olivier for proofreading our report. Next, we thank Sofie for providing us with guidance in the Data Analytics field. Finally, we want to thank the reader for his or her interest and we hope he or she will enjoy reading our work. Lore Aelter Michiel Vanluchene iii iv Table of content Confidentiality agreement ............................................................................................................................... i Preface.............................................................................................................................................................iii Table of content .............................................................................................................................................. v List of used abbreviations .............................................................................................................................. vii List of tables .................................................................................................................................................. viii List of figures ................................................................................................................................................... ix Samenvatting .................................................................................................................................................. 1 Abstract ........................................................................................................................................................... 2 1. Introduction ................................................................................................................................................. 3 2. Literature overview ..................................................................................................................................... 4 3. Methodology ............................................................................................................................................... 9 3.1 Data ....................................................................................................................................................... 9 3.2 Variables .............................................................................................................................................. 10 3.2.1 Model description ........................................................................................................................ 10 3.2.2 Dependent variable description ................................................................................................... 11 3.2.3 Sentiment analysis ....................................................................................................................... 11 3.2.4 Predictors ..................................................................................................................................... 12 3.3 Analytical techniques .......................................................................................................................... 15 3.3.1 Linear regression (LR) ................................................................................................................... 15 3.3.2 K-nearest neighbors (KNN) ........................................................................................................... 16 3.3.3 Decision trees (DT) ....................................................................................................................... 16 3.3.4 Bagged trees (BT) ......................................................................................................................... 17 3.3.5 Random forest (RF) ...................................................................................................................... 17 3.3.6 Gradient boosted models (GBM) ................................................................................................. 18 3.3.7 Neural networks (NN) .................................................................................................................. 18 3.4 Model evaluation criteria .................................................................................................................... 19 3.5 Cross validation ................................................................................................................................... 20 3.5.1 Information fusion-based variable importance ........................................................................... 21 3.5.2 Partial dependence plots ............................................................................................................. 23 4. Results and discussion ............................................................................................................................... 23 v 4.1 Benchmarking algorithms ................................................................................................................... 23 4.2 Model performance comparison ........................................................................................................ 26 4.3 Variable importances and partial dependence plots .......................................................................... 28 4.3.1 Information fusion-based variable importances .......................................................................... 28 4.3.2 Partial dependence plots ............................................................................................................. 31 5. Conclusion and practical implications ....................................................................................................... 33 6. Limitations and further research ............................................................................................................... 35 References ....................................................................................................................................................... xi Attachments ................................................................................................................................................. 1-1 Attachment 1 Overview movies .......................................................................................................... 1-1 Attachment 2 Overview and explanation variables ............................................................................ 2-1 Attachment 3 Variable importances ................................................................................................... 3-1 Attachment 4 Partial dependence plot FbTwAll ................................................................................. 4-1 vi List of used abbreviations API Application programming interface BT Bagged trees CART Classification and regression trees CEB Customer engagement behavior DT Decision trees FbAll Model including all data from Facebook FbPost Model including page info and page-generated data from Facebook FbPostTwTweet: Model including page info and page-generated data from Facebook and Twitter FbTwAll Model including all data from Facebook and Twitter GBM Gradient boosted models KNN K-nearest neighbors Lasso Least absolute shrinkage and selection operator LR Linear regression MAE Mean average error MAPE Mean absolute percentage error NN Neural networks PGC Page-generated content PPI Page popularity indicators RF Random forest RMSE Root mean squared error T Time-based measure TwAll Model including all data from Twitter TwTWeet Model including page info and page-generated data from Twitter U Unbounded measure UGC User-generated content
Details
-
File Typepdf
-
Upload Time-
-
Content LanguagesEnglish
-
Upload UserAnonymous/Not logged-in
-
File Pages77 Page
-
File Size-