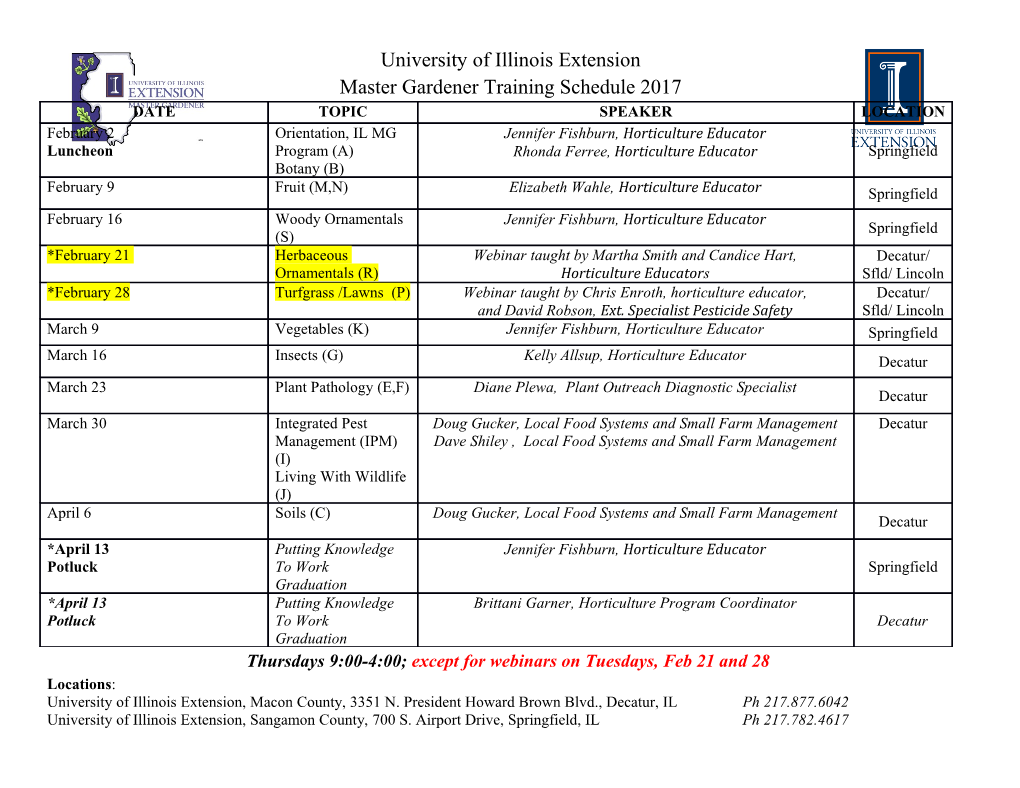
GEOGRAPHIC INFORMATION SYSTEMS FOR SPATIAL DISEASE CLUSTER DETECTION, SPATIO-TEMPORAL DISEASE MAPPING, AND HEALTH SERVICE PLANNING by PING YIN (Under the Direction of Lan Mu and Marguerite Madden) ABSTRACT Geographic information systems (GIS) are increasingly recognized as an effective and efficient tool to deal with geographic questions in health studies. The overarching research question of this dissertation asks how GIS and spatial analysis can be used to facilitate public health studies. Three aspects of health studies are included: spatial disease cluster detection, spatio-temporal disease mapping, and health service planning. New methods or models are proposed and implemented with GIS in this dissertation to address an important problem in each of the three aspects. First, a redesigned spatial scan statistic (RSScan) is proposed to quickly detect disease clusters in arbitrary shapes. The experimental results indicate that the improved RSScan method generally has higher power and accuracy than three existing methods for detecting the clusters in irregular shapes. Second, to explore the spatio-temporal patterns of lung cancer incidence risks in Georgia between 2000 and 2007, a total of seven hierarchical Bayesian models are developed and compared at the census tract level using a two-year time period as the temporal unit. The study shows the northwest region of Georgia has stably elevated lung cancer incidence risks for all the population groups by race and sex. It also shows that there are strong inverse relationships between socioeconomic status and lung cancer incidence risk in males and weak inverse relationships in females in Georgia. Finally, two transportation models that address the modular capacitated maximal covering location problem (MCMCLP) are proposed and used to optimally site ambulances for Emergency Medical Services (EMS) Region 10 in Georgia. As a component of the allocation-location problems for health service planning, spatial demand representation is discussed and three representation approaches are empirically compared in both problem complexity and representation error. Results of this dissertation contribute to the advancement of geospatial analysis in disease surveillance and health service decision making. Future research could include using GIS and spatial analysis to improve the accuracy of detected clusters, explore the environmental factors related to the spatio-temporal patterns of lung cancer incidence risks in Georgia, and integrate population movement in health service planning. INDEX WORDS: GIS, Public health, Cluster detection, Disease mapping, Health planning GEOGRAPHIC INFORMATION SYSTEMS FOR SPATIAL DISEASE CLUSTER DETECTION, SPATIO-TEMPORAL DISEASE MAPPING, AND HEALTH SERVICE PLANNING by PING YIN B.E., Tsinghua University, China, 2002 M.E., Tsinghua University, China, 2005 A Dissertation Submitted to the Graduate Faculty of The University of Georgia in Partial Fulfillment of the Requirements for the Degree DOCTOR OF PHILOSOPHY ATHENS, GEORGIA 2012 © 2012 Ping Yin All Rights Reserved GEOGRAPHIC INFORMATION SYSTEMS FOR SPATIAL DISEASE CLUSTER DETECTION, SPATIO-TEMPORAL DISEASE MAPPING, AND HEALTH SERVICE PLANNING by PING YIN Major Professor: Lan Mu Marguerite Madden Committee: Xiaobai Yao Thomas Jordan John Vena Electronic Version Approved: Maureen Grasso Dean of the Graduate School The University of Georgia August 2012 ACKNOWLEDGEMENTS Five years’ Ph.D. study in the Department of Geography at the University of Georgia (UGA) is great experience to me. I am grateful to all of those people who supported and helped me to finish my dissertation research. First and foremost, my deepest gratitude goes to my major professors, Dr. Lan Mu and Dr. Marguerite Madden, for their excellent guidance and full supports. Without their endless input, timely feedbacks, and great inspiration, I cannot have my research finished today. I really appreciate their dedication and generous help to my research and other academic activities. I would thank Dr. John Vena in the Department of Epidemiology and Biostatistics at UGA for providing me the health data for my research. His invaluable advice from an epidemiological perspective greatly improves my research. I would also acknowledge Dr. Xiaobai Yao and Dr. Thomas Jordan for their insightful advices and suggestions on this research and other academic areas. I want to thank Dr. Andrew Herod. He made me realize that how important correct citations are in academic writing. The institutions that sponsored my research deserve special notice. They are the UGA research foundation and the UGA graduate school with the dean’s award in social sciences and the dissertation completion award. Finally, I deeply thank my parents and my wife, Jing. It is their unconditional love and endless patience that encourage me to finish my dissertation. iv TABLE OF CONTENTS Page ACKNOWLEDGEMENTS .......................................................................................................... iv LIST OF TABLES ...................................................................................................................... viii LIST OF FIGURES ........................................................................................................................ x CHAPTER 1 INTRODUCTION AND LITERATURE REVIEW .................................................... 