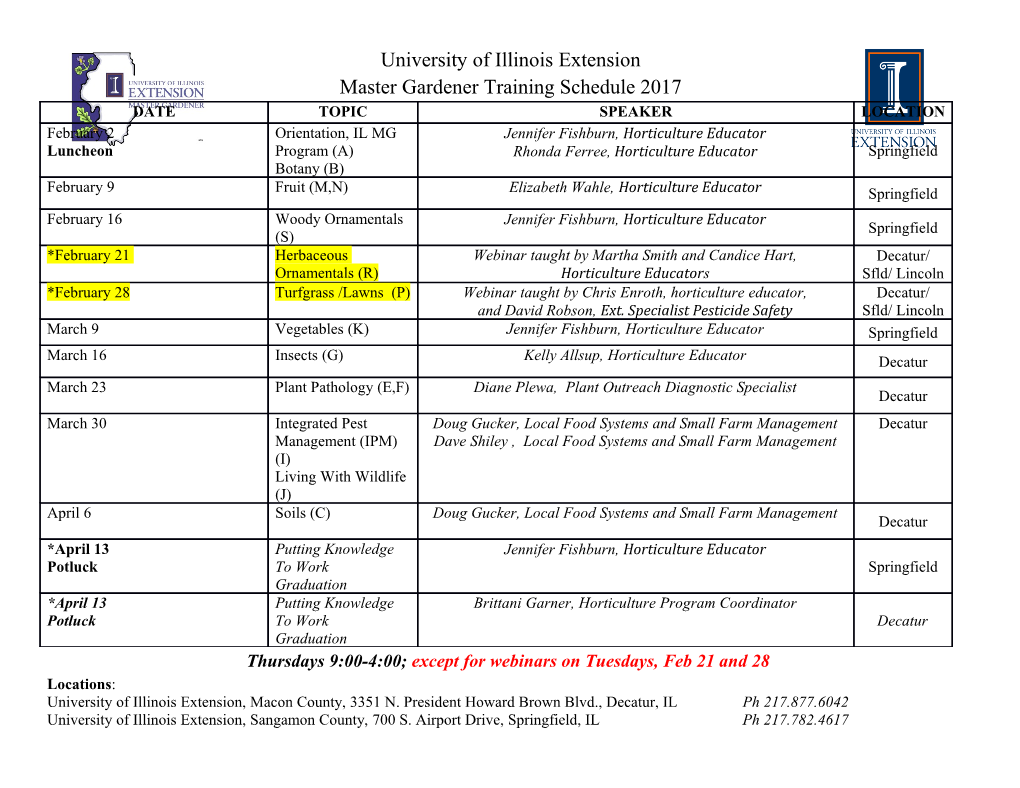
CoMPLEX University College London Mini Project 1 Design Principles of Gene Regulatory Networks in Developmental Biology Dimitrios Voulgarelis Supervisors: Dr. Chris Barnes & Dr. James Briscoe Abstract In the current project two extensively studied examples of pattern formation in developmental biology will be presented. Their mathematical analysis has provided evidence on the importance of GRN dynamics for robust and accurate patterning, essential for correct development. Furthermore, the main emphasis will be put on the research done for the identification of design principles for these transcription factor networks and their relevance to the real biological systems will be discussed. The two examples are the neuronal subtype differentiation in the vertebrate neural tube and the establishment of the body plan in Drosophila melanogaster from the patterning of the anterior-posterior axis. Finally, some original modelling will be presenting comparing the stripe-forming ability of mutual inhibition and cooperativity in a simple 3 − gene network using a new theoretical framework for modelling developmental networks. Contents 1 Gene Regulatory Networks in Real Biological Systems 2 1.1 Vertebrate Neural Tube . .2 1.2 Drosophila Melanogaster Body Plan . .4 2 Design Principles 6 3 Modelling of A Three-Gene Network-Mutual Repression vs Coopera- tivity 12 3.0.1 Methods . 12 3.0.2 Results . 14 3.0.3 Discussion . 16 1 Chapter 1 Gene Regulatory Networks in Real Biological Systems Pattern formation plays a crucial and dominant role in developmental biology. It is es- sential for the differentiation of equivalent cells in the early embryo development. This spatial differentiation is now known to originate from the production and interpretation of morphogens. The first major part of pattern formation during development is the mor- phogen, because morphogens are used by organisms to create polarity along embryonic axes [18]. In developmental biology they can take different forms and they usually are transcription factors acting on downstream genes or target genes directly. The simplest example is a temporally static decaying gradient, but there are cases where the morpogen gradient dynamics change throughout the development [1]. The second part of pattern formation, and the main discussion of this project, are the gene regulatory networks (GRNs), whose role is the interpretation of the morphogens.Gene regulatory networks are comprised of interconnected morphogen downstream genes which can be transcrip- tion factors regulating other transcription factors and target genes, forming a complex circuit. Although other mechanisms were initially believed to drive spatial differentiation in developmental biology (Affinity-Threshold model), GRNs gained ground and a lot of interest has been shown for their dynamics and architecture. It is widely accepted nowa- days that these properties (dynamics & topology) are at the core of pattern formation and cell differentiation for the early embryonic development. The two main examples of pattern formation in developmental biology are the patterning of the vertebrate neural tube and of the embryonic axes in Drosophila melanogaster. 1.1 Vertebrate Neural Tube During the early development, the vertebrate neural tube consists of neural progenitors. From the ventral midline of the neural tube Sonic Hedgehog (Shh) is secreted forming a ventral-dorsal gradient. This gradient is then interpreted to form the expression of patterns of transcription factors (TFs). The expression of these different factors estab- lishes the discrete dorsal-ventral progenitor domains that produce the specific subtypes of motor neurons and interneurons [1]. The basic TFs controlled by the Shh gradient are the Gli TFs. Normally the Gli protein is converted to GliR, a transcriptional repressor, 2 but in the presence of Shh this process is blocked and GliA activator is produced. Due to the fact that there is a Shh gradient present in the neural tube, high near the ventral region and low near the dorsal, two opposite gradients of GliA and GliR are formed [4]. The GRN of downstream Shh target genes is considered to play an essential role in this patterning. To see why the logic of the cross-regulatory network is indeed impor- tant we must first consider a classical model of morphogen pattern formation called the Affinity-Threshold model (AT) [22]. In this model there is positive correlation between the expression range of morphogen target gene and their binding affinity. In the current systems this would mean that genes expressed more ventrally, where the concentration of the morphogen is high, must have lower affinity whereas genes that are expressed more dorsally must have higher binding affinities in order to be able to be expressed in regions where the morphogen concentration is low. Consequently, increasing the binding affinity would increase the range of expression of all genes and lowering the concentration would decrease it. For the neural tube such correlation between expression range and binding affinity was not found [4]. Experimental data using in vivo reporter