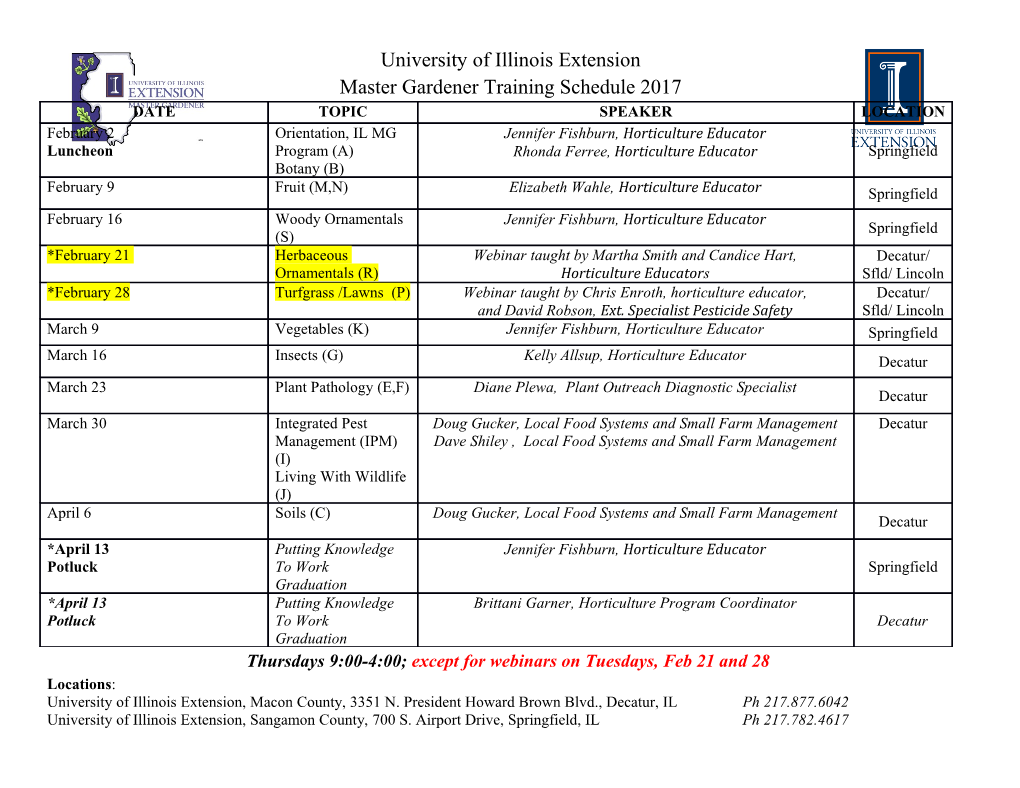
RNA Alternative Splicing Prediction with Discrete Compositional Energy Network Alvin Chan∗ Anna Korsakova∗ Yew-Soon Ong [email protected] [email protected] [email protected] Nanyang Technological University Nanyang Technological University Nanyang Technological University Singapore Singapore Singapore Fernaldo Richtia Winnerdy Kah Wai Lim Anh Tuan Phan [email protected] [email protected] [email protected] Nanyang Technological University Nanyang Technological University Nanyang Technological University Singapore Singapore Singapore ABSTRACT Inference, and Learning (ACM CHIL ’21), April 8–10, 2021, Virtual Event, USA. A single gene can encode for different protein versions through a ACM, New York, NY, USA, 11 pages. https://doi.org/10.1145/3450439.3451857 process called alternative splicing. Since proteins play major roles in cellular functions, aberrant splicing profiles can result in a variety 1 INTRODUCTION of diseases, including cancers. Alternative splicing is determined RNA plays a key role in the human body and other organisms as by the gene’s primary sequence and other regulatory factors such a precursor of proteins. RNA alternative splicing (AS) is a process as RNA-binding protein levels. With these as input, we formulate where a single gene may encode for more than one protein isoforms the prediction of RNA splicing as a regression task and build a new (or mRNA transcripts) by removing selected regions in the initial training dataset (CAPD) to benchmark learned models. We propose pre-mRNA sequence. In the human genome, up to 94% of genes discrete compositional energy network (DCEN) which leverages undergo alternative splicing [36]. AS not only serves as a regulatory the hierarchical relationships between splice sites, junctions and mechanism for controlling levels of protein isoforms suitable for transcripts to approach this task. In the case of alternative splicing different tissue types but is also responsible for many biological prediction, DCEN models mRNA transcript probabilities through states involved in disease [35], cell development and differentiation its constituent splice junctions’ energy values. These transcript [14]. While advances in RNA-sequencing technologies [5] have probabilities are subsequently mapped to relative abundance values made quantification of AS in patients’ tissues more accessible, an of key nucleotides and trained with ground-truth experimental AS prediction model will alleviate the burden from experimental measurements. Through our experiments on CAPD1, we show that RNA profiling and open doors for more scalable in-silico studies of DCEN outperforms baselines and ablation variants.2 alternatively spliced genes. Previous studies on AS prediction mostly either approach it as CCS CONCEPTS a classification task or study a subset of AS scenarios. Training • Applied computing ! Bioinformatics; Health informatics; models that can predict strengths of AS in a continuous range and • Computing methodologies ! Neural networks. are applicable for all AS cases across the genome would allow wider applications in studying AS and factors affecting this important KEYWORDS biological mechanism. To this end, we propose AS prediction as a regression task and curate Context Augmented Psi Dataset (CAPD) energy-based models, machine learning, deep neural networks, to benchmark learned models. CAPD is constructed using high- splicing