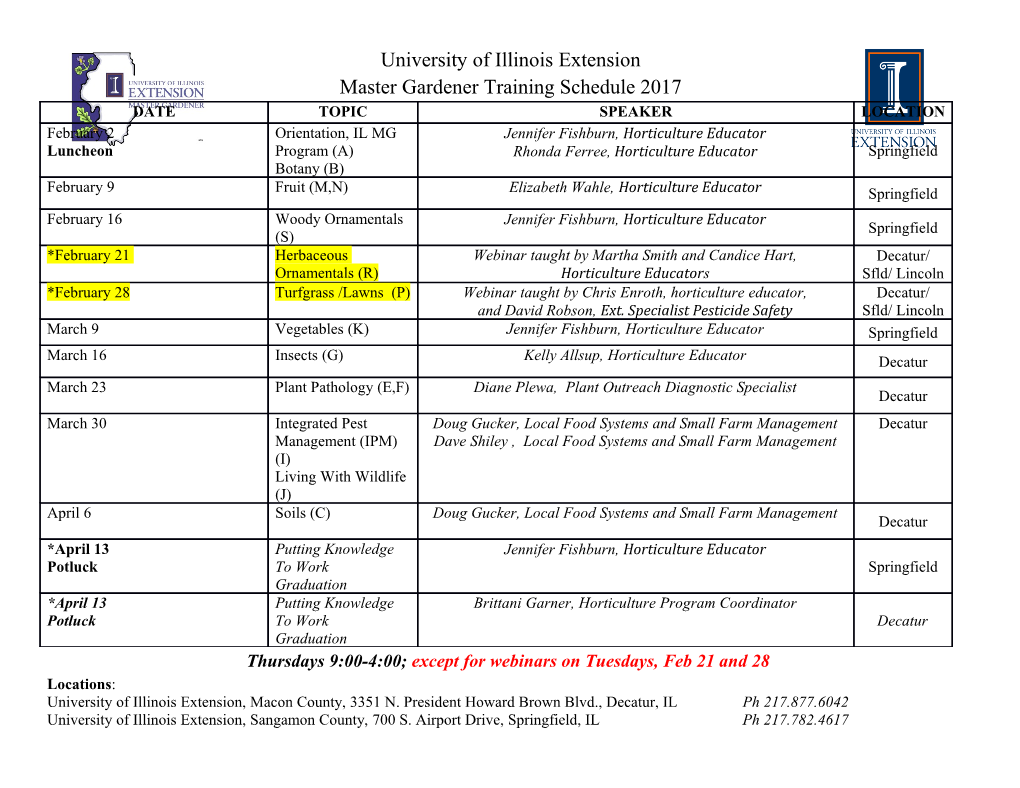
Techniques for indexing large and complex datasets with missing attribute values Safia Brinis SERVIÇO DE PÓS-GRADUAÇÃO DO ICMC-USP Data de Depósito: Assinatura: ______________________ Safia Brinis Techniques for indexing large and complex datasets with missing attribute values Doctoral dissertation submitted to the Instituto de Ciências Matemáticas e de Computação – ICMC- USP, in partial fulfillment of the requirements for the degree of the Doctorate Program in Computer Science and Computational Mathematics. FINAL VERSION Concentration Area: Computer Science and Computational Mathematics Advisor: Prof. Dr. Caetano Traina Junior Co-advisor: Profa. Dra. Agma Juci Machado Traina USP – São Carlos July 2016 Ficha catalográfica elaborada pela Biblioteca Prof. Achille Bassi e Seção Técnica de Informática, ICMC/USP, com os dados fornecidos pelo(a) autor(a) Brinis, Safia B634t Techniques for indexing large and complex datasets with missing attribute values / Safia Brinis; orientador Caetano Traina Junior; coorientadora Agma Juci Machado Traina. – São Carlos – SP, 2016. 136 p. Tese (Doutorado - Programa de Pós-Graduação em Ciências de Computação e Matemática Computacional) – Instituto de Ciências Matemáticas e de Computação, Universidade de São Paulo, 2016. 1. Missing attribute values. 2. Similarity search. 3. Metric access methods. 4. Fractal dimension. I. Junior, Caetano Traina, orient. II. Traina, Agma Juci Machado, coorient. III. Título. Safia Brinis Técnicas de indexação de grandes conjuntos de dados complexos com valores de atributos faltantes Tese apresentada ao Instituto de Ciências Matemáticas e de Computação – ICMC-USP, como parte dos requisitos para obtenção do título de Doutora em Ciências – Ciências de Computação e Matemática Computacional. VERSÃO REVISADA Área de Concentração: Ciências de Computação e Matemática Computacional Orientador: Prof. Dr. Caetano Traina Junior Coorientadora: Profa. Dra. Agma Juci Machado Traina USP – São Carlos Julho de 2016 ACKNOWLEDGEMENTS I would like to thank my advisor Prof. Caetano Traina Jr and my co-advisor Prof. Agma Juci Machado Traina for their wonderful inspiration and commitment to this project, without which this work would not be accomplished. I also like to thank my family and my friends for their inestimable support and encouragement during my work on this project. Many special thanks go to the funding agency CAPES1 for the financial support during this doctoral project. I would also like to thank the funding agencies FAPESP2 and CNPq3 that are supporting the undergoing research in the GBdI4 laboratory. 1 http://www.capes.gov.br 2 http://www.fapesp.br 3 http://cnpq.br 4 http://www.gbdi.icmc.usp.br RESUMO BRINIS, S.. Techniques for indexing large and complex datasets with missing attribute values. 2016. 136 f. Doctoral dissertation (Doctorate Candidate Program in Computer Sci- ence and Computational Mathematics) – Instituto de Ciências Matemáticas e de Computação (ICMC/USP), São Carlos – SP. O crescimento em quantidade e complexidade dos dados processados e armazenados torna a busca por similaridade uma tarefa fundamental para tratar esses dados. No entanto, atributos faltantes ocorrem freqüentemente, inviabilizando os métodos de acesso métricos (MAMs) projetados para apoiar a busca por similaridade. Assim, técnicas de tratamento de dados faltantes precisam ser desenvolvidas. A abordagem mais comum para executar as técnicas de indexação existentes sobre conjuntos de dados com valores faltantes é usar um ”indicador” de valores faltantes e usar as técnicas de indexação tradicionais. Embora, esta técnica seja útil para os métodos de indexação multidimensionais, é impraticável para os métodos de acesso métricos. Esta dissertação apresenta os resultados da pesquisa realizada para identificar e lidar com os problemas de indexação e recuperação de dados em espaços métricos com valores faltantes. Uma análise experimental dos MAMs aplicados a conjuntos de dados incompletos identificou dois problemas principais: distorção na estrutura interna do índice quando a falta é aleatória e busca tendenciosa na estrutura do índice quando o processo de falta não é aleatório. Uma variante do MAM Slim-tree, chamada Hollow-tree foi proposta com base nestes resultados. A Hollow-tree usa novas técnicas de indexação e de recuperação de dados com valores faltantes quando o processo de falta é aleatório. A técnica de indexação inclui um conjunto de políticas de indexação que visam a evitar distorções na estrutura interna dos índices. A técnica de recuperação de dados melhora o desempenho das consultas por similaridade sobre bases de dados incompletas. Essas técnicas utilizam o conceito de dimensão fractal do conjunto de dados e a densidade local da região de busca para estimar um raio de busca ideal para obter uma resposta mais correta, considerando