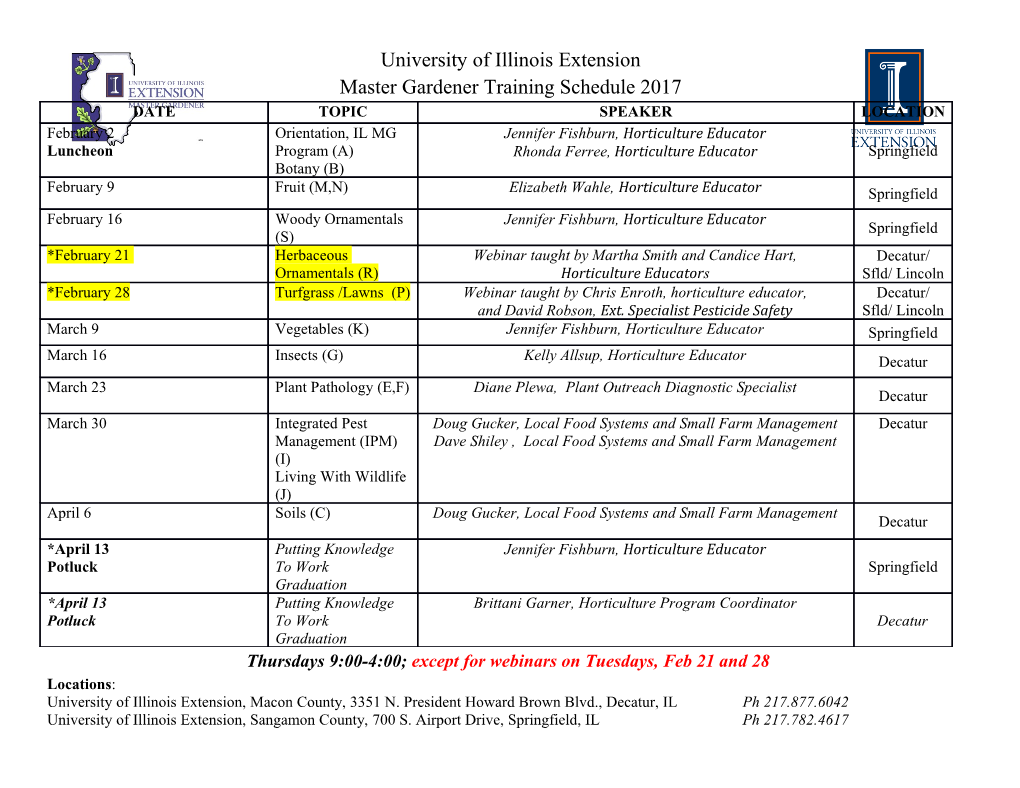
Örebro University School of Business and Economics Statistics, advanced level thesis, 15 hp Supervisor: Panagiotis Mantalos Examiner: Per-Gösta Andersson Spring 2014 Does it exist any excess skewness in GARCH models? – A comparison of theoretical and White Noise values Åsa Grek 890727 Abstract GARCH models are widely used to estimate the volatility. According to previous studies higher moments (skewness and kurtosis) has an impact on how well the GARCH models can capture the volatility sufficiently. There is not any previous study, which investigate if there is excess skewness of the GARCH process. So the aim in this thesis is to investigate the skewness and see if it differs from the theoretical values for the normal distribution. If the values differ it can be an indication of excess skewness. A Monte Carlo simulation conducted the results, where different GARCH models were generated. The mean, variance, skewness and kurtosis were calculated, compared to theoretical values and White Noise values. The result shows that there is an affect on all the mean, variance, skewness and kurtosis. Keywords: GARCH, moments, skewness, kurtosis, White Noise, High Persistence, Medium Persistence, Low Persistence Table of content 1. Introduction 1 1.1 Background and aim 1 1.2 Previous studies 2 1.3 Disposition 3 2. Theory 4 2.1 GARCH (Generalized autoregressive conditional heteroskedasticity) model 4 2.2 GARCH with Generalized Error Distribution (GED) (innovation) 5 2.3 GARCH with Student’s t distribution (innovation) 5 2.4 Centred moments about the mean 5 2.5 White Noise 7 2.4 Kurtosis of the GARCH 7 3. Method 9 3.1 Data generating process 9 3.2 Monte Carlo simulation 14 4. Analysis and Results 15 5. Discussion and conclusion 24 References 25 Appendix 27 1. Introduction 1.1 Background In finance it is important to estimate the volatility (the conditional standard deviation of asset return), but there is usually problem to estimate it correctly due to the skewness and kurtosis. The asset is assumed to follow a Gaussian distribution (i.e. normal distribution) and then the skewness is equal to zero and kurtosis is equal to three. This leads to uncertainty problem because sometimes the asset seems to follow other distributions. In case when the skewness do not have the same values as if the asset has a normal distribution (see section 2.4) (Tsay, 2005). Often the attention has been on the estimation of the mean and the standard deviation since skewness and kurtosis have valuable information about the volatility and thereby the risk of holding the asset (Schittenkopf, Dorffner and Dockner). Patton (2004) found that knowledge of higher moments (skewness and kurtosis) make a significant better forecast on assets, both statistically and economically. As skewness and kurtosis clearly have an effect on volatility then they are vital measurements for Value-at- Risk (VaR) (Jondeau and Rockinger, 2003). But even if the higher moments are important for forecasting the volatility, few studies have been done in this field. 1.2 Previous studies The first study that examined and derived the formula for kurtosis in GARCH models were Bai, Russell and Tiao in 2003 (se section 2.6). According to them other distributions than the Gaussian includes leptokurtosis (excess kurtosis), which exists in volatility clustering. They found that this affects the kurtosis on the whole time series and the effect is symmetrical. 