prediction quality transcript counts from the ARCHS4 database [21]. The data ACM Reference Format: in CAPD encompass genes from all 23 pairs of human chromosomes Alvin Chan, Anna Korsakova, Yew-Soon Ong, Fernaldo Richtia Winnerdy, and include 14 tissue types. In this regression task, given inputs arXiv:2103.04246v1 [q-bio.GN] 7 Mar 2021 Kah Wai Lim, and Anh Tuan Phan. 2021. RNA Alternative Splicing Prediction such as the gene sequence and an array of tissue-wide RNA regula- with Discrete Compositional Energy Network. In ACM Conference on Health, tory factors, a model would predict, for key positions on the gene sequence, these positions’ relative abundance (k) in the mRNAs ∗ Both authors contributed equally to this research. found in a patient’s tissue. 1Dataset available at: https://doi.org/10.21979/N9/FFN0XH 2Codes and models are released at: https://github.com/alvinchangw/DCEN_CHIL2021 Each mRNA transcript may contain one or more splice junctions, locations where splicing occurred in the gene sequence. We hy- Permission to make digital or hard copies of part or all of this work for personal or pothesize that a model design that considers these splice junctions classroom use is granted without fee provided that copies are not made or distributed would be key for good AS prediction. More specifically, these splice for profit or commercial advantage and that copies bear this notice and the full citation on the first page. Copyrights for third-party components of this work must be honored. junctions are produced through a series of molecular processes For all other uses, contact the owner/author(s). during splicing, so modeling the energy involved at each junction ACM CHIL ’21, April 8–10, 2021, Virtual Event, USA may offer an avenue to model the whole AS process. This isthe © 2021 Copyright held by the owner/author(s). ACM ISBN 978-1-4503-8359-2/21/04. intuition behind our proposed discrete compositional energy net- https://doi.org/10.1145/3450439.3451857 work (DCEN) which models the energy of each splice junction, ACM CHIL ’21, April 8–10, 2021, Virtual Event, USA Alvin Chan, Anna Korsakova, Yew-Soon Ong, Fernaldo Richtia Winnerdy, Kah Wai Lim, and Anh Tuan Phan composes candidate mRNA transcripts’ energy values through in all mRNAs found from experimental RNA-sequencing measure- their constituent splice junctions and predicts the transcript proba- ments whilek = 0 means the exon is missing in that particular gene. bilities. The final component maps transcript probabilities to each k can also be annotated onto exon’s key positions such as its start known exon start and end’s k values. DCEN is trained end-to-end and end locations. This allows one to approach the AS prediction in our experiments with ground-truth k labels. Through our ex- as a regression task of predicting k for each nucleotide of interest. periments on CAPD, DCEN outperforms other baselines that lack the hierarchical design to model relationships between splice site, junctions and transcript. To the best of our knowledge, DCEN is the first approach to model the AS process through this hierarchical design. While DCEN is evaluated on AS prediction here, it can po- tentially be used for other applications where the compositionality of objects (e.g., splice junctions/transcripts) applies. All in all, the prime contributions of our paper are as follows: • We construct Context Augmented Psi Dataset (CAPD) to serve as a benchmark for machine learning models in alter- . native splicing (AS) prediction. • To predict AS outcomes, we propose discrete compositional energy network (DCEN) to output k by modeling transcript probabilities and energy levels through their constituent splice junctions. • Through experiments on CAPD, we show that DCEN out- performs baselines and ablation variants in AS outcome pre- diction, generalizing to genes from withheld chromosomes and of much larger lengths. 