os dados com valores faltantes como uma resposta potencial. As técnicas propostas foram avaliadas sobre diversos conjuntos de dados reais e sintéticos. Os resultados mostram que a Hollow-tree atinge quase 100% de precisão e revocação para consultas por abrangência e mais de 90% para k vizinhos mais próximos, enquanto a Slim-tree rapidamente deteriora com o aumento da quantidade de valores faltantes. Tais resultados indicam que a técnica de indexação proposta ajuda a estabelecer a consistência na estrutura do índice e a técnica de busca pode ser realizada com um desempenho notável. As técnicas propostas são independentes do MAM básico usado e podem ser aplicadas em uma grande variedade deles, permitindo estender a classe dos MAMs em geral para tratar dados faltantes. Palavras-chave: Valores de atributos faltantes, Busca por similaridade, Métodos de acesso métricos, Dimensão fractal. ABSTRACT BRINIS, S.. Techniques for indexing large and complex datasets with missing attribute values. 2016. 136 f. Doctoral dissertation (Doctorate Candidate Program in Computer Sci- ence and Computational Mathematics) – Instituto de Ciências Matemáticas e de Computação (ICMC/USP), São Carlos – SP. Due to the increasing amount and complexity of data processed in real world applications, similarity search became a vital task to store and retrieve such data. However, missing attribute values are very frequent and metric access methods (MAMs), designed to support similarity search, do not operate on datasets when attribute values are missing. Currently, the approach to use the existing indexing techniques on datasets with missing attribute values just use an ”indicator” to identify the missing values and employ a traditional indexing technique. Although, this approach can be applied over multidimensional indexing techniques, it is impractical for metric access methods. This dissertation presents the results of a research conducted to identify and deal with the issues related to indexing and querying datasets with missing values in metric spaces. An empirical analysis of the metric access methods when applied on incomplete datasets leads us to identify two main issues: distortion of the internal structure of the index when data are missing at random and skew of the index structure when data are not missing at random. Based on those findings, a new variant of the Slim-tree access method, called Hollow-tree, is presented. It employs new techniques that are capable to handle missing data issues when missingness is ignorable. The first technique includes a set of indexing policies that allow to index objects with missing attribute values and prevent distortions to occur in the internal structure of the indexes. The second technique targets the similarity queries to improve the query performance over incomplete datasets. This technique employs the fractal dimension of the dataset and the local density around the query object to estimate an ideal radius able to achieve an accurate query answer, considering data with missing values as a potential response. Results from experiments with a variety of real and synthetic datasets show that Hollow-tree achieves nearly 100% of precision and recall for Range queries and more than 90% for k Nearest Neighbor queries, while Slim-tree access method deteriorates with the increasing amount of missing values. The results confirm that the indexing technique helps to establish consistency in the index structure and the searching technique achieves a remarkable performance. When combined, the new techniques allow to explore properly all the available data even with high amounts of missing attribute values. As they are independent of the underlying access method, they can be adopted by a broad range of metric access methods, allowing to extend the class of MAMs. Key-words: Missing attribute values, Similarity search, Metric access methods, Fractal dimen- sion. LIST OF FIGURES Figure 1 – Example of objects with missing values projected in a three-dimensional space using an indicator value. ........................ 30 Figure 2 – Example illustrating objects assinged to a tree node ............. 31 Figure 3 – Example of data distribution in nodes where representatives are complete and where represnetatives have missing values................... 32 Figure 4 – Examples of similarity queries ........................ 41 Figure 5 – An example of a metric space and a range query............... 43 Figure 6 – Slim-tree index structure............................ 46 Figure 7 – Sierpinski triangle............................... 49 Figure 8 – Distance plot of the Sierpinski dataset and its fractal dimension. 50 Figure 9 – An example of a range and k-NNq queries performed over a metric
Details
-
File Typepdf
-
Upload Time-
-
Content LanguagesEnglish
-
Upload UserAnonymous/Not logged-in
-
File Pages138 Page
-
File Size-