1 According to Galeano and Tsay (2010) asset returns often have high excess kurtosis, but even when GARCH models with heavy tailed innovations are used the volatility is not sufficiently caught. Posedel (2005) examine the properties of the GARCH (1,1) and find out that the model is heavy tailed but asymptotic normal. Posedel also examine the higher moments and found a restricted conditional kurtosis of the standard deviation in the model. Jondeau and Rockinger (2003) used a GARCH model with Student’s t distribution and then calculated the skewness and kurtosis. The skewness and kurtosis were compared with the maximum theoretical values (see part 2.4) and it seemed like the GARCH model allowed for varying moments. They applied the model on real data and discovered that skewness exists more frequently compared to kurtosis. Breuer and Jandacka (2010) investigated the properties of the aggregated distributions of a GARCH procedure. They observed that as the time series became longer the closer the kurtosis became to three (i.e. converged to the normal distribution) or converged into infinity. From these studies it can be observed that there is an information gap in how the skewness for GARCH models behaves. Because the assets are assume to follow a normal distribution, to be able to capture the movements of the assets (volatility) then the GARCH models has also to follow a normal distribution. So the aim of this thesis is to generate different GARCH models and try to see if the skewness differs from the theoretical value and to the values of White Noise. The study will use GARCH models with different persistence (movement) and different innovation (distribution) terms. The results will be conducted by using Monte Carlo simulation. According to the Central Limit Theorem (CLT) the skewness should converge to the normal distribution and the skewness mean and variance should be close to the theoretical values (see part 2.4). Otherwise if the skewness differs from the values for the theoretical values it indicates that there is excess skewness. 2 1.3 Disposition The next chapter is an introduction to the GARCH model and the different innovations that will be used in the Monte Carlo simulation. The third part is the method, where Data Generating Process (DGP) and the steps in the Monte Carlo simulation are explained. The fourth section is the result where some of the simulation results are shown. The fifth and the final part is discussion and conclusion, where the results are discussed and the conclusions are drawn. 3 2. Theory 2.1 GARCH (Generalized Autoregressive Conditional Heteroskedasticity) models Bollerslev (1986) created the GARCH model in 1986 as a transformation to the ARCH (Autoregressive Conditional Heteroskedasticity) model, which was first created by Engle in 1982. The GARCH (m,s) model is written as: , ∑ ∑ (2.1) Where the ARCH parameters are and the GARCH parameter is . The restrictions is >0, ≥ 0, ≥ 0 and ∑ , which makes the conditional variance is positive and increases ( ). If then the model becomes an ARCH (m) model (Tsay, 2005). For the GARCH (m,s) model with Gaussian distribution , but if the GARCH has a Generalized Error distribution (GED) or Student’s t distribution, follows an GED or a Student’s t distribution (Bollerslev, 2009). The GARCH (1,1) can be written as , (2.2) Where (Tsay, 2005). 