2 BACKGROUND: RNA ALTERNATIVE Figure 1: Mechanism of alternative splicing and its relation- SPLICING ship with k annotations. Introns in the gene sequences are colored gray while exons (4 , 4 , 4 ) are colored otherwise. RNA alternative splicing (AS) is a process where a single gene (DNA 1 2 3 Each patient sample has an unique set of regulatory factors, / pre-mRNA) can produce multiple mRNAs, and consequently pro- leading to a variety of alternative splicing outcomes in the teins, increasing the biodiversity of proteins encoded by the human population. In the training of DCEN, the DNA sequences of genome. Pre-mRNAs contain two kinds of nucleotide segments, patients are assumed to be the same. introns and exons. Each post-splicing mRNA transcript would only have a subset of exons while the introns and remaining exons are removed. A molecular machine called spliceosome joins the up- stream exon’s end with the downstream exon’s start nucleotides to 3 RELATED WORK form a splice junction and removes the intronic segment between We review prior art on RNA splicing prediction and energy-based these two sites. In an example of an exon-skipping AS event in Fig- models, highlighting those most similar to our work. ure 1, a single pre-mRNA molecule can be spliced into more than one possible mRNA transcripts ()U , )V ) with different probabilities 3.1 Splice Site Classification 2 » ¼ (%)U ,%)V 0, 1 ). These probabilities are largely determined by The earliest task of machine learning on RNA splicing involves local features surrounding the splice sites (exon starts/ends) such as classification of splicing sites such as exon start and end positions the presence of key motifs on the exonic and intronic regions sur- in a given gene sequence, first using models such as decision trees rounding the splice sites nucleotide. Global contextual regulatory [28] and support vector machines [9]. As deep learning gains wider factors such as RNA-binding proteins and small molecular signals adoption, a line of works uses neural networks for splice site pre- [3, 34, 37] can also influence the transcript probabilities, creating diction from raw sequence [19, 24, 43, 45]. In a recent example, variability for AS outcomes in cells from different tissue types or [19] used a 1-D Resnet model to classify individual nucleotides in patients. While exon-skipping is the most common form of AS, a pre-mRNA sequence into 3 categories: 1) exon’s start, 2) exon’s there are others such as alternative exon start/end positions and end or 3) none of the two classes. Unlike these models that only intron retention. classify splice sites, we propose DCEN to predict k levels of splice sites which involve the consideration of patient-specific input such 2.1 Measurement of Alternative Splicing as levels of RNA regulatory factors on top of just primary gene Outcome sequences.
Details
-
File Typepdf
-
Upload Time-
-
Content LanguagesEnglish
-
Upload UserAnonymous/Not logged-in
-
File Pages11 Page
-
File Size-