1 1.1 Background ....................................................................................................... 1 1.2 Research Objectives .......................................................................................... 6 1.3 Literature Review.............................................................................................. 8 1.4 Dissertation Structure...................................................................................... 12 References ............................................................................................................. 13 2 DETECTING DISEASE CLUSTERS IN ARBITRARY SHAPES WITH A REDESIGNED SPATIAL SCAN STATISTIC ......................................................... 18 Abstract ................................................................................................................. 19 2.1 Introduction ..................................................................................................... 20 2.2 Existing Methods for Detection of Disease Clusters ...................................... 21 2.3 Redesigned Spatial Scan Method (RSScan) ................................................... 24 2.4 Performance Evaluation .................................................................................. 28 2.5 Application: Georgia Lung Cancer, 1998 -2005 ............................................. 37 2.6 Discussion and Conclusions ........................................................................... 38 v References ............................................................................................................. 41 3 HIERARCHICAL BAYESIAN MODELING OF THE SPATIO-TEMPORAL PATTERNS OF LUNG CANCER INCIDENCE RISKS IN GEORGIA, 2000-2007 44 Abstract ................................................................................................................. 45 3.1 Introduction ..................................................................................................... 46 3.2 Study Area and Data ....................................................................................... 48 3.3 Methods........................................................................................................... 50 3.4 Results ............................................................................................................. 57 3.5 Discussions ..................................................................................................... 67 3.6 Conclusions ..................................................................................................... 68 References ............................................................................................................. 70 4 MODULAR CAPACITATED MAXIMAL COVERING LOCATION PROBLEM FOR THE OPTIMAL SITING OF EMERGENCY VEHICLES ............................... 73 Abstract ................................................................................................................. 74 4.1 Introduction ..................................................................................................... 75 4.2 Modular Capacitated Maximal Covering Location Problem (MCMCLP) ..... 78 4.3 Spatial Demand Representation ...................................................................... 84 4.4 Applications: Optimal Siting of Ambulances ................................................. 85 4.5 Discussion ....................................................................................................... 96 4.6 Conclusion ...................................................................................................... 98 References ............................................................................................................. 99 5 AN EMPIRICAL COMPARISON OF SPATIAL DEMAND REPRESENTATIONS IN MAXIMAL COVERAGE MODELING ............................................................. 102 vi Abstract ............................................................................................................... 103 5.1 Introduction ................................................................................................... 104 5.2 Representation
Details
-
File Typepdf
-
Upload Time-
-
Content LanguagesEnglish
-
Upload UserAnonymous/Not logged-in
-
File Pages158 Page
-
File Size-