4 2.2 GARCH models with Generalized Error Distribution (GED)1 (innovation) The GARCH models can have different distribution (innovation) terms, as in equation (2.1) and (2.2) the first part where for GARCH with Gaussian distribution is for GARCH with Generalized error distribution with d degrees of freedom. The degrees of freedom are by default two (Bollerslev, 2009). 2.3 GARCH models with Student’s t distribution (innovation) As in the previous section the GARCH with student’s t distribution (innovation) is when , where where is degrees of freedom. By default the degree of freedom is usually four (Bollerslev, 2009). 2.4 Central moments about the mean The jth central moment (moment about the mean) for continuous distribution is defined by: [ ] ∫ (2.3) where the first centred moment is the mean and the second is the variance . Skewness is and the kurtosis is . The j:th sample moment is 1 The Generalized error distribution is also known as the generalized normal distribution and generalized Gaussian distribution. The distribution is centred round zero and it is a member of the exponential family of distribution. See Giller (2005) for more information. 5 ∑ ̅ (2.4) To estimate the sample skewness and kurtosis one can use: ̂ ∑ ̅ ̂ (2.5) ̂ ∑ ̅ ̂ (2.6) which are consistent estimates for skewness and kurtosis (Danielsson, 2011) and asymptotically normal distributed (Pearson, 1931). When the sample has a normal distribution2 (or in this case normal innovation) then the sample distribution have movements (2.7) (2.8) where is the sample size (Pearson, 1931). 2 For the normal distribution the skewness is equal to zero and kurtosis is equal to three (Casella and Berger, 2002). 6 2.5 White Noise To be able to estimate the skewness and kurtosis for a Normal (i.e. Gaussian) distribution a White Noise process is used. A sample from a White Noise process is asymptotically normal with mean zero and variance , as the sample size gets large. According to Anderson (1942), Bartlett (1946) and Quenouille (1949) the White Noise can be used to evaluate properties because it is asymptotic normally distributed. 2.6 Kurtosis of GARCH models As in equation (2.2) the GARCH (1,1) model is: , Where >0, ≥ 0, ≥ 0, and . With . Where is the excess kurtosis of (Tsay, 2005). This implies according to Bai, Russell and Tiao (2003) that the expected value and variance of the GARCH (1,1) is: ⁄ (2.9) Then the excess kurtosis of the GARCH (1,1) model is (2.10) 7 If the GARCH (1,1) model has a Gaussian distribution ( ) then the excess kurtosis is written as (2.11) The excess kurtosis can only exist if and > 0 if then (Tsay, 2005). 8 3. Method 3.1 Data generating process To construct the study, recall that the GARCH (1,1) is written as (equation (2.2)) , where the parameters are generated by using different GARCH process. High Persistence (HP) [0.01, 0.09, 0.9] generates a high volatility GARCH process.
Details
-
File Typepdf
-
Upload Time-
-
Content LanguagesEnglish
-
Upload UserAnonymous/Not logged-in
-
File Pages37 Page
-
File Size-