of Gli activity showed that although more ventrally expressed genes reacted to binding affinity changes according to the Affinity-Threshold model, the more dorsally expressed genes did not. Decreasing the binding affinity increased the expression of these genes. These data showed a clear discordance to the AT model. This could be due to the fact that there are two opposite gradients acting at the same time and could be partially be explained by differences in the cooperativity or binding affinity of Gli isoforms. However, there is no evidence of dif- ferent cooperativity or affinity of Gli isoforms [4] and also the observation of the reporter activity over the course of development showed that the activity of some genes cannot be explained by fixed threshold of Gli activity [1]. These can be an indication that a different mechanism is needed to explain the observed spatial differentiation. In addition, mathematical and computational modeling provided in silico evidence that the architecture of the downstream network was not only able to interpret the morphogen correctly but also to give robustness and accuracy to the patterning. The mathematical model used was a known statistical thermodynamics formulation [24]. In this formulation the probability of a gene being turned ON is given by the ratio of all ON states over all possible states, where ON signifies the gene being expressed. The states are the bound configuration of the DNA to the RNA and to the TFs [3].Then, the concentration of the gene products is found using an ODE that includes the effect of production and degredation. P i=stateswithP bound !i Pbound = P (1.1) 1 + i=allboundstates !i d[C] = α[C] − β[C] (1.2) dt 3 Q Q Where !i = µ Kµ[Xµ] µ,ν cµ,ν. Kµs are the binding affinities, cµ,ν is the coopera- tivity between two occupied sites, µ, ν are the states bound in state i, α is the production rate of proteins, β is the degradation rate and finally [C] is the protein concentration of each transcription factor [3]. Using this model, it was possible to simulate the combinatorial effect of two opposite transcriptional effectors (GliA, GliR) and of the specified GRN. Two independent bind- ing sites were used for the effectors, to provide non-linearity. The GRN was comprised of four mutually repressive genes (Olig2, Nkx2.2, Pax 6, and Irx3). Then using Bayesian methodology the stripe-forming parameter space was found by comparison to the wild- type gene expression patterns [3]. The model was able to both reproduce the experimentally observed behavior occurring when the binding affinity changes and the sharp boundaries of gene expression. Further- more, the system displayed hysteresis, meaning that after a steady state has been reached it can be maintained even if the signal is reduced[3]. Hysteresis is memory and confers robustness in case of decrease of the signaling gradient. In addition to the above, another modeling approach was used for the description of the neural tube GRN providing again evidence that the accurate and robust patterning is an emergent property of the underly- ing network [1]. This other model, despite the fact that it used a different mathematical approach, again shows hysteresis and proves that the network acts as a buffer to signal fluctuations, both essential for in vivo reliable patterning. So, it is clear from both the experimental data and the modelling analysis that GRNs are crucial for the development of the neural tube. 1.2 Drosophila Melanogaster Body Plan One of the most widely studied examples of developmental patterning is the anterior- posterior (head-tail) axis patterning in Drosophila melanogaster. This patterning deter- mines the different body parts of the adult Drosophila. There are three main gene types involved in this procedure, maternal effect genes, segmentation genes and homeotic genes. The maternal effect genes form the protein gradients that are essential for the patterning. These genes include Bicoid (Bcd), Caudal and Nanos. The first two are mainly related to anterior development whereas the last is related to posterior. Bicoid and Nanos act as morphogens of opposite polarity, anterior posterior and posterior-anterior respectively [19]. The second type of genes, segmentation genes, are themselves divided into three categories, gap, pair-rule and segment-polarity genes, all responsible for the final segmen- tation of the embryo. Gap genes are first transcriptionally regulated by maternal effect genes to establish the primary body plan dividing the embryo in large parts. Gap-genes act as transcription factors for pair-rule genes which divide the embryo in pairs of seg- ments. Finally pair-rule genes regulate segment-polarity genes which form the final body segmentations. These different segments assume their identities through the final type of genes, homeotic genes, whose role is the transcriptional regulation of genes responsible for anatomical structures [5]. 4 Manu et al. explored the ability of Drosophila embryos to reduce the phenotypic variations [27].
Details
-
File Typepdf
-
Upload Time-
-
Content LanguagesEnglish
-
Upload UserAnonymous/Not logged-in
-
File Pages20 Page